SLISEMAP : supervised dimensionality reduction through local explanations
arxiv(2022)
摘要
Existing methods for explaining black box learning models often focus on building local explanations of the models’ behaviour for particular data items. It is possible to create global explanations for all data items, but these explanations generally have low fidelity for complex black box models. We propose a new supervised manifold visualisation method, slisemap , that simultaneously finds local explanations for all data items and builds a (typically) two-dimensional global visualisation of the black box model such that data items with similar local explanations are projected nearby. We provide a mathematical derivation of our problem and an open source implementation implemented using the GPU-optimised PyTorch library. We compare slisemap to multiple popular dimensionality reduction methods and find that slisemap is able to utilise labelled data to create embeddings with consistent local white box models. We also compare slisemap to other model-agnostic local explanation methods and show that slisemap provides comparable explanations and that the visualisations can give a broader understanding of black box regression and classification models.
更多查看译文
关键词
Manifold visualisation, Explainable AI, Local approximation
AI 理解论文
溯源树
样例
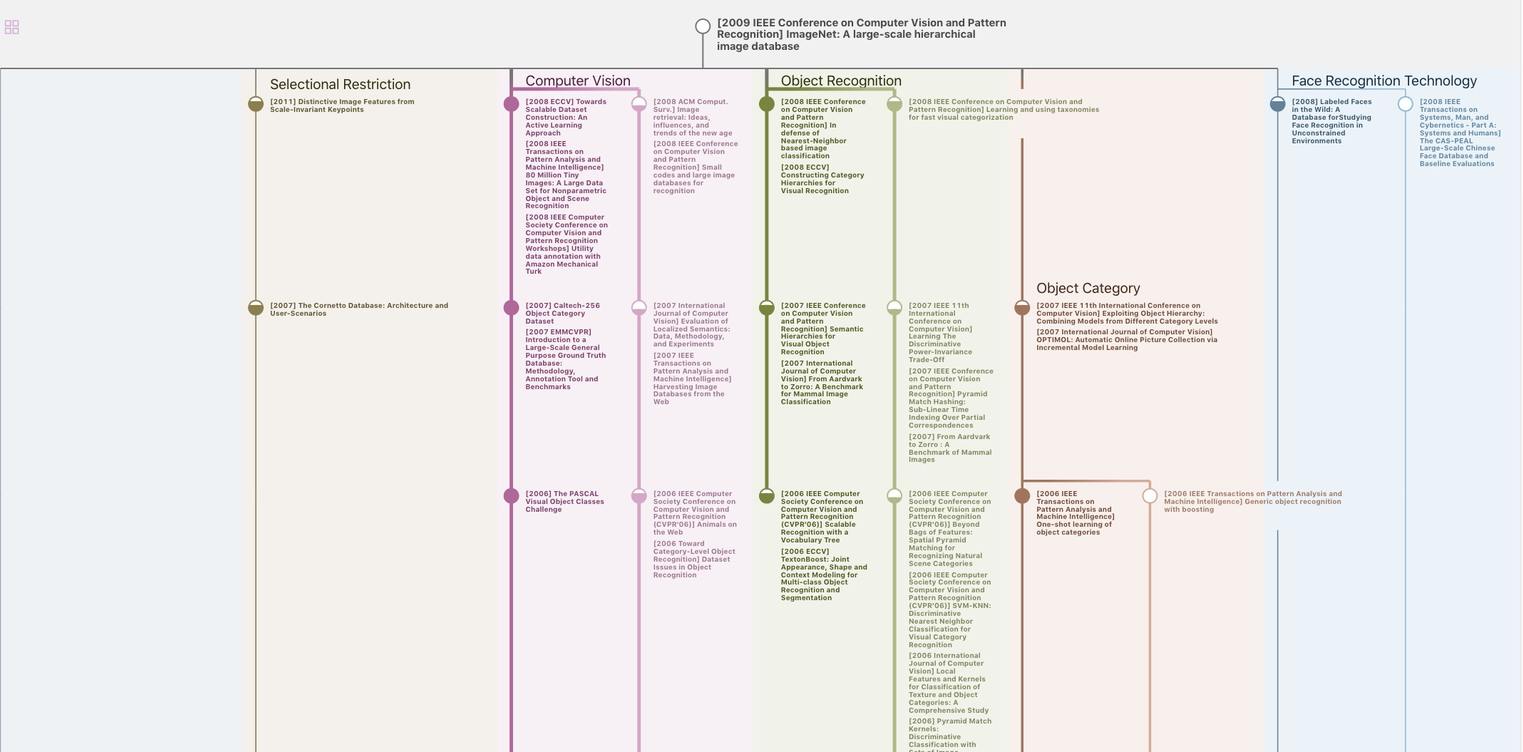
生成溯源树,研究论文发展脉络
Chat Paper
正在生成论文摘要