Fusing Convolutional Neural Network and Geometric Constraint for Image-Based Indoor Localization
IEEE ROBOTICS AND AUTOMATION LETTERS(2022)
摘要
This letter proposes a new image-based localization framework that explicitly localizes the camera/robot by fusing Convolutional Neural Network (CNN) and sequential images' geometric constraints. The camera is localized using a single or few observed images and training images with 6-degree-of-freedom pose labels. A Siamese network structure is adopted to train an image descriptor network, and the visually similar candidate image in the training set is retrieved to localize the testing image geometrically. Meanwhile, a probabilistic motion model predicts the pose based on a constant velocity assumption. The two estimated poses are finally fused using their uncertainties to yield an accurate pose prediction. This method leverages the geometric uncertainty and is applicable in indoor scenarios predominated by diffuse illumination. Experiments on simulation and real data sets demonstrate the efficiency of our proposed method. The results further show that combining the CNN-based framework with geometric constraint achieves better accuracy when compared with CNN-only methods, especially when the training data size is small.
更多查看译文
关键词
Vision-based navigation, localization, probability and statistical methods
AI 理解论文
溯源树
样例
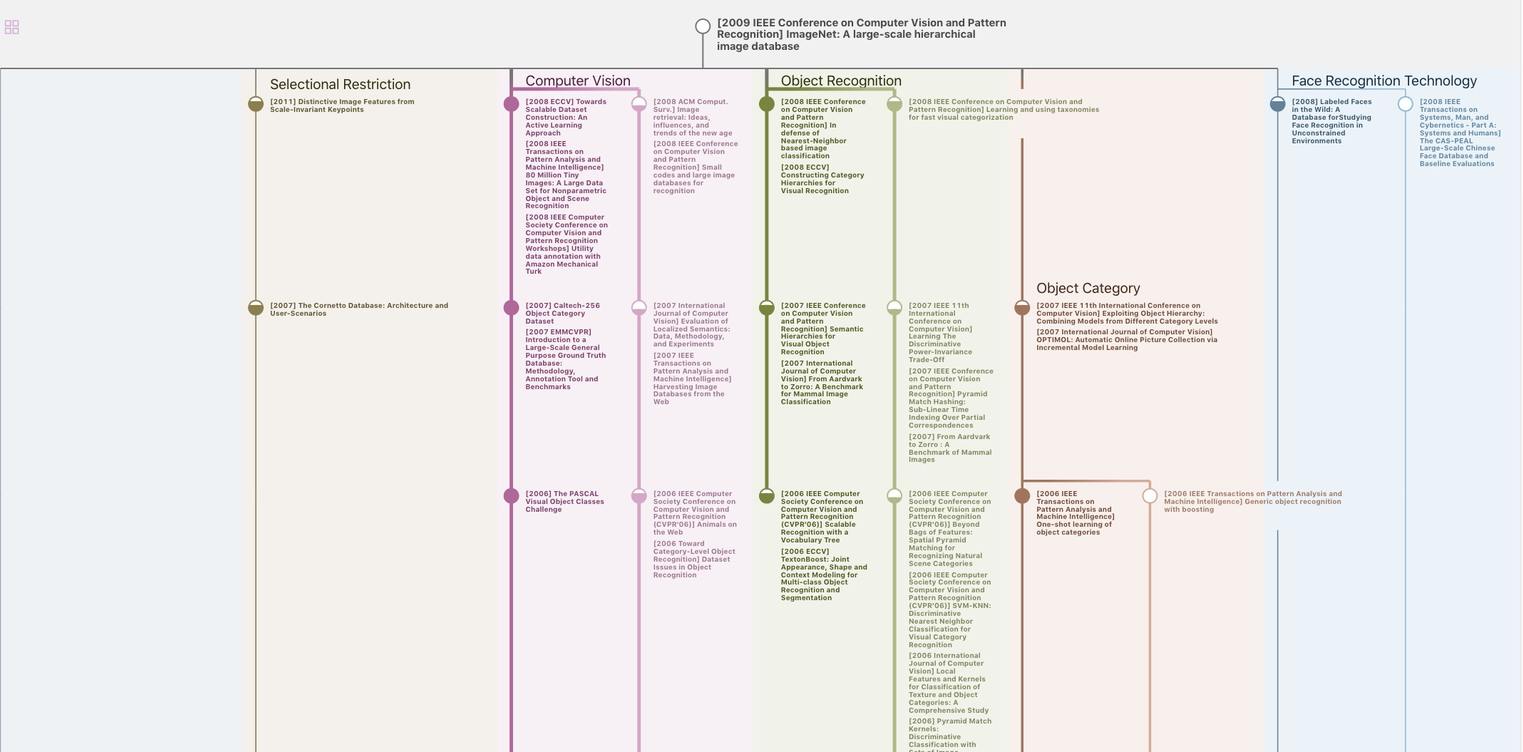
生成溯源树,研究论文发展脉络
Chat Paper
正在生成论文摘要