Linear Variational State Space Filtering
arxiv(2022)
摘要
We introduce Variational State-Space Filters (VSSF), a new method for unsupervised learning, identification, and filtering of latent Markov state space models from raw pixels. We present a theoretically sound framework for latent state space inference under heterogeneous sensor configurations. The resulting model can integrate an arbitrary subset of the sensor measurements used during training, enabling the learning of semi-supervised state representations, thus enforcing that certain components of the learned latent state space to agree with interpretable measurements. From this framework we derive L-VSSF, an explicit instantiation of this model with linear latent dynamics and Gaussian distribution parameterizations. We experimentally demonstrate L-VSSF's ability to filter in latent space beyond the sequence length of the training dataset across several different test environments.
更多查看译文
关键词
state-space
AI 理解论文
溯源树
样例
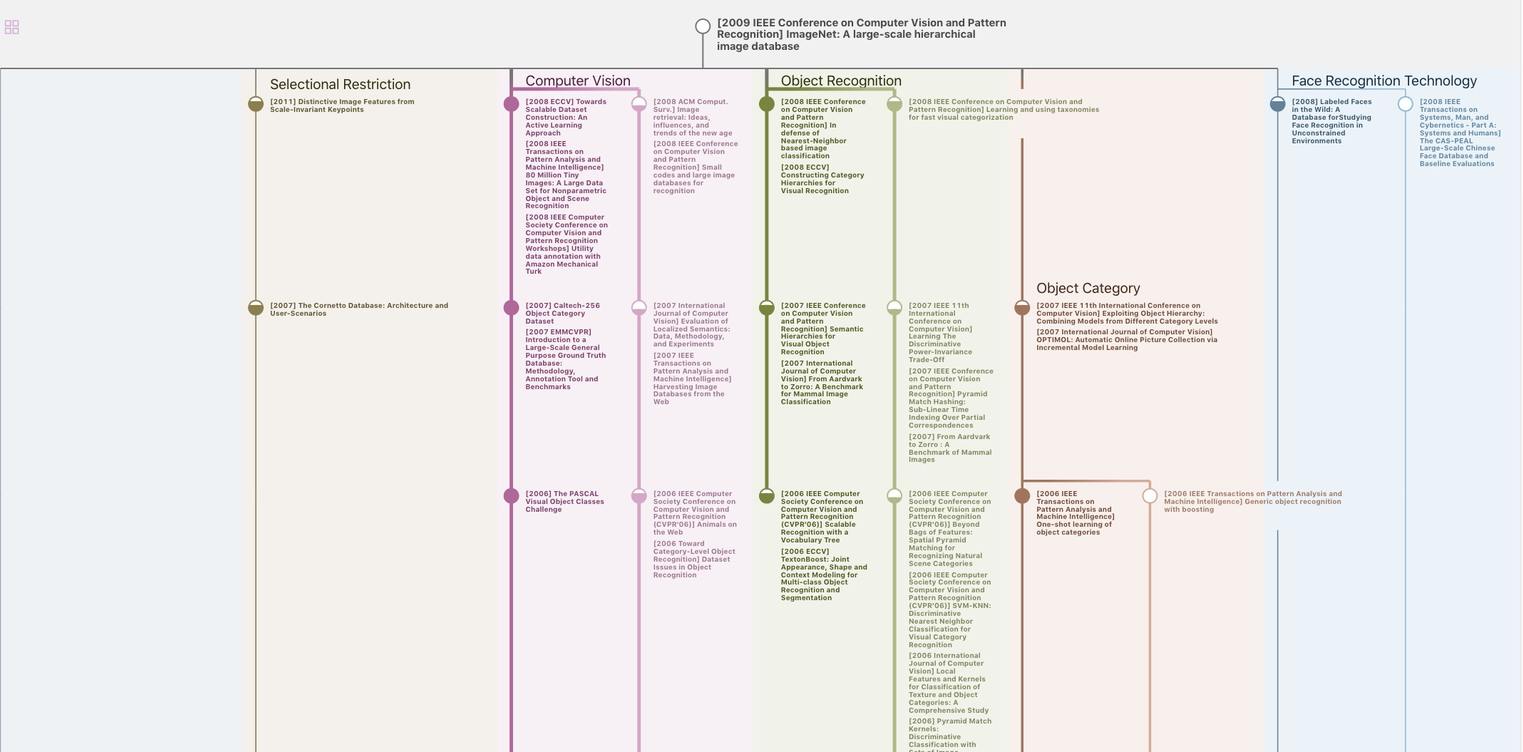
生成溯源树,研究论文发展脉络
Chat Paper
正在生成论文摘要