Sparsity-based Feature Selection for Anomalous Subgroup Discovery
arxiv(2022)
摘要
Anomalous pattern detection aims to identify instances where deviation from normalcy is evident, and is widely applicable across domains. Multiple anomalous detection techniques have been proposed in the state of the art. However, there is a common lack of a principled and scalable feature selection method for efficient discovery. Existing feature selection techniques are often conducted by optimizing the performance of prediction outcomes rather than its systemic deviations from the expected. In this paper, we proposed a sparsity-based automated feature selection (SAFS) framework, which encodes systemic outcome deviations via the sparsity of feature-driven odds ratios. SAFS is a model-agnostic approach with usability across different discovery techniques. SAFS achieves more than $3\times$ reduction in computation time while maintaining detection performance when validated on publicly available critical care dataset. SAFS also results in a superior performance when compared against multiple baselines for feature selection.
更多查看译文
关键词
feature selection,discovery,sparsity-based
AI 理解论文
溯源树
样例
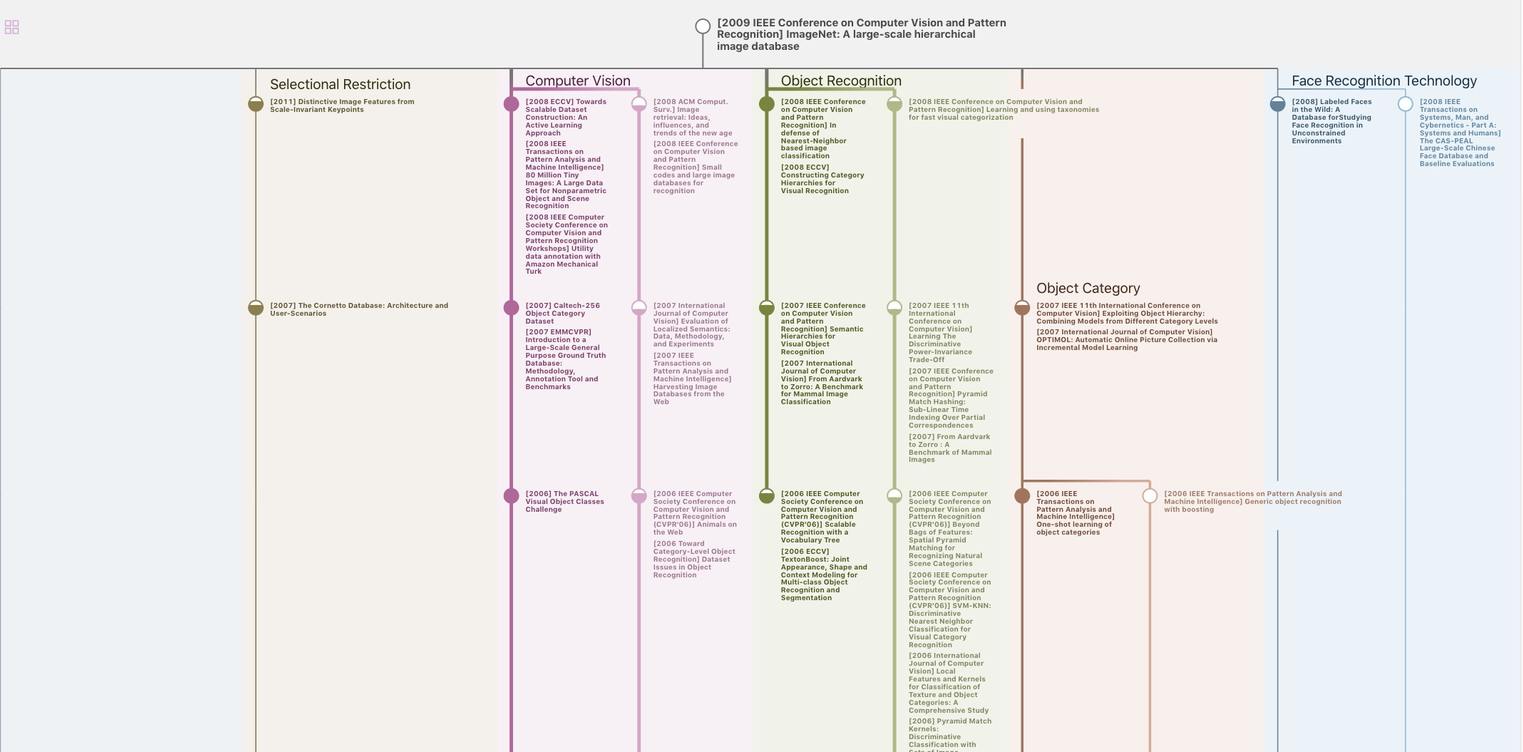
生成溯源树,研究论文发展脉络
Chat Paper
正在生成论文摘要