Differentially Private Hypothesis Testing for Linear Regression
JOURNAL OF MACHINE LEARNING RESEARCH(2023)
摘要
In this work, we design differentially private hypothesis tests for the following problems in the multivariate linear regression model: testing a linear relationship and testing for the presence of mixtures. The majority of our hypothesis tests are based on differentially private versions of the F-statistic for the multivariate linear regression model framework. We also present other differentially private tests-not based on the F-statistic- for these problems. We show that the differentially private F-statistic converges to the asymptotic distribution of its non-private counterpart. As a corollary, the statistical power of the differentially private F-statistic converges to the statistical power of the non-private F- statistic. Through a suite of Monte Carlo based experiments, we show that our tests achieve desired significance levels and have a high power that approaches the power of the non-private tests as we increase sample sizes or the privacy-loss parameter. We also show when our tests outperform existing methods in the literature.
更多查看译文
关键词
differential privacy,linear regression,robust statistics,small-area analysis
AI 理解论文
溯源树
样例
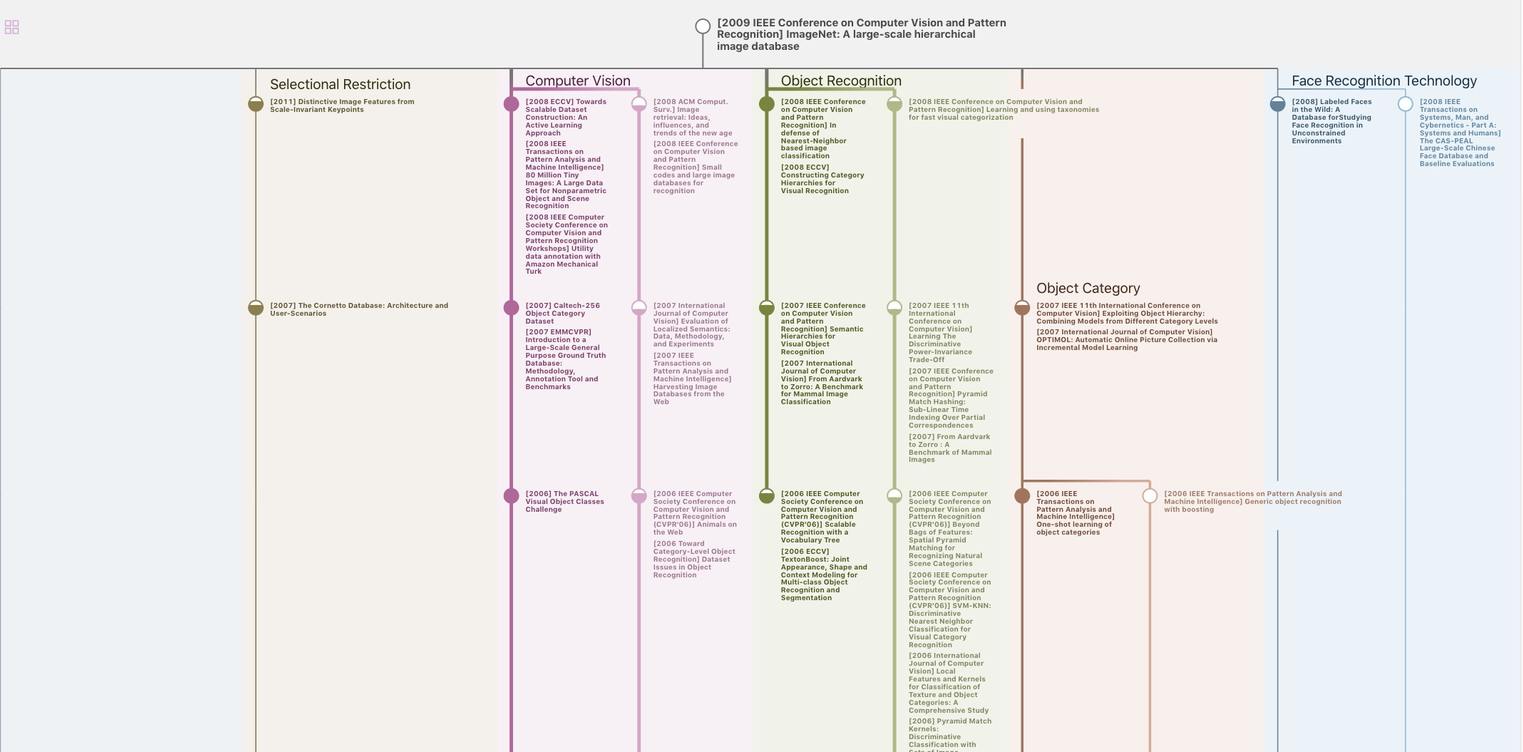
生成溯源树,研究论文发展脉络
Chat Paper
正在生成论文摘要