Weak Supervision for Affordable Modeling of Electrocardiogram Data.
AMIA ... Annual Symposium proceedings. AMIA Symposium(2021)
摘要
Analysing electrocardiograms (ECGs) is an inexpensive and non-invasive, yet powerful way to diagnose heart disease. ECG studies using Machine Learning to automatically detect abnormal heartbeats so far depend on large, manually annotated datasets. While collecting vast amounts of unlabeled data can be straightforward, the point-by-point annotation of abnormal heartbeats is tedious and expensive. We explore the use of multiple weak supervision sources to learn diagnostic models of abnormal heartbeats via human designed heuristics, without using ground truth labels on individual data points. Our work is among the first to define weak supervision sources directly on time series data. Results show that with as few as six intuitive time series heuristics, we are able to infer high quality probabilistic label estimates for over 100,000 heartbeats with little human effort, and use the estimated labels to train competitive classifiers evaluated on held out test data.
更多查看译文
关键词
weak supervision,affordable modeling
AI 理解论文
溯源树
样例
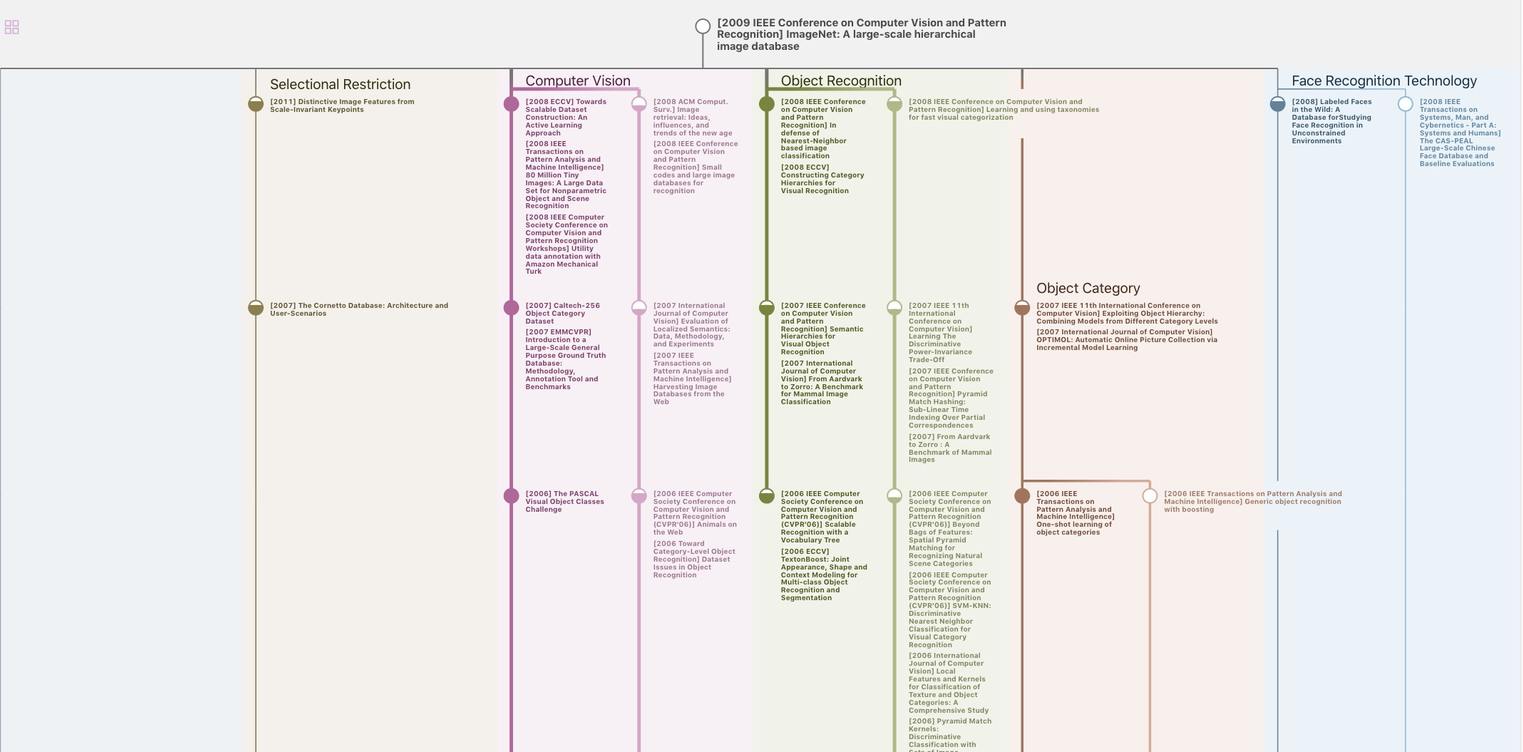
生成溯源树,研究论文发展脉络
Chat Paper
正在生成论文摘要