Horizontal Auto-Scaling for Multi-Access Edge Computing Using Safe Reinforcement Learning
ACM Transactions on Embedded Computing Systems(2021)
摘要
AbstractMulti-Access Edge Computing (MEC) has emerged as a promising new paradigm allowing low latency access to services deployed on edge servers to avert network latencies often encountered in accessing cloud services. A key component of the MEC environment is an auto-scaling policy which is used to decide the overall management and scaling of container instances corresponding to individual services deployed on MEC servers to cater to traffic fluctuations. In this work, we propose a Safe Reinforcement Learning (RL)-based auto-scaling policy agent that can efficiently adapt to traffic variations to ensure adherence to service specific latency requirements. We model the MEC environment using a Markov Decision Process (MDP). We demonstrate how latency requirements can be formally expressed in Linear Temporal Logic (LTL). The LTL specification acts as a guide to the policy agent to automatically learn auto-scaling decisions that maximize the probability of satisfying the LTL formula. We introduce a quantitative reward mechanism based on the LTL formula to tailor service specific latency requirements. We prove that our reward mechanism ensures convergence of standard Safe-RL approaches. We present experimental results in practical scenarios on a test-bed setup with real-world benchmark applications to show the effectiveness of our approach in comparison to other state-of-the-art methods in literature. Furthermore, we perform extensive simulated experiments to demonstrate the effectiveness of our approach in large scale scenarios.
更多查看译文
关键词
Multi-access edge computing,safe reinforcement learning,auto-scaling
AI 理解论文
溯源树
样例
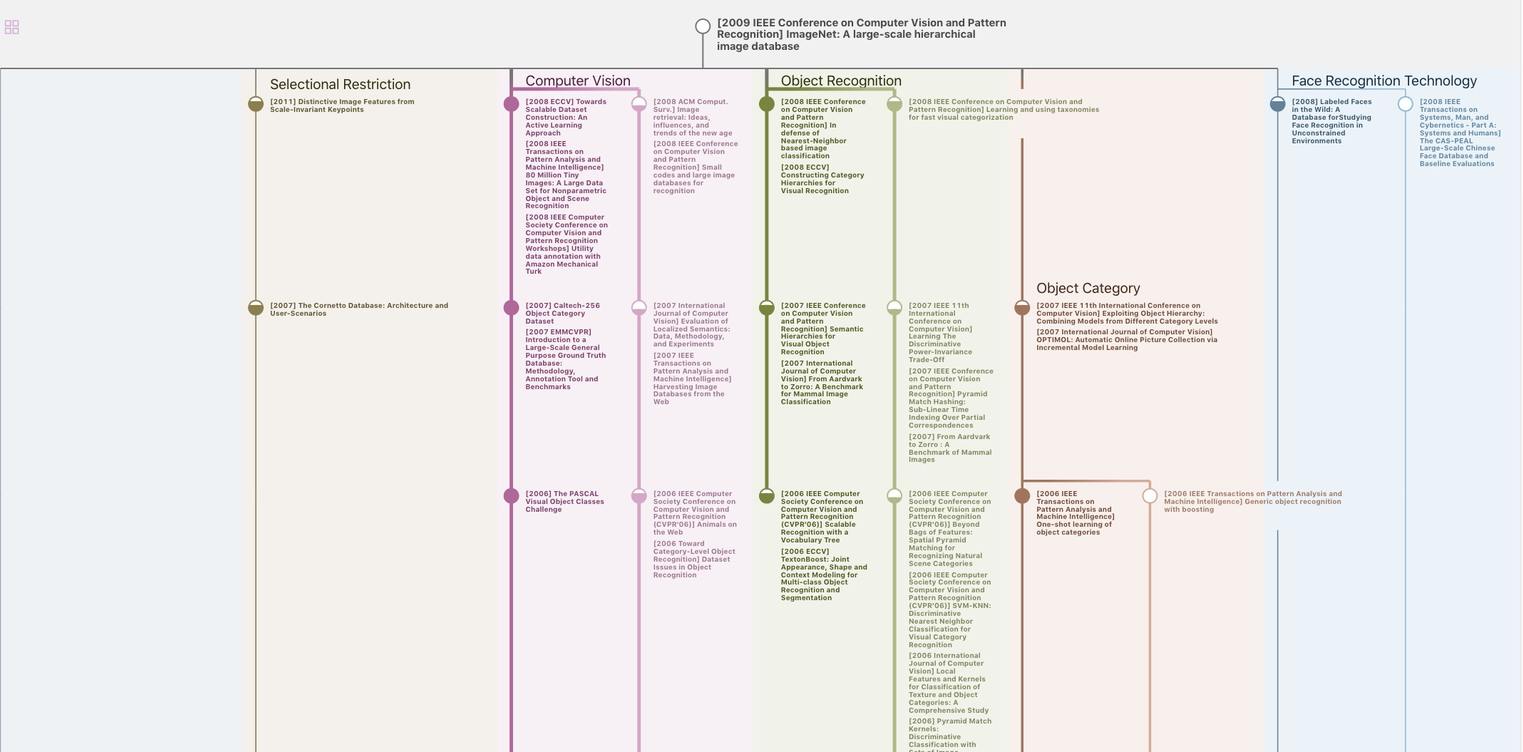
生成溯源树,研究论文发展脉络
Chat Paper
正在生成论文摘要