Self-supervised Variational Autoencoder for Recommender Systems
2021 IEEE 33RD INTERNATIONAL CONFERENCE ON TOOLS WITH ARTIFICIAL INTELLIGENCE (ICTAI 2021)(2021)
摘要
Variational autoencoder (VAE) is considered as an emerging model for ensuring competitive performance in recom-mender systems. However, its performance is severely limited by the amount of training examples and, as a result, existing VAE models may fail to provide satisfactory recommendation results in presence of highly sparse user-item interactions. In this paper, we propose a self-supervised VAE model, SSVAE in short, to improve the generalization ability of VAE model on the sparse interaction datasets. Concretely, we first build multiple views for each user by data augmentation, and then design a pretext task to align the representations learned from different views of each user. Particularly, SSVAE aims to optimize a combined objective of recommendation task and pretext task, making them to rein-force each other during the learning process. Our encouraging experimental results on three real-world benchmarks validate the superiority of our SSVAE model to state-of-the-art VAE style recommendation techniques.
更多查看译文
关键词
Recommender Systems, Self-supervised Learning, Variational Autoencoder
AI 理解论文
溯源树
样例
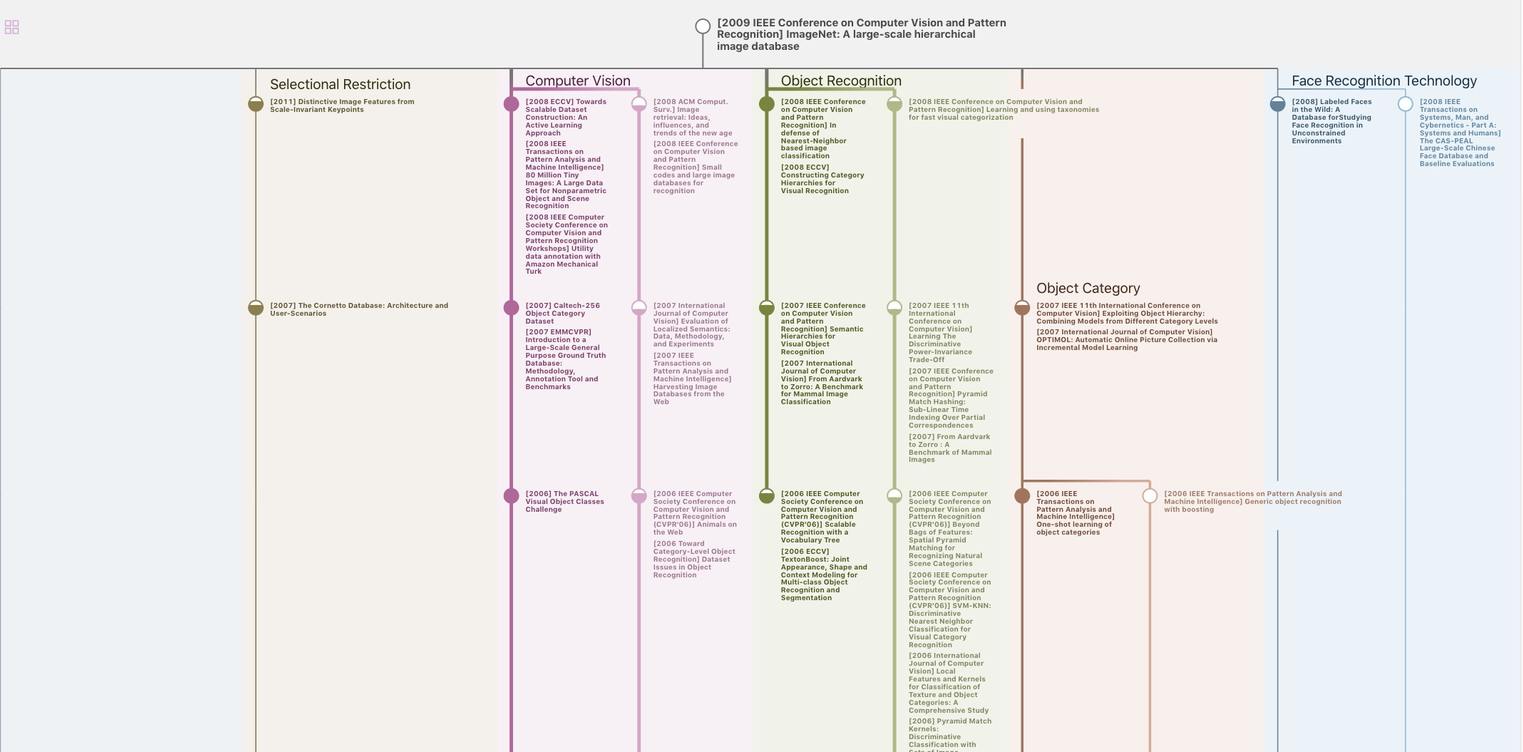
生成溯源树,研究论文发展脉络
Chat Paper
正在生成论文摘要