Self-Organizing Interval Type-2 Fuzzy Neural Network Using Information Aggregation Method
IEEE transactions on neural networks and learning systems(2023)
摘要
Interval type-2 fuzzy neural networks (IT2FNNs) usually stack adequate fuzzy rules to identify nonlinear systems with high-dimensional inputs, which may result in an explosion of fuzzy rules. To cope with this problem, a self-organizing IT2FNN, based on the information aggregation method (IA-SOIT2FNN), is developed to avoid the explosion of fuzzy rules in this article. First, a relation-aware strategy is proposed to construct rotatable type-2 fuzzy rules (RT2FRs). This strategy uses the individual RT2FR, instead of multiple standard fuzzy rules, to interpret interactive features of high-dimensional inputs. Second, a comprehensive information evaluation mechanism, associated with the interval information and rotation information of RT2FR, is developed to direct the structural adjustment of IA-SOIT2FNN. This mechanism can achieve a compact structure of IA-SOIT2FNN by growing and pruning RT2FRs. Third, a multicriteria-based optimization algorithm is designed to optimize the parameters of IA-SOIT2FNN. The algorithm can simultaneously update the rotatable parameters and the conventional parameters of RT2FR, and further maintain the accuracy of IA-SOIT2FNN. Finally, the experiments showcase that the proposed IA-SOIT2FNN can compete with the state-of-the-art approaches in terms of identification performance.
更多查看译文
关键词
Optimization,Neurons,Fuzzy neural networks,Explosions,Uncertainty,Genetic algorithms,Standards,Comprehensive information evaluation mechanism (CIM),fuzzy rule explosion,interval type-2 fuzzy neural network (IT2FNN),multicriteria-based optimization algorithm (MOA),rotatable type-2 fuzzy rules (RT2FRs)
AI 理解论文
溯源树
样例
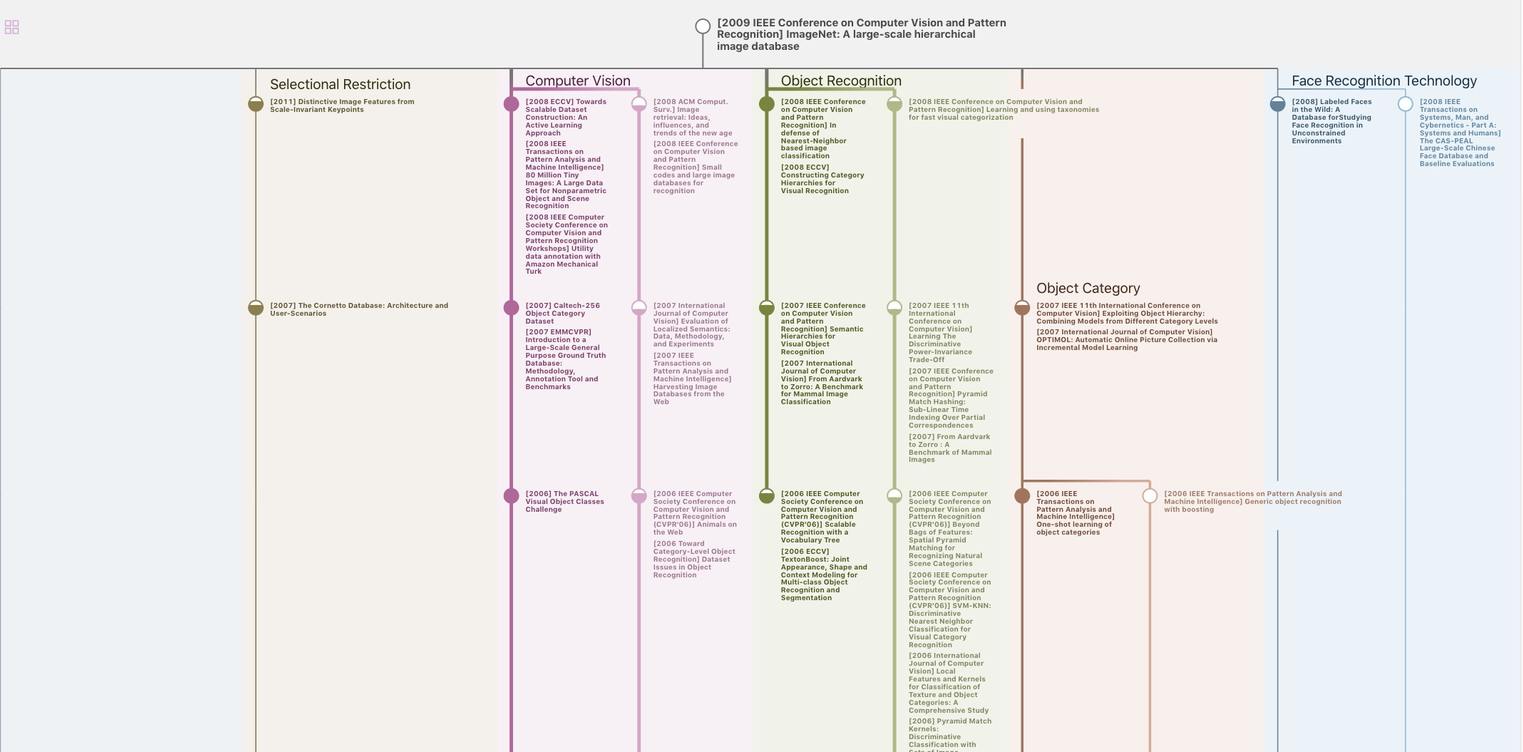
生成溯源树,研究论文发展脉络
Chat Paper
正在生成论文摘要