Elastic Product Quantization for Time Series.
International Conference on Discovery Science (DS)(2022)
摘要
Analyzing numerous or long time series is difficult in practice due to the high storage costs and computational requirements. Therefore, techniques have been proposed to generate compact similarity-preserving representations of time series, enabling real-time similarity search on large in-memory data collections. However, the existing techniques are not ideally suited for assessing similarity when sequences are locally out of phase. In this paper, we propose the use of product quantization for efficient similarity-based comparison of time series under time warping. The idea is to first compress the data by partitioning the time series into equal length sub-sequences which are represented by a short code. The distance between two time series can then be efficiently approximated by pre-computed elastic distances between their codes. The partitioning into sub-sequences forces unwanted alignments, which we address with a pre-alignment step using the maximal overlap discrete wavelet transform (MODWT). To demonstrate the efficiency and accuracy of our method, we perform an extensive experimental evaluation on benchmark datasets in nearest neighbors classification and clustering applications. Overall, the proposed solution emerges as a highly efficient (both in terms of memory usage and computation time) replacement for elastic measures in time series applications.
更多查看译文
关键词
elastic product quantization,time series
AI 理解论文
溯源树
样例
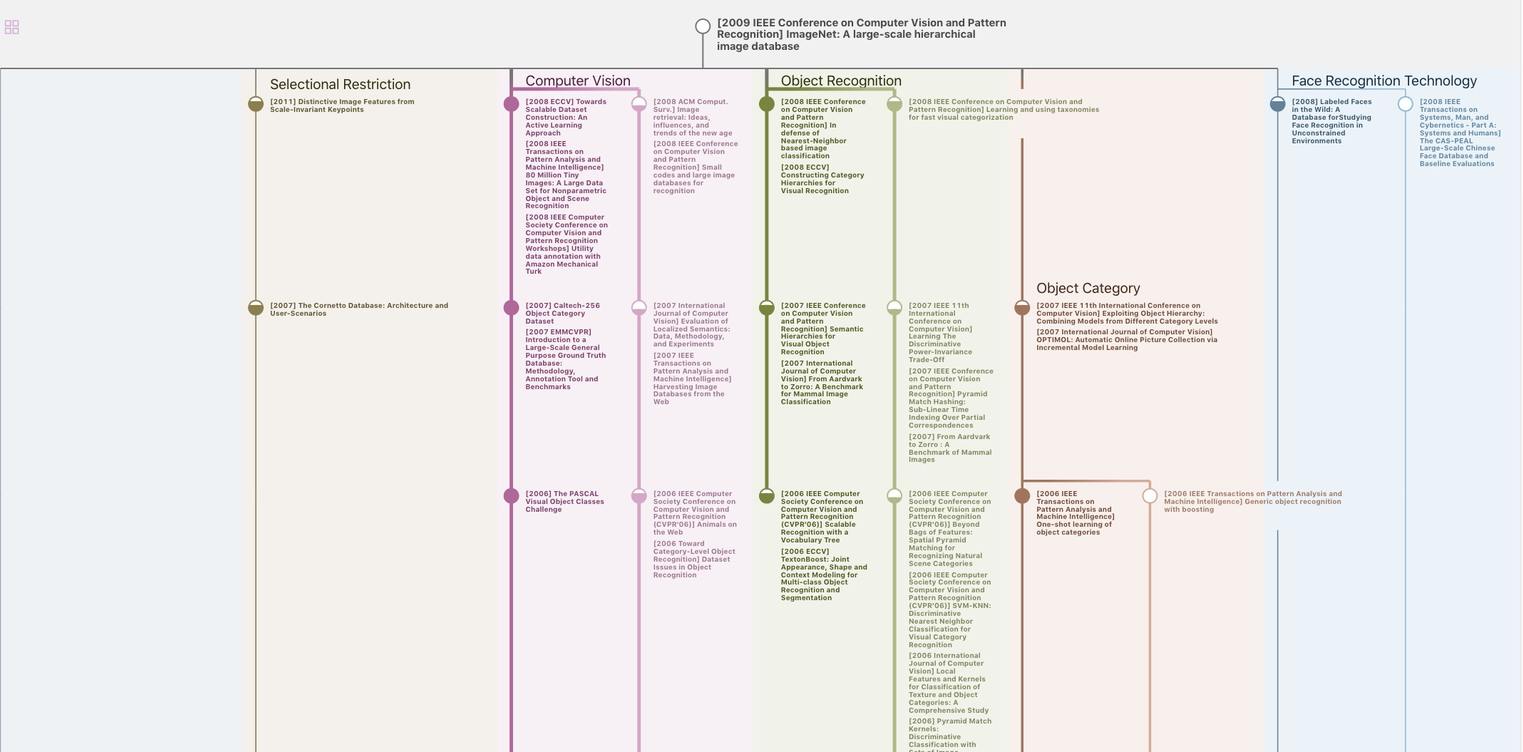
生成溯源树,研究论文发展脉络
Chat Paper
正在生成论文摘要