FEDBS: Learning on Non-IID Data in Federated Learning using Batch Normalization
2021 IEEE 33RD INTERNATIONAL CONFERENCE ON TOOLS WITH ARTIFICIAL INTELLIGENCE (ICTAI 2021)(2021)
摘要
Federated learning (FL) is a well-established distributed machine-learning paradigm that enables training global models on massively distributed data i.e., training on multiowner data. However, classic FL algorithms, such as Federated Averaging (FedAvg), generally underperform when faced with Non-Independent and Identically Distributed (Non-IID) data. Such a problem is aggravated for some hyperparametric methods such as optimizers, regularization, and normalization techniques. In this paper, we introduce FedBS, a new efficient strategy to handle global models having batch normalization layers, in the presence of Non-HD data. FedBS modifies FedAvg by introducing a new aggregation rule at the server-side, while also retaining full compatibility with Batch Normalization (BN). Through our evaluations, we have empirically proven that FedBS outperforms both classical FedAvg, as well as the state-of-the-art FedProx through a comprehensive set of experiments conducted on Cifar10, Mnist, and Fashion-Mnist datasets under various Non-HD data settings. Furthermore, we observed that in some cases, FedBS can be 2x faster than other FL approaches, coupled with higher testing accuracy.
更多查看译文
关键词
Federated learning, Batch Normalization, Non-HD data
AI 理解论文
溯源树
样例
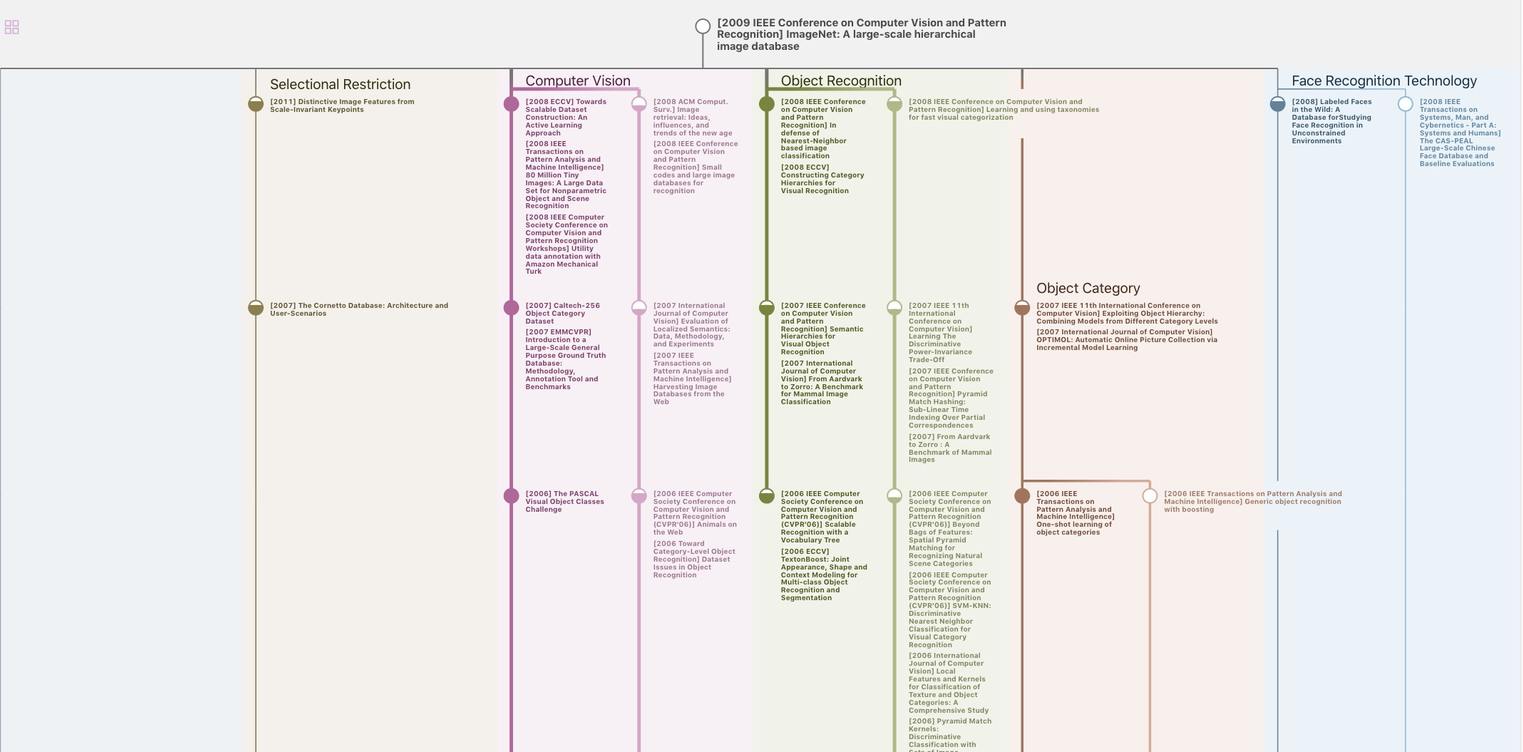
生成溯源树,研究论文发展脉络
Chat Paper
正在生成论文摘要