Data-Efficient Information Extraction from Form-Like Documents
user-618b90cce554220b8f25959c(2021)
摘要
Automating information extraction from form-like documents at scale is a pressing need due to its potential impact on automating business workflows across many industries like financial services, insurance, and healthcare. The key challenge is that form-like documents in these business workflows can be laid out in virtually infinitely many ways; hence, a good solution to this problem should generalize to documents with unseen layouts and languages. A solution to this problem requires a holistic understanding of both the textual segments and the visual cues within a document, which is non-trivial. While the natural language processing and computer vision communities are starting to tackle this problem, there has not been much focus on (1) data-efficiency, and (2) ability to generalize across different document types and languages. In this paper, we show that when we have only a small number of labeled documents for training (~50), a straightforward transfer learning approach from a considerably structurally-different larger labeled corpus yields up to a 27 F1 point improvement over simply training on the small corpus in the target domain. We improve on this with a simple multi-domain transfer learning approach, that is currently in production use, and show that this yields up to a further 8 F1 point improvement. We make the case that data efficiency is critical to enable information extraction systems to scale to handle hundreds of different document-types, and learning good representations is critical to accomplishing this.
更多查看译文
关键词
extraction,information,data-efficient,form-like
AI 理解论文
溯源树
样例
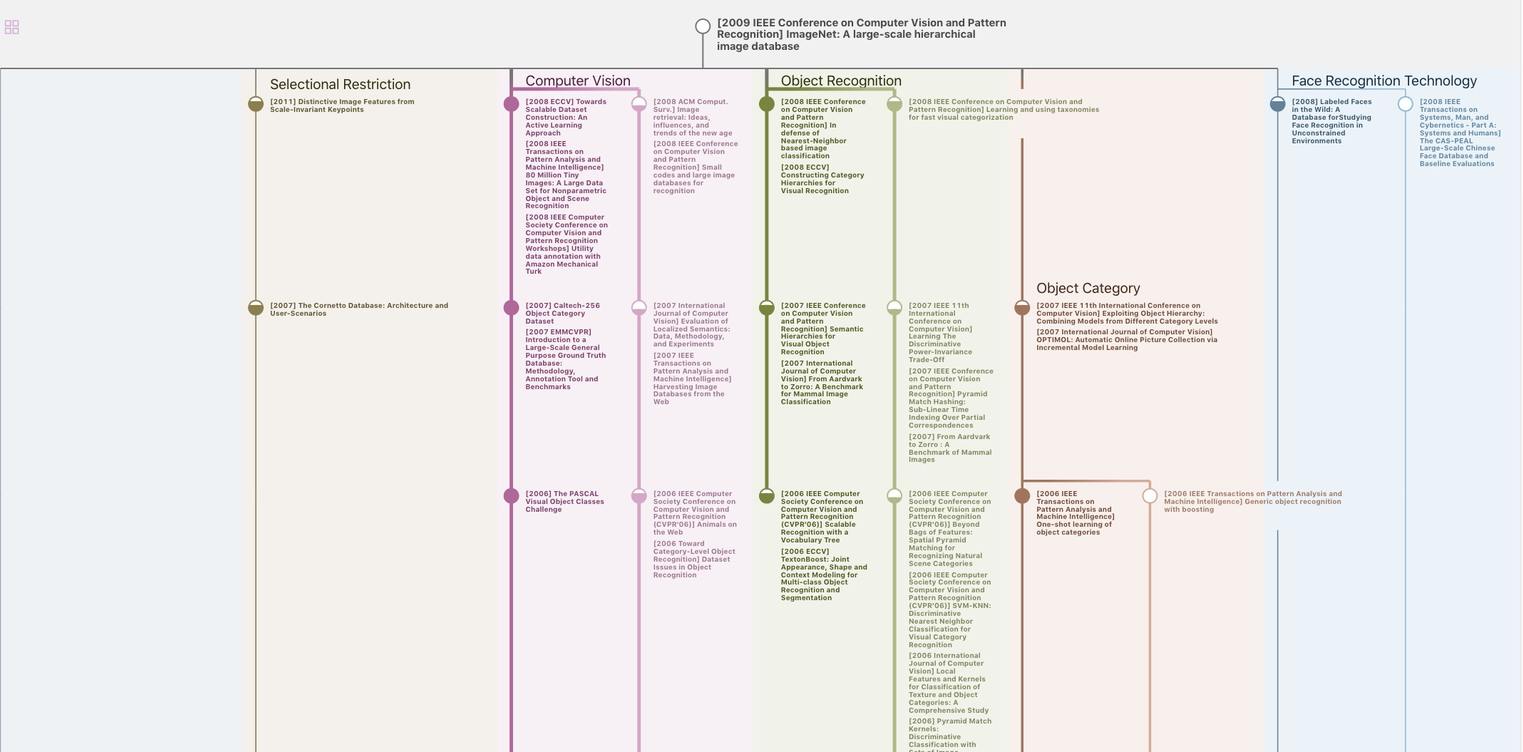
生成溯源树,研究论文发展脉络
Chat Paper
正在生成论文摘要