Using mathematical modelling to identify data requirements for increased prediction accuracy in radiotherapy
arxiv(2022)
摘要
Longitudinal gross tumour volume (GTV) clinical data from head and neck cancer patients show that tumours of a similar size and stage pre-treatment may respond very differently to the same radiotherapy fractionation protocol. Mathematical models of radiation response are often proposed as a means to predict treatment outcome and prescribe more personalised fractionation protocols. Predictive mathematical models aimed towards clinical applications should be sufficiently detailed to capture the range of dynamics observed in vivo while being sufficiently simple such that the model parameters are identifiable with respect to the data typically available for model calibration. In this paper we show that models describing the spatiotemporal heterogeneity of the intratumoural composition may better capture observed in vivo dynamics than those describing the evolution of the tumour volume alone. We compare the range of qualitative responses with respect to GTV data throughout radiotherapy treatment to those observed in a cohort of head and neck cancer patients. Synthetic data studies are used to address questions of identifiability and uncertainty quantification. Our results argue that, to develop accurate, predictive mathematical models, there is a simultaneous need for more detailed models (possibly incorporating some notion of tumour heterogeneity) along with more sophisticated data metrics with which to inform such models.
更多查看译文
AI 理解论文
溯源树
样例
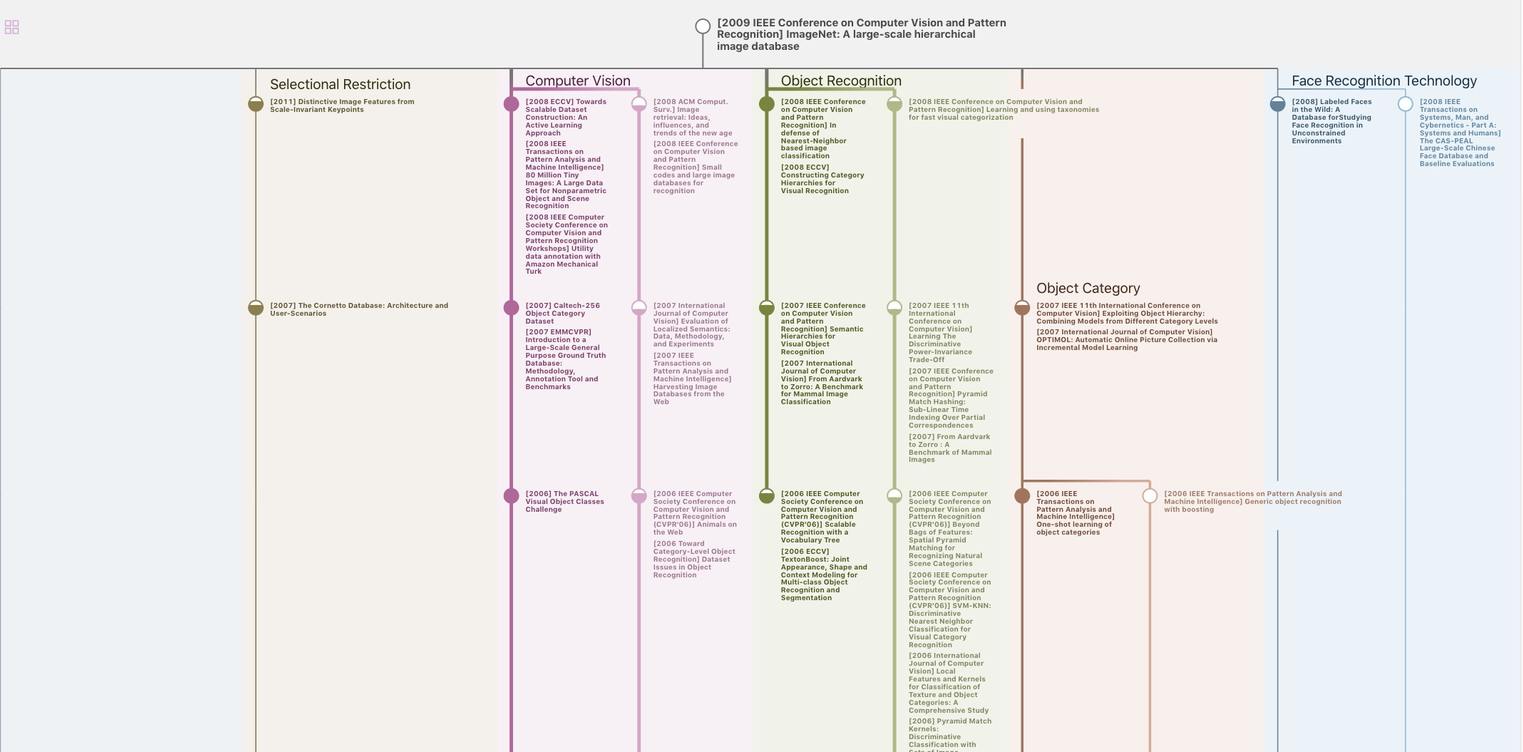
生成溯源树,研究论文发展脉络
Chat Paper
正在生成论文摘要