VDPC: Variational density peak clustering algorithm
Information Sciences(2023)
摘要
The widely applied density peak clustering (DPC) algorithm makes an intuitive cluster for-mation assumption that cluster centers are often surrounded by data points with lower local density and far away from other data points with higher local density. However, this assumption suffers from one limitation that it is often problematic when identifying clus-ters with lower density because they might be easily merged into other clusters with higher density. As a result, DPC may not be able to identify clusters with variational den-sity. To address this issue, we propose a variational density peak clustering (VDPC) algo-rithm, which is designed to systematically and autonomously perform the clustering task on datasets with various types of density distributions. Specifically, we first propose a novel method to identify the representatives among all data points and construct initial clusters based on the identified representatives for further analysis of the clusters' prop-erty. Furthermore, we divide all data points into different levels according to their local density and propose a unified clustering framework by combining the advantages of both DPC and DBSCAN. Thus, all the identified initial clusters spreading across different density levels are systematically processed to form the final clusters. To evaluate the effectiveness of the proposed VDPC algorithm, we conduct extensive experiments using 20 datasets including eight synthetic, six real-world, and six image datasets. The experimental results show that VDPC outperforms two classical algorithms (i.e., DPC and DBSCAN) and four state-of-the-art extended DPC algorithms.(c) 2022 Elsevier Inc. All rights reserved.
更多查看译文
关键词
Density peak clustering,Representatives,Local density analysis
AI 理解论文
溯源树
样例
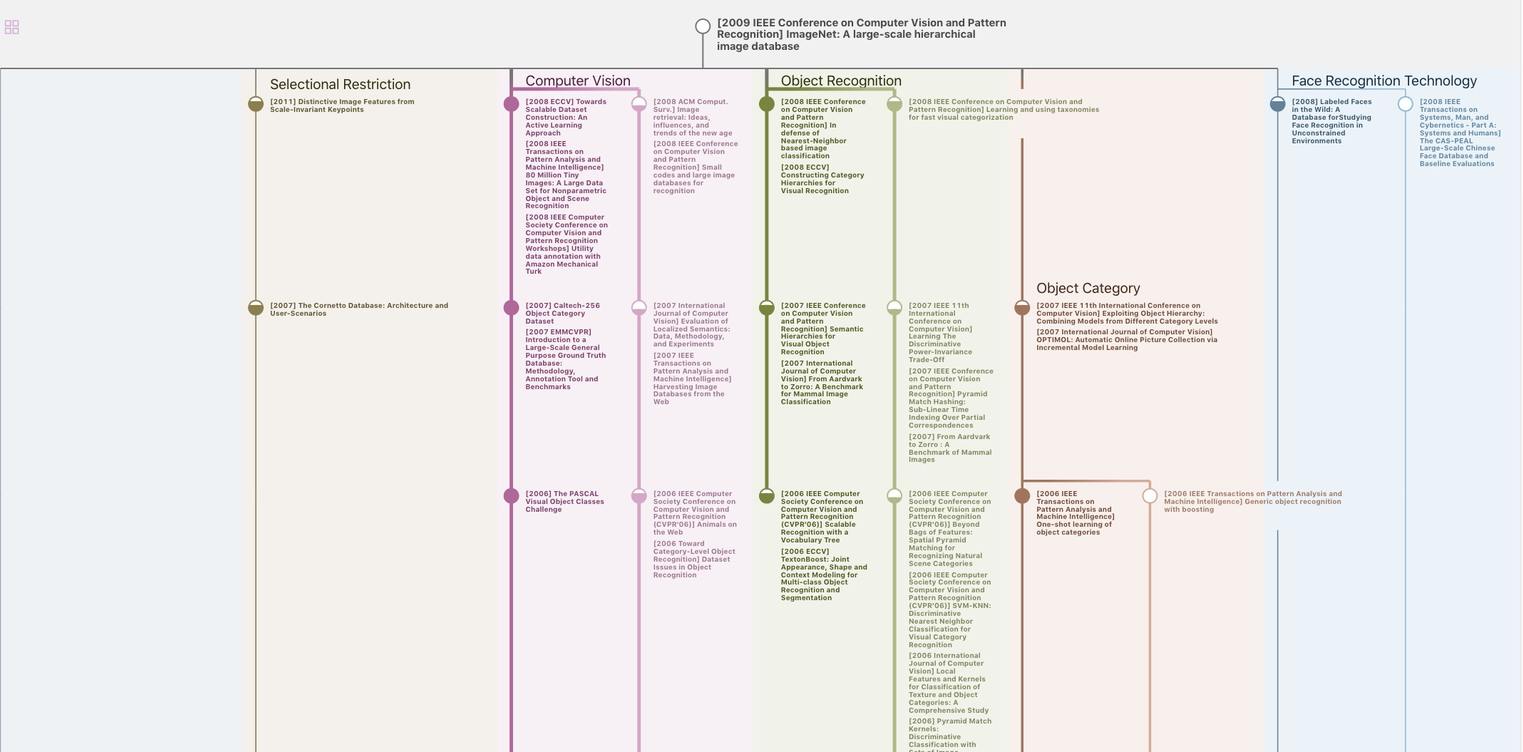
生成溯源树,研究论文发展脉络
Chat Paper
正在生成论文摘要