Low-Rank and Sparse Matrix Recovery for Hyperspectral Image Reconstruction Using Bayesian Learning
SENSORS(2022)
摘要
In order to reduce the amount of hyperspectral imaging (HSI) data transmission required through hyperspectral remote sensing (HRS), we propose a structured low-rank and joint-sparse (L&S) data compression and reconstruction method. The proposed method exploits spatial and spectral correlations in HSI data using sparse Bayesian learning and compressive sensing (CS). By utilizing a simultaneously L&S data model, we employ the information of the principal components and Bayesian learning to reconstruct the hyperspectral images. The simulation results demonstrate that the proposed method is superior to LRMR and SS&LR methods in terms of reconstruction accuracy and computational burden under the same signal-to-noise tatio (SNR) and compression ratio.
更多查看译文
关键词
hyperspectral images, hyperspectral remoting sensing, Bayesian learning, compressive sensing, low-rank and joint-sparse
AI 理解论文
溯源树
样例
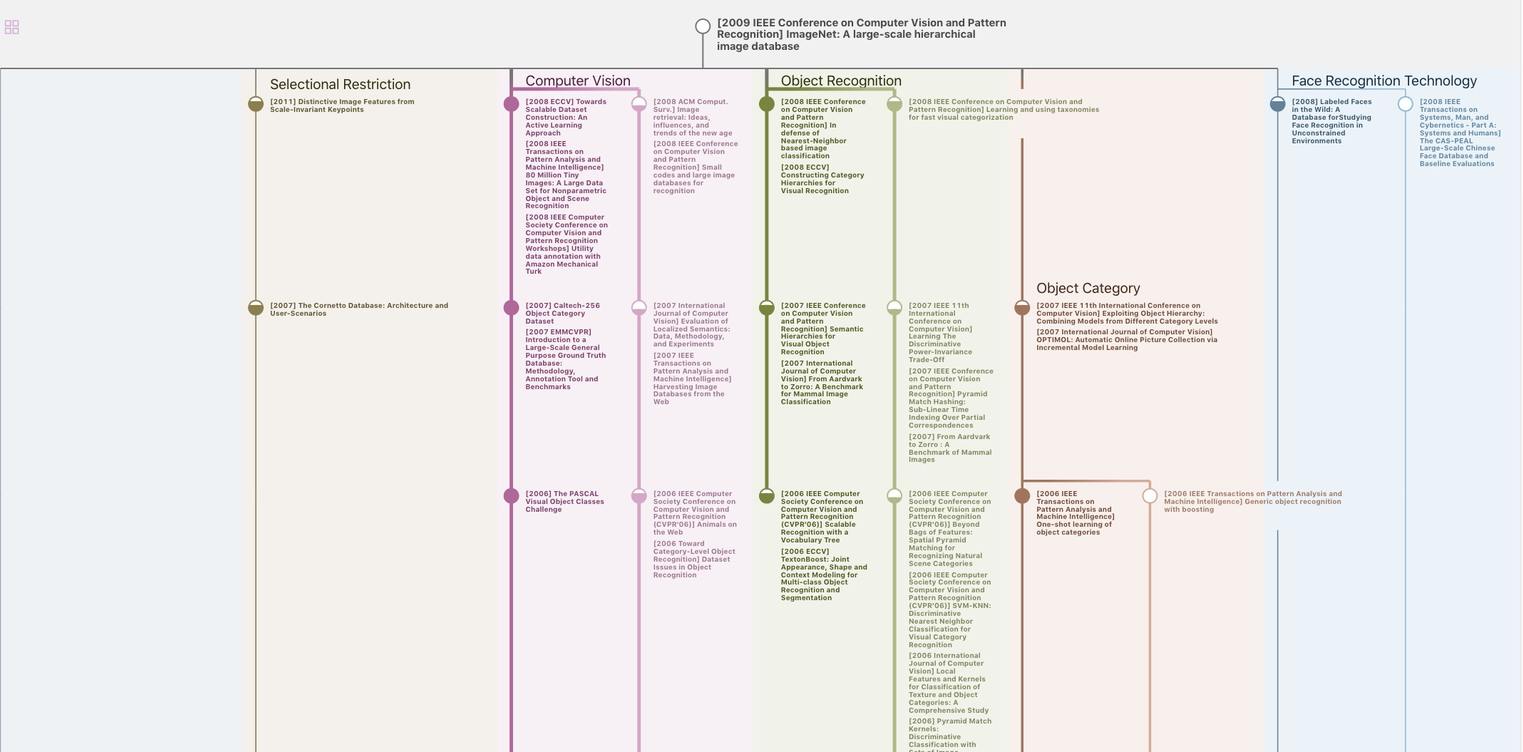
生成溯源树,研究论文发展脉络
Chat Paper
正在生成论文摘要