MERLOT RESERVE: Neural Script Knowledge through Vision and Language and Sound
IEEE Conference on Computer Vision and Pattern Recognition(2022)
摘要
As humans, we navigate a multimodal world, building a holistic understanding from all our senses. We introduce @MERLOT RESERVE, a model that represents videos jointly over time - through a new training objective that learns from audio, subtitles, and video frames. Given a video, we replace snippets of text and audio with a MASK token; the model learns by choosing the correct masked-out snippet. Our objective learns faster than alternatives, and performs well at scale: we pretrain on 20 million YouTube videos. Empirical results show that @MERLOT RESERVE learns strong multimodal representations. When finetuned, it sets state-of-the-art on Visual Commonsense Reasoning (VCR), TVQA, and Kinetics-600; outperforming prior work by 5%, 7%, and 1.5% respectively. Ablations show that these tasks benefit from audio pretraining - even VCR, a QA task centered around images (without sound). Moreover, our objective enables out-of-the-box prediction, revealing strong multimodal commonsense understanding. In a fully zero-shot setting, our model obtains competitive results on four video tasks, even outperforming supervised approaches on the recently proposed Situated Reasoning (STAR) benchmark. We analyze why audio enables better vision-language representations, suggesting significant opportunities for future research. We conclude by discussing ethical and societal implications of multimodal pretraining.
更多查看译文
关键词
Vision + language, Representation learning, Video analysis and understanding, Visual reasoning
AI 理解论文
溯源树
样例
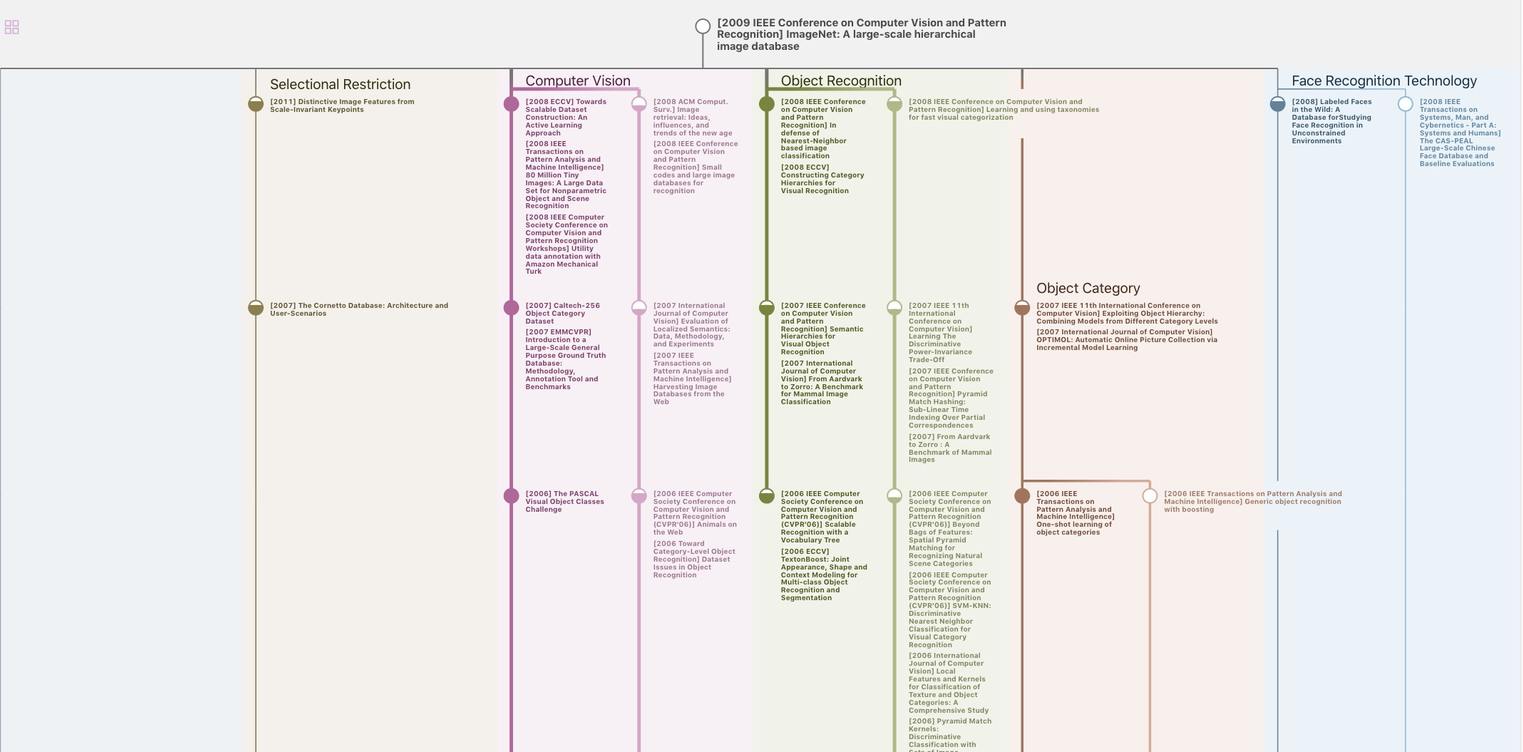
生成溯源树,研究论文发展脉络
Chat Paper
正在生成论文摘要