Unsupervised learning of brain state dynamics during emotion imagination using high-density EEG
NeuroImage(2022)
摘要
This study applies adaptive mixture independent component analysis (AMICA) to learn a set of ICA models, each optimized by fitting a distributional model for each identified component process while maximizing component process independence within some subsets of time points of a multi-channel EEG dataset. Here, we applied 20-model AMICA decomposition to long-duration (1–2 h), high-density (128-channel) EEG data recorded while participants used guided imagination to imagine situations stimulating the experience of 15 specified emotions. These decompositions tended to return models identifying spatiotemporal EEG patterns or states within single emotion imagination periods. Model probability transitions reflected time-courses of EEG dynamics during emotion imagination, which varied across emotions. Transitions between models accounting for imagined “grief” and “happiness” were more abrupt and better aligned with participant reports, while transitions for imagined “contentment” extended into adjoining “relaxation” periods. The spatial distributions of brain-localizable independent component processes (ICs) were more similar within participants (across emotions) than emotions (across participants). Across participants, brain regions with differences in IC spatial distributions (i.e., dipole density) between emotion imagination versus relaxation were identified in or near the left rostrolateral prefrontal, posterior cingulate cortex, right insula, bilateral sensorimotor, premotor, and associative visual cortex. No difference in dipole density was found between positive versus negative emotions. AMICA models of changes in high-density EEG dynamics may allow data-driven insights into brain dynamics during emotional experience, possibly enabling the improved performance of EEG-based emotion decoding and advancing our understanding of emotion.
更多查看译文
关键词
Emotion,Affective computing,Electroencephalography (EEG),Brain states,Non-stationarity,Independent component analysis (ICA),Adaptive mixture ICA (AMICA),Unsupervised learning,Source localization
AI 理解论文
溯源树
样例
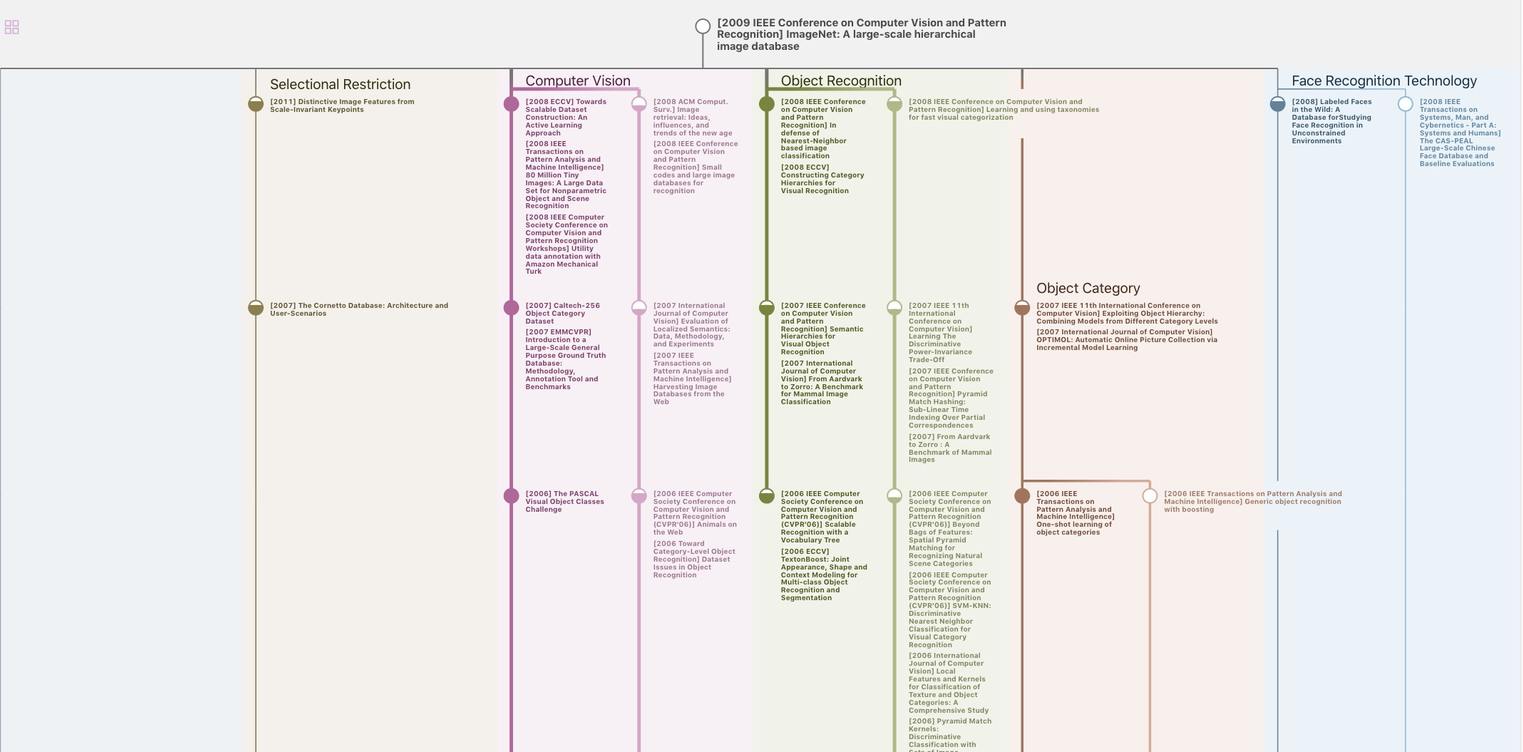
生成溯源树,研究论文发展脉络
Chat Paper
正在生成论文摘要