A Scalable Short-Text Clustering Algorithm Using Apache Spark
2021 IEEE 33RD INTERNATIONAL CONFERENCE ON TOOLS WITH ARTIFICIAL INTELLIGENCE (ICTAI 2021)(2021)
摘要
Short text clustering deals with the problem of grouping together semantically similar documents with small lengths. Nowadays, huge amounts of text data is being generated by numerous applications such as microblogs, messengers, and services that generate or aggregate entitled entities. This large volume of highly dimensional and sparse information may easily overwhelm the current serial approaches and render them inefficient, or even inapplicable. Although many traditional clustering algorithms have been successfully parallelized in the past, the parallelization of short text clustering algorithms is a rather overlooked problem. In this paper we introduce pVEPHC, a short text clustering method that can be executed in parallel in large computer clusters. The algorithm draws inspiration from VEPHC, a recent two-stage approach with decent performance in several diverse tasks. More specifically, in this work we employ the Apache Spark framework to design parallel implementations of both stages of VEPHC. During the first stage, pVEPHC generates an initial clustering by identifying and modelling common low-dimensional vector representations of the original documents. In the sequel, the initial clustering is improved in the second stage by applying cluster split and merge operations in a hierarchical fashion. We have attested our implementation on an experimental Spark cluster and we report an almost linear improvement in the execution times of the algorithm.
更多查看译文
关键词
short text clustering, clustering, machine learning, big data, parallel algorithms, Spark
AI 理解论文
溯源树
样例
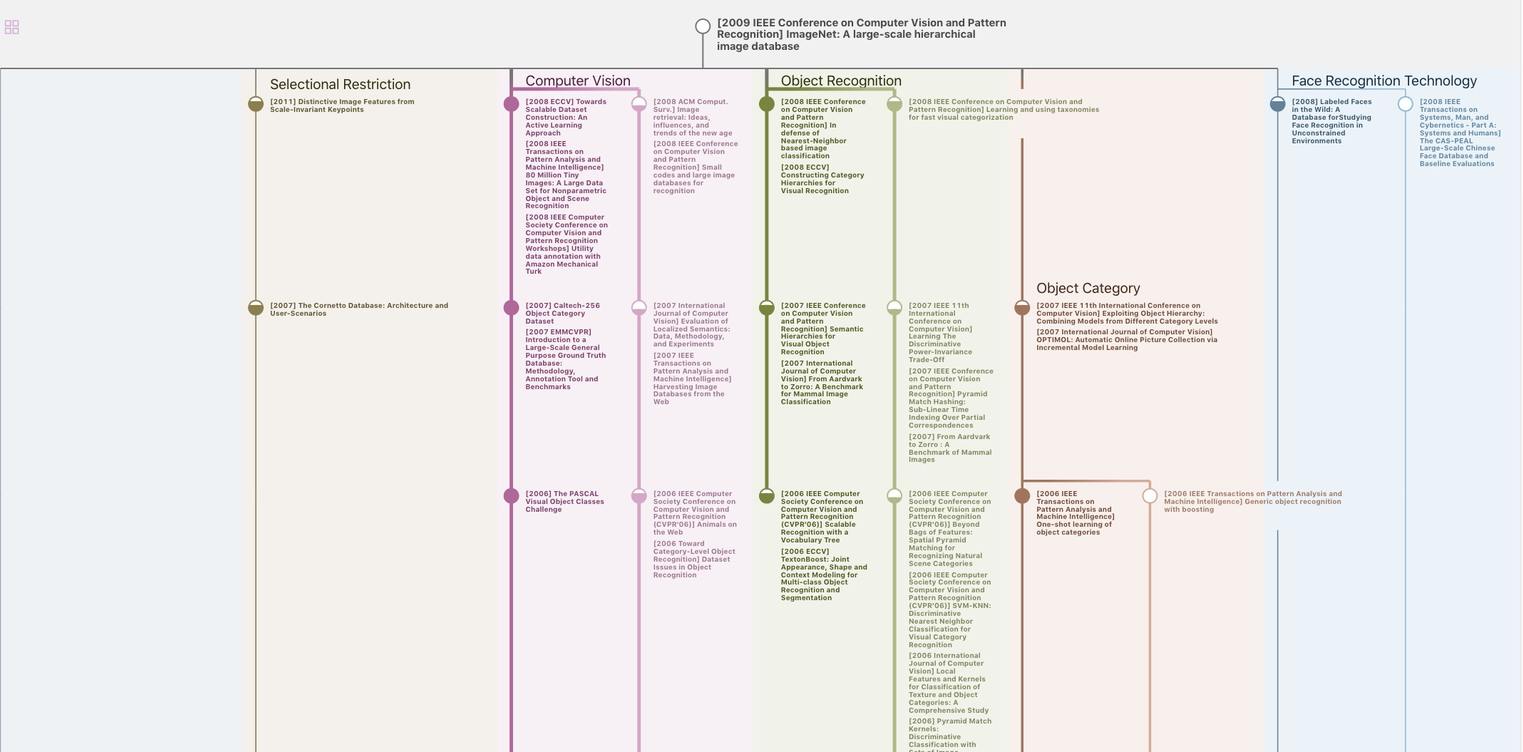
生成溯源树,研究论文发展脉络
Chat Paper
正在生成论文摘要