Enhancing Egocentric 3D Pose Estimation with Third Person Views
arxiv(2022)
摘要
In this paper, we propose a novel approach to enhance the 3D body pose estimation of a person computed from videos captured from a single wearable camera. The key idea is to leverage high-level features linking first- and third-views in a joint embedding space. To learn such embedding space we introduce First2Third-Pose, a new paired synchronized dataset of nearly 2,000 videos depicting human activities captured from both first- and third-view perspectives. We explicitly consider spatial- and motion-domain features, combined using a semi-Siamese architecture trained in a self-supervised fashion. Experimental results demonstrate that the joint multi-view embedded space learned with our dataset is useful to extract discriminatory features from arbitrary single-view egocentric videos, without needing domain adaptation or knowledge of camera parameters. We achieve significant improvement of egocentric 3D body pose estimation performance on two unconstrained datasets, over three supervised state-of-the-art approaches. Our dataset and code will be available for research purposes.
更多查看译文
关键词
3D pose estimation,Self-supervised learning,Egocentric vision
AI 理解论文
溯源树
样例
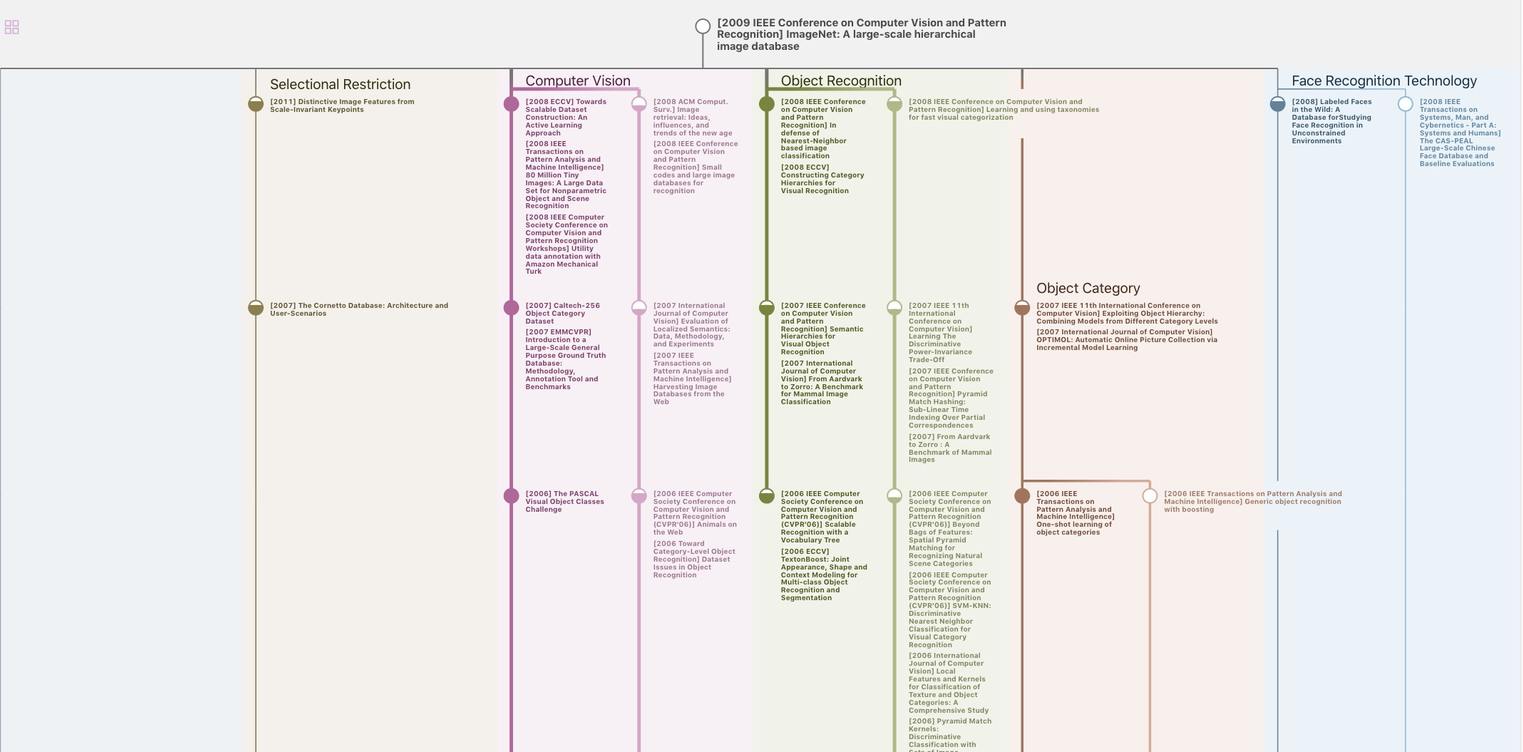
生成溯源树,研究论文发展脉络
Chat Paper
正在生成论文摘要