Neural Network Analysis of Crystalluria Content to Predict Urinary Stone Type
RESEARCH AND REPORTS IN UROLOGY(2021)
摘要
Purpose: To investigate the relationship between urinary stone type and the type of crystals in the urine. Patients and Methods: This retrospective study involved 485 patients with urinary stones treated at King Saud University Medical City from May 2015 to June 2017. Clinical data were obtained from medical records. Different statistical analysis methods were applied, including basic contingency analysis, analysis of variance, logistic regression, discriminant analysis, partition modeling, and neural network evaluations. Results: Of 485 patients, 47 had crystals detected by urinalysis. The most common type of crystal was calcium oxalate (n = 31), which had the highest association with calcium oxalate stones. Uric acid crystals (n = 8) were associated with uric acid stones. The neural network model used for determining the sensitivity and specificity showed an R-square value of 0.88, with an area under the curve of 0.94 for calcium oxalate, 0.94 for carbonate apatite, and 1.0 for uric acid. Conclusion: The predictive algorithm developed in the present study may be used with a patient's clinical parameters to predict the stone type. This approach predicts the stone types associated with certain patient characteristics with a high sensitivity and specificity, indicating that the models may be a valuable clinical tool in the diagnosis, management, and monitoring of stone diseases.
更多查看译文
关键词
crystalluria, urinary sediment, neural network, urolithiasis, stone disease, urinary crystals
AI 理解论文
溯源树
样例
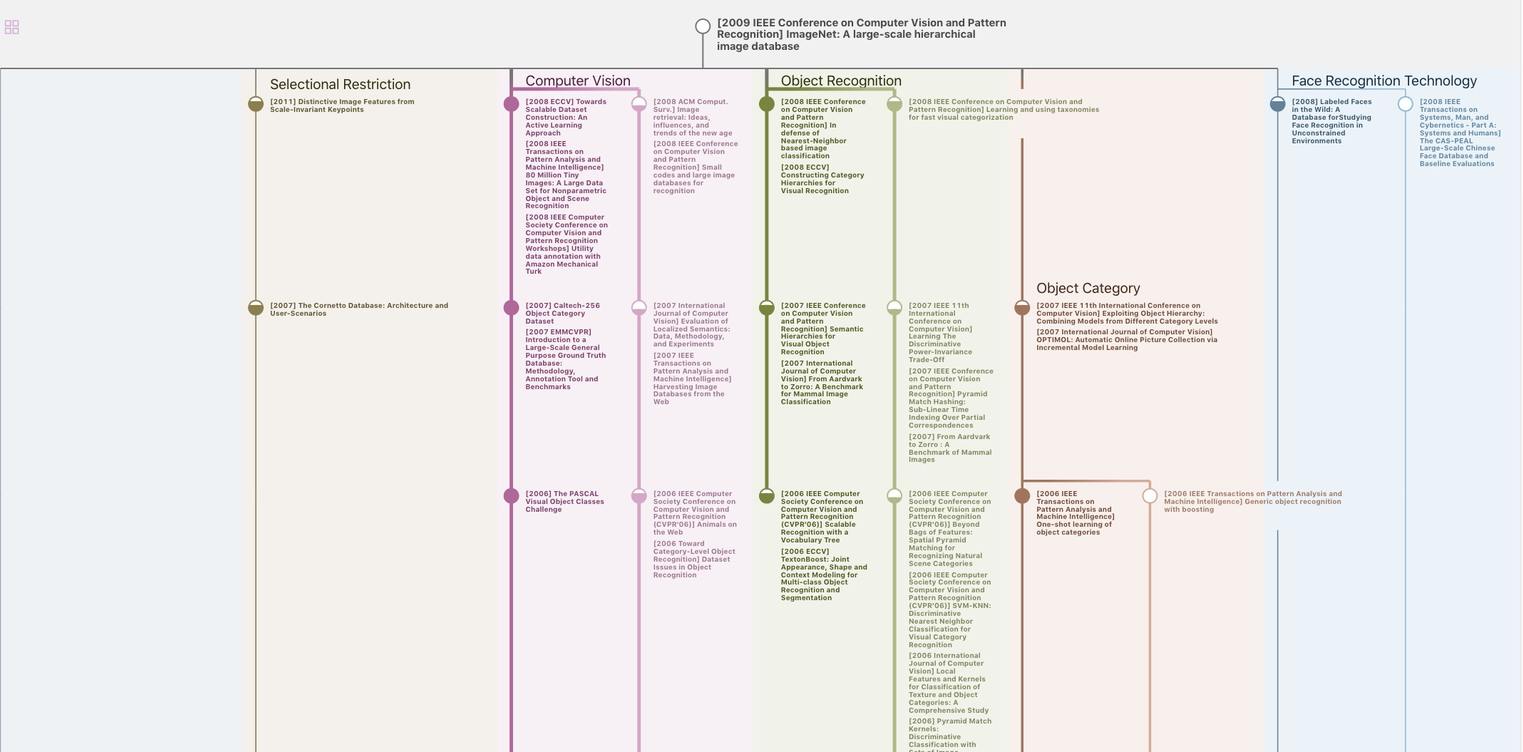
生成溯源树,研究论文发展脉络
Chat Paper
正在生成论文摘要