Noisy Neonatal Chest Sound Separation for High-Quality Heart and Lung Sounds
IEEE journal of biomedical and health informatics(2023)
摘要
Stethoscope-recorded chest sounds provide the opportunity for remote cardio-respiratory health monitoring of neonates. However, reliable monitoring requires high-quality heart and lung sounds. This paper presents novel artificial intelligence-based Non-negative Matrix Factorisation (NMF) and Non-negative Matrix Co-Factorisation (NMCF) methods for neonatal chest sound separation. To assess these methods and compare them with existing single-channel separation methods, an artificial mixture dataset was generated comprising heart, lung, and noise sounds. Signal-to-noise ratios were then calculated for these artificial mixtures. These methods were also tested on real-world noisy neonatal chest sounds and assessed based on vital sign estimation error, and a signal quality score of 1-5, developed in our previous works. Overall, both the proposed NMF and NMCF methods outperform the next best existing method by 2.7 dB to 11.6 dB for the artificial dataset, and 0.40 to 1.12 signal quality improvement for the real-world dataset. The median processing time for the sound separation of a 10 s recording was found to be 28.3 s for NMCF and 342 ms for NMF. With the stable and robust performance of our proposed methods, we believe these methods are useful to denoise neonatal heart and lung sounds in the real-world environment.
更多查看译文
关键词
Heart,Lung,Pediatrics,Recording,Training,Time-frequency analysis,Noise measurement,Artificial intelligence,breath sound,heart sound,lung sound,neonatal,phonocardiogram (PCG),signal quality,single-channel sound separation,telehealth
AI 理解论文
溯源树
样例
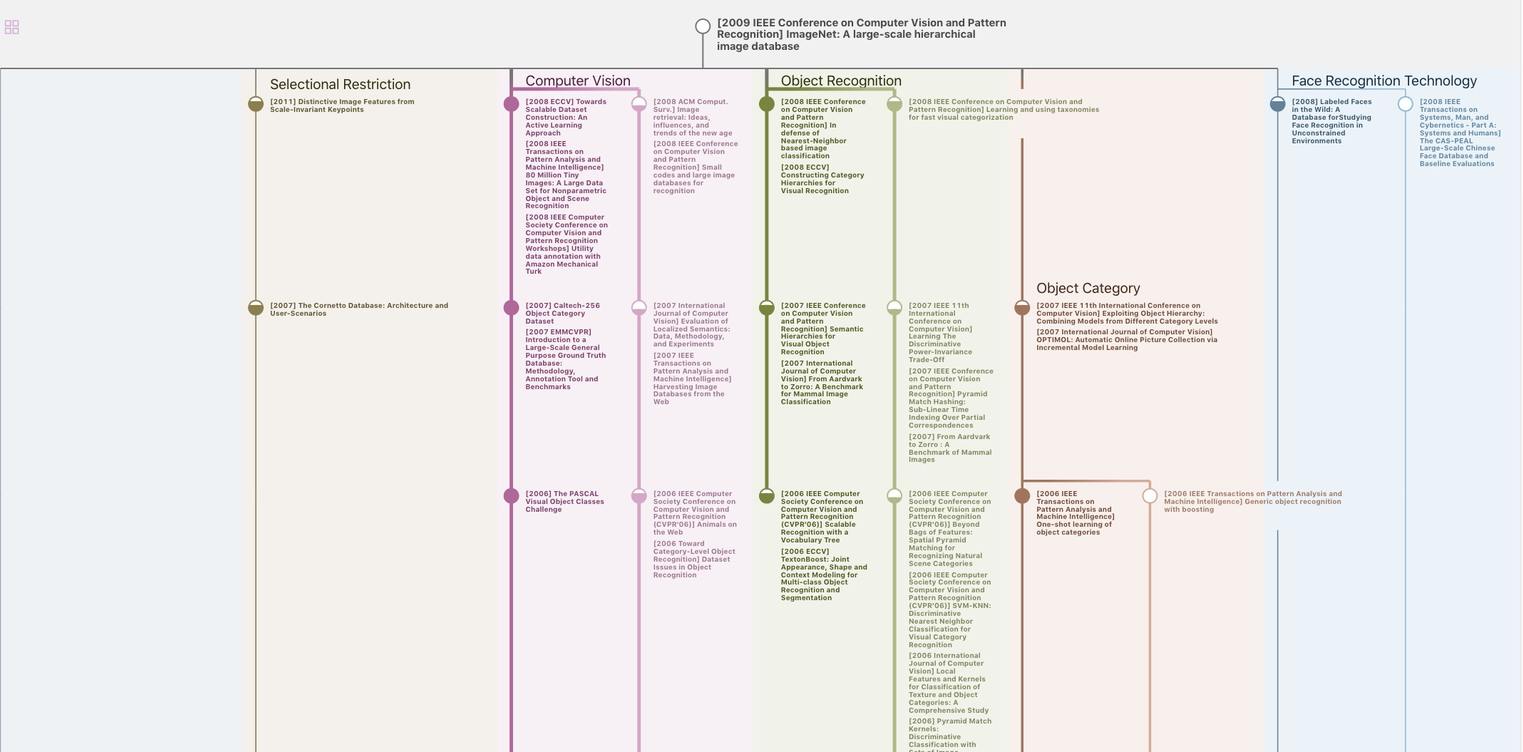
生成溯源树,研究论文发展脉络
Chat Paper
正在生成论文摘要