Integrating contrastive learning with dynamic models for reinforcement learning from images.
Neurocomputing(2022)
摘要
Recent methods for reinforcement learning from images use auxiliary tasks to learn image features that are used by the agent’s policy or Q-function. In particular, methods based on contrastive learning that induce linearity of the latent dynamics or invariance to data augmentation have been shown to greatly improve the sample efficiency of the reinforcement learning algorithm and the generalizability of the learned embedding. We further argue, that explicitly improving Markovianity of the learned embedding is desirable and propose a self-supervised representation learning method which integrates contrastive learning with dynamic models to synergistically combine these three objectives: (1) We maximize the InfoNCE bound on the mutual information between the state- and action-embedding and the embedding of the next state to induce a linearly predictive embedding without explicitly learning a linear transition model, (2) we further improve Markovianity of the learned embedding by explicitly learning a non-linear transition model using regression, and (3) we maximize the mutual information between the two nonlinear predictions of the next embeddings based on the current action and two independent augmentations of the current state, which naturally induces transformation invariance not only for the state embedding, but also for the nonlinear transition model. Experimental evaluation on the Deepmind control suite shows that our proposed method achieves higher sample efficiency and better generalization than state-of-art methods based on contrastive learning or reconstruction.
更多查看译文
关键词
Deep learning in robotics and automation,Reinforcement learning,Contrastive learning,Sensor-based control
AI 理解论文
溯源树
样例
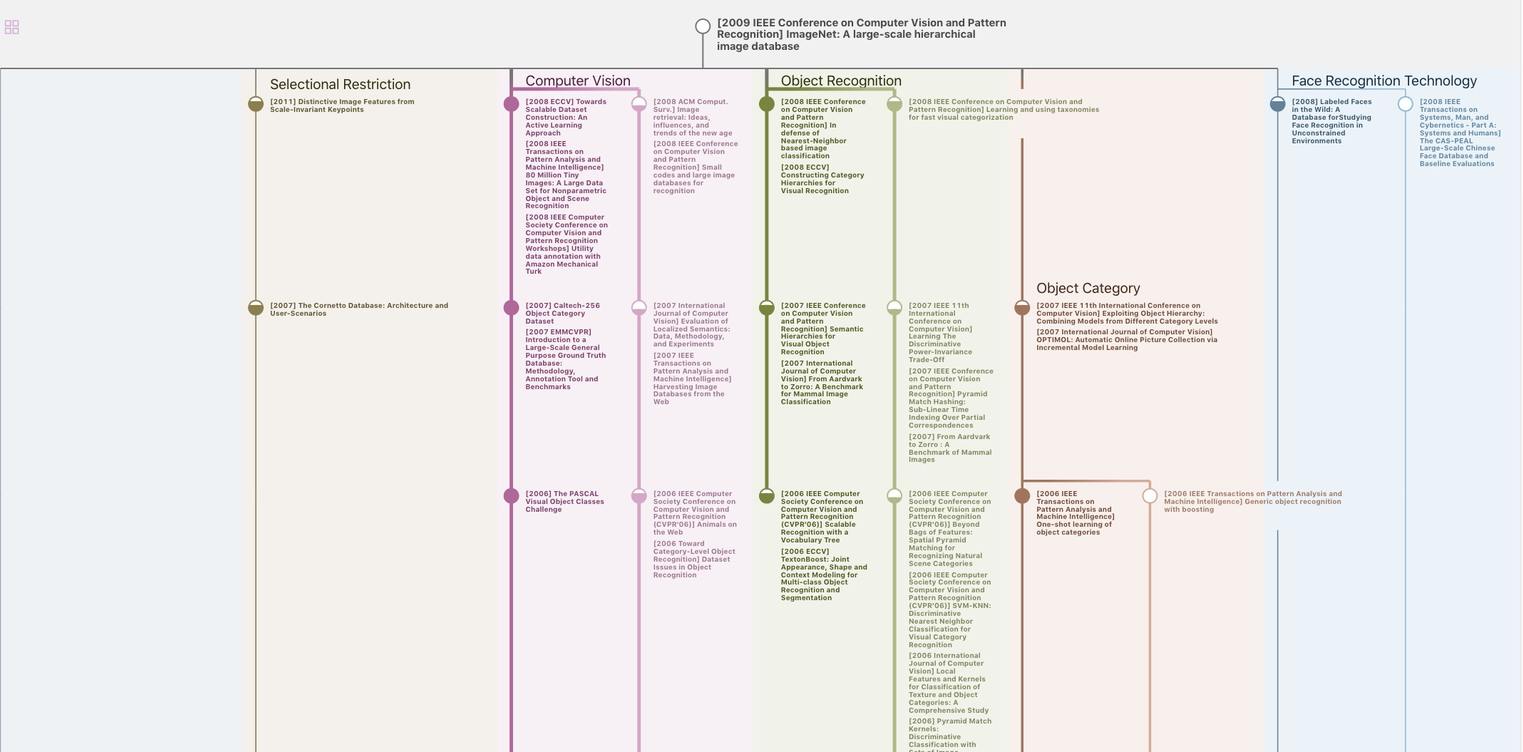
生成溯源树,研究论文发展脉络
Chat Paper
正在生成论文摘要