Cardiac Arrhythmias Classification in Kardiovize Population Study
2021 Computing in Cardiology (CinC)(2021)
摘要
Automatic classification of heart rhythm becomes essential in population studies. There are new options for ECG data handling, such as deep-learning models. In this study, we compare our detector and ELIT 350 ECG Mortara detector using 12-lead volunteers ECG, from Kardiovize study. The ECGs were analyzed by a self-developed deeplearning arrhythmia detector. The evaluation process was focused on atrial fibrillation (AF), the most common arrhythmia in the Czech population. The training database from publicly available datasets included 43,000 variable records. On the training set, the F1-score of the model reached 0.86 and 0.87 for normal sinus rhythm and AF, respectively. In both categories, false positives occur. One of the reasons for the model misclassification was incorrect expert evaluation used as a predicted model output. In the test phase, no records were assigned to the AF category. On the contrary, the Mortara system classified 6 records as AF. Visual verification confirmed the correctness of the model output. From present pilot study, deep-learning classifier ResNet model outperform currently used system in both main categories reaching Sp of 1.0 for atrial fibrillation, and F1 of 0.875 for long QT syndrome. Particularly, no false atrial fibrillation detection were indicated in model output. ECG evaluation using a deep-learning model seems to be useful tool for handling population data.
更多查看译文
关键词
Training,Visualization,Databases,Sociology,Atrial fibrillation,Detectors,Electrocardiography
AI 理解论文
溯源树
样例
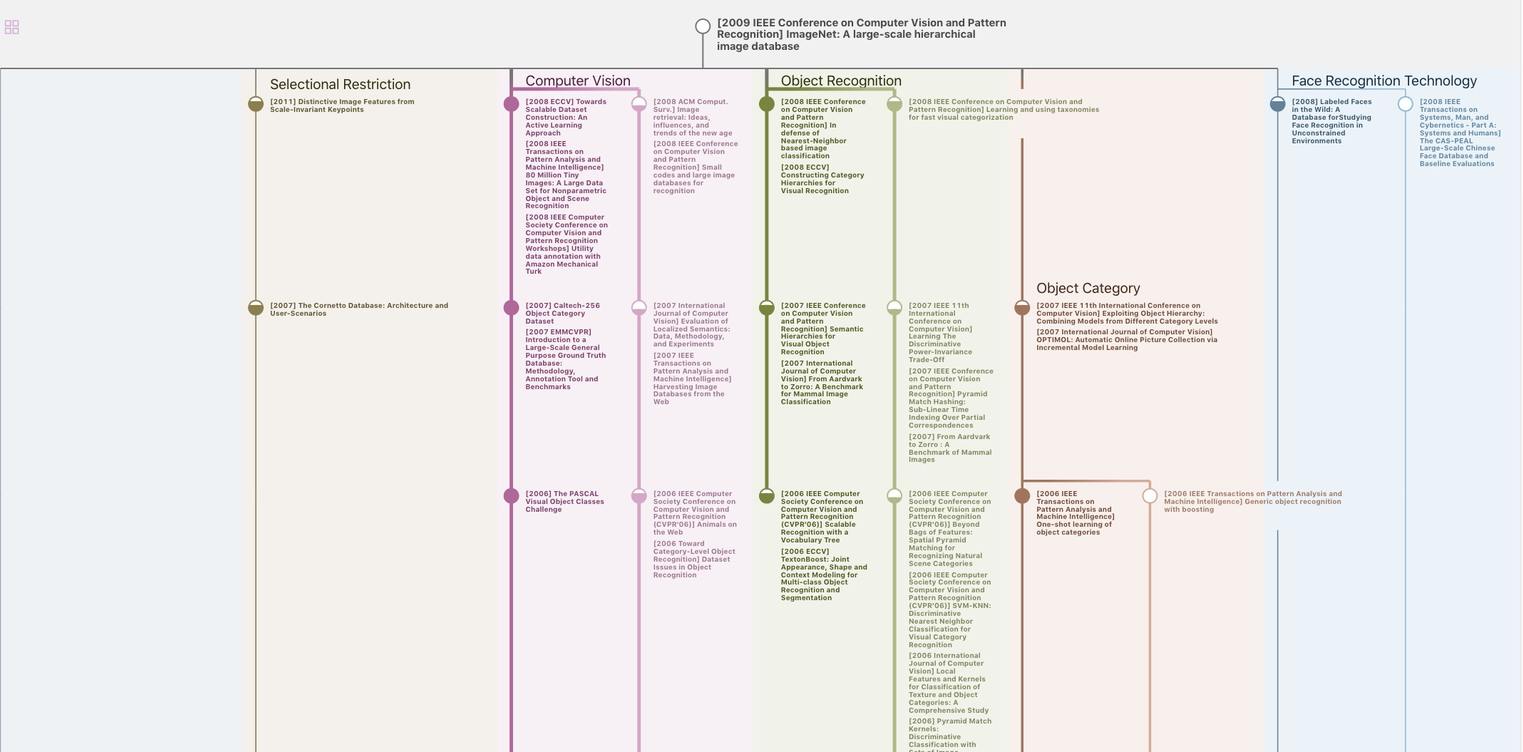
生成溯源树,研究论文发展脉络
Chat Paper
正在生成论文摘要