Combining weighted SMOTE with ensemble learning for the class-imbalanced prediction of small business credit risk
Complex & Intelligent Systems(2022)
摘要
In small business credit risk assessment, the default and nondefault classes are highly imbalanced. To overcome this problem, this study proposes an extended ensemble approach rooted in the weighted synthetic minority oversampling technique (WSMOTE), which is called WSMOTE-ensemble. The proposed ensemble classifier hybridizes WSMOTE and Bagging with sampling composite mixtures to guarantee the robustness and variability of the generated synthetic instances and, thus, minimize the small business class-skewed constraints linked to default and nondefault instances. The original small business dataset used in this study was taken from 3111 records from a Chinese commercial bank. By implementing a thorough experimental study of extensively skewed data-modeling scenarios, a multilevel experimental setting was established for a rare event domain. Based on the proper evaluation measures, this study proposes that the random forest classifier used in the WSMOTE-ensemble model provides a good trade-off between the performance on default class and that of nondefault class. The ensemble solution improved the accuracy of the minority class by 15.16% in comparison with its competitors. This study also shows that sampling methods outperform nonsampling algorithms. With these contributions, this study fills a noteworthy knowledge gap and adds several unique insights regarding the prediction of small business credit risk.
更多查看译文
关键词
Small business,Credit risk,Imbalanced data,Oversampling,Weighted SMOTE,Ensemble learning
AI 理解论文
溯源树
样例
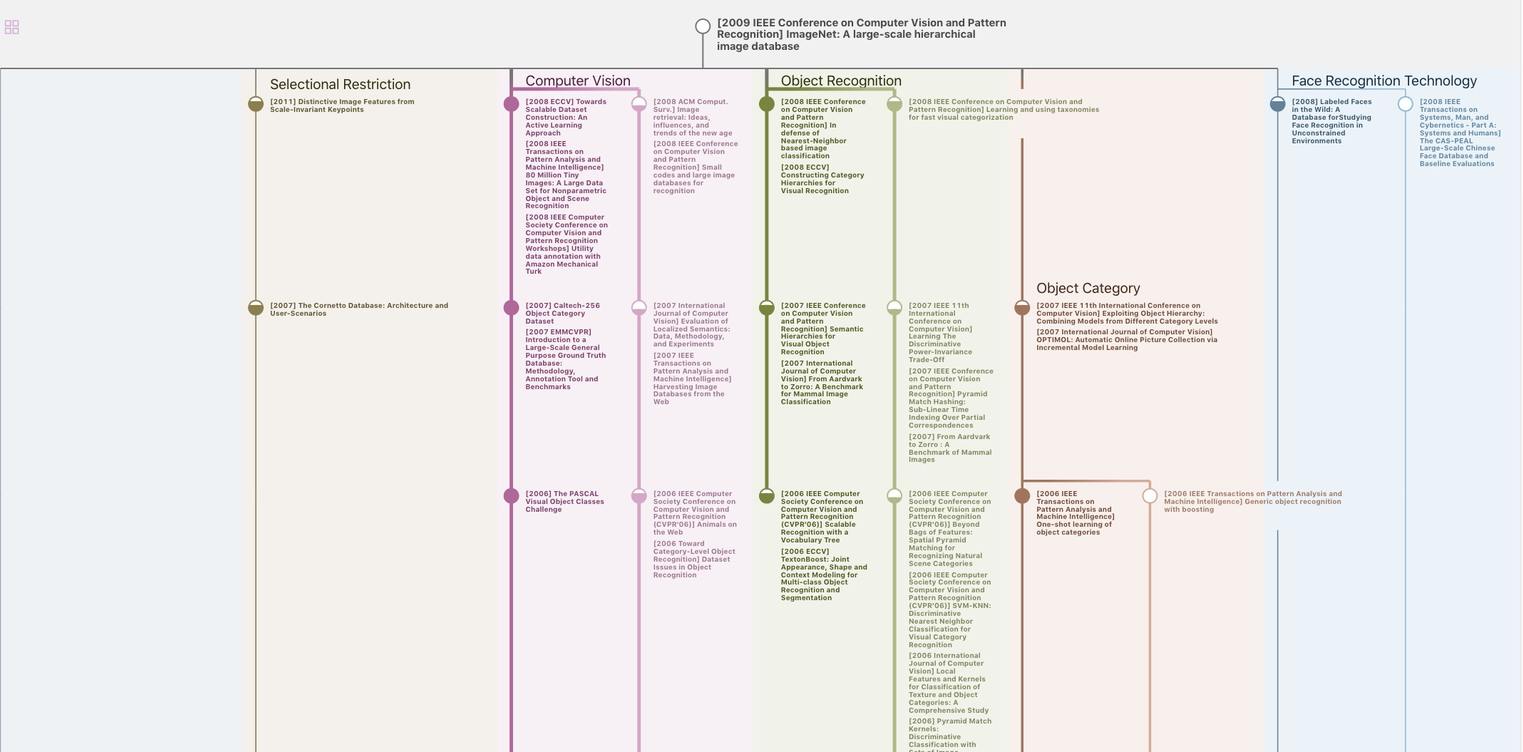
生成溯源树,研究论文发展脉络
Chat Paper
正在生成论文摘要