A Deep Separable Convolutional Neural Network for Multiscale Image-Based Smoke Detection
Fire Technology(2022)
摘要
This paper proposes a multiscale object detection algorithm based on a deep separable convolutional neural network for image-based smoke detection. First, we added a convolution path in the convolution module of the CSPdarknet53 network to widen the backbone network so that it could extract image features more effectively. Then, after the feature layers were output by the backbone network, a space pyramid pool (SPP) module was added to enhance the features of small targets. Finally, depthwise-separable convolution was used to reduce network parameters. Experimental results show that the algorithm was more sensitive to early smoke and achieved an accuracy rate of 98.5% on the smoke dataset, which was 1.1% higher than YOLO V4. The detection speed reached 32 frames/s, which meets the requirements of real-time detection.
更多查看译文
关键词
Smoke detection, Depth separable convolution, Space pyramid pool module, YOLO V4
AI 理解论文
溯源树
样例
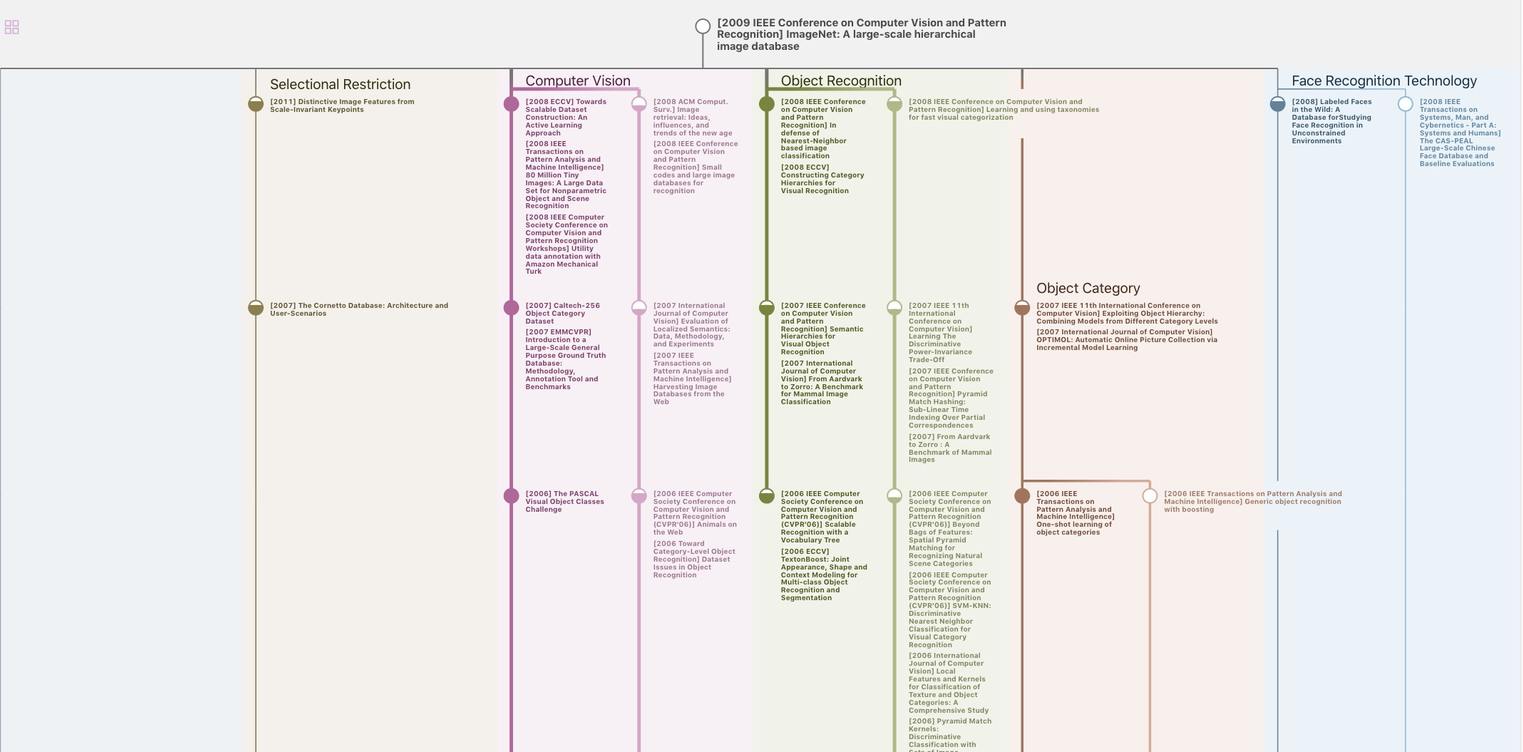
生成溯源树,研究论文发展脉络
Chat Paper
正在生成论文摘要