Deep learning based cervical screening by the cross-modal integration of colposcopy, cytology, and HPV test
International Journal of Medical Informatics(2022)
摘要
Purpose
To develop and evaluate the colposcopy based deep learning model using all kinds of cervical images for cervical screening, and investigate the synergetic benefits of the colposcopy, the cytology test, and the HPV test for improving cervical screening performance.
Methods
This study consisted of 2160 women who underwent cervical screening, there were 442 cases with the histopathological confirmed high-grade squamous intraepithelial lesion (HSIL) or cancer, and the remained 1718 women were controls. Three kinds of cervical images were acquired from colposcopy including the saline image of cervix after saline irrigation, the acetic acid image of cervix after applying acetic acid solution, and the iodine image of cervix after applying Lugol’s iodine solution. Each kind of image was used to build a single-image based deep learning model by the VGG-16 convolutional neural network, respectively. A multiple-images based deep learning model was built using multivariable logistic regression (MLR) by combining the single-image based models. The performance of the visual inspection was also obtained. The results of the cytology test and HPV test were used to build a Cytology-HPV joint diagnostic model by MLR. Finally, a cross-modal integrated model was built using MLR by combining the multiple-images based deep learning model, the cytology test results, and the HPV test results. The performances of models were tested in an independent test set using the area under the receiver operating characteristic curve (AUC).
Results
The saline image, acetic acid image, and iodine image based deep learning models had AUC of 0.760, 0.791, and 0.840. The multiple-images based deep learning model achieved an improved AUC of 0.845. The AUC of the visual inspection was 0.751. The Cytology-HPV joint diagnostic model had an AUC of 0.837, which was higher than the cytology test (AUC = 0.749) and the HPV test (AUC = 0.742). The cross-modal integrated model achieved the best performance with AUC of 0.921.
Conclusions
Combining all kinds of cervical images were benefit for improving the performance of the colposcopy based deep learning model, and more accurate cervical screening could be achieved by incorporating the colposcopy based deep learning model, the cytology test results, and the HPV test results.
更多查看译文
关键词
Deep learning,Cervical screening,Colposcopy,Cytology,HPV
AI 理解论文
溯源树
样例
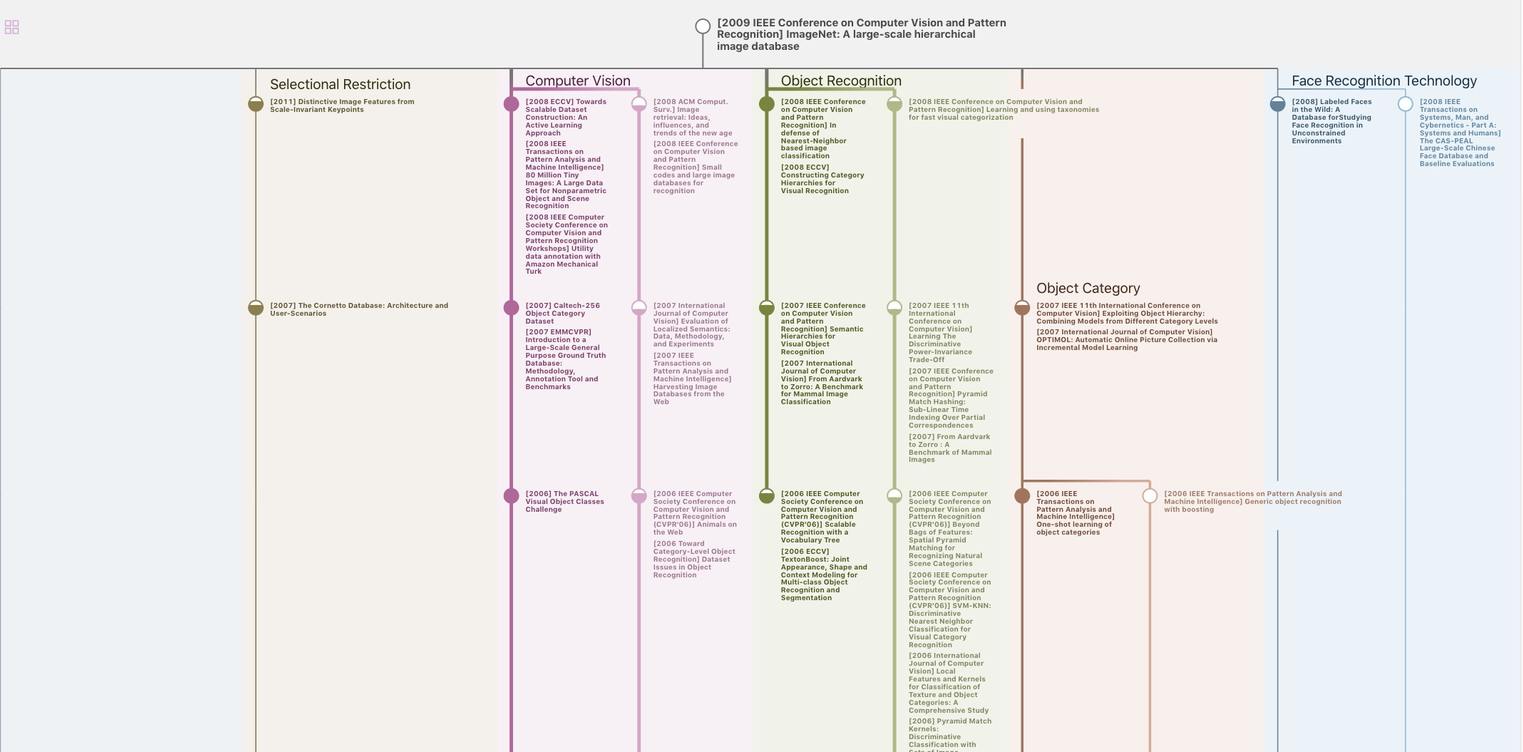
生成溯源树,研究论文发展脉络
Chat Paper
正在生成论文摘要