Interactive Lexical and Semantic Graphs for Semisupervised Relation Extraction
IEEE transactions on neural networks and learning systems(2023)
摘要
The performance of relation extraction (RE) is hindered by the lack of sufficient labeled data. Semisupervised methods can offer to help hands with this problem by augmenting high-quality unlabeled samples into the training data. However, existing semisupervised RE methods either need a set of manually defined rules or rely on the classifier trained on the small labeled data, i.e., the former requires the heavy intervention of human knowledge, and the latter is bound to the number and the quality of the labeled data. In this article, we present a novel semisupervised RE method that involves small human efforts and is robust to the size of the initial set of labeled data. Specifically, we adopt only two simple rules to build the lexical and semantic graphs which connect the labeled samples with the unlabeled ones. In this way, the graphs are much easier to construct yet keep the ability to transfer knowledge from labeled samples to unlabeled ones. We then develop a graph interaction module to fully exploit the reference information in lexical and semantic graphs, which is used to jointly recognize the high-quality unlabeled samples with the classifier. We conduct extensive experimental results on two public datasets. The results demonstrate that our proposed method significantly outperforms the state-of-the-art baselines.
更多查看译文
关键词
Semantics,Hidden Markov models,Task analysis,Training data,Data models,Semisupervised learning,Training,Lexical and semantic graphs,prior knowledge,relation extraction (RE),semisupervised learning
AI 理解论文
溯源树
样例
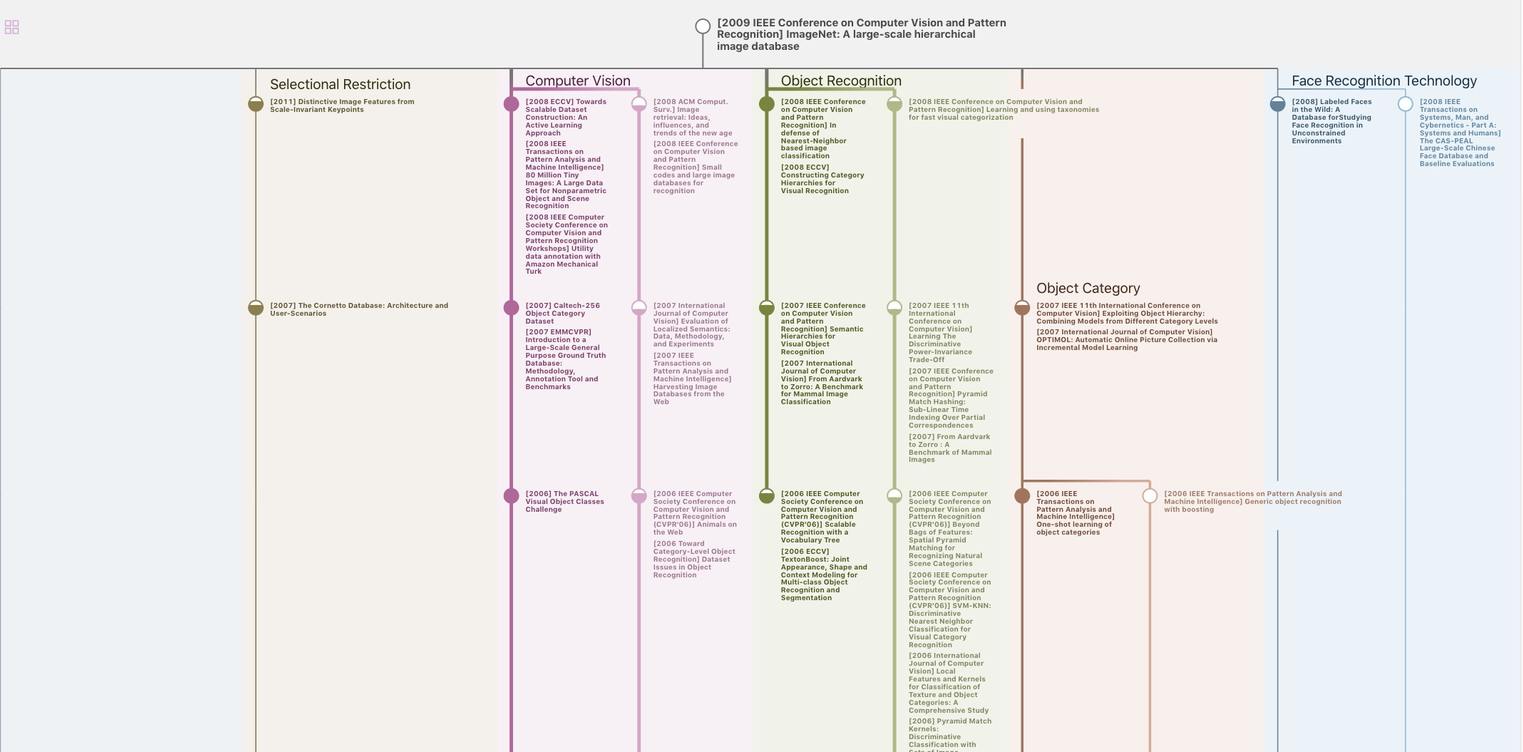
生成溯源树,研究论文发展脉络
Chat Paper
正在生成论文摘要