Feature matching as improved transfer learning technique for wearable EEG
arxiv(2021)
摘要
Objective: With the rapid rise of wearable sleep monitoring devices with non-conventional electrode configurations, there is a need for automated algorithms that can perform sleep staging on configurations with small amounts of labeled data. Transfer learning has the ability to adapt neural network weights from a source modality (e.g. standard electrode configuration) to a new target modality (e.g. non-conventional electrode configuration). Methods: We propose feature matching, a new transfer learning strategy as an alternative to the commonly used finetuning approach. This method consists of training a model with larger amounts of data from the source modality and few paired samples of source and target modality. For those paired samples, the model extracts features of the target modality, matching these to the features from the corresponding samples of the source modality. Results: We compare feature matching to finetuning for three different target domains, with two different neural network architectures, and with varying amounts of training data. Particularly on small cohorts (i.e. 2 - 5 labeled recordings in the non-conventional recording setting), feature matching systematically outperforms finetuning with mean relative differences in accuracy ranging from 0.4% to 4.7% for the different scenarios and datasets. Conclusion: Our findings suggest that feature matching outperforms finetuning as a transfer learning approach, especially in very low data regimes. Significance: As such, we conclude that feature matching is a promising new method for wearable sleep staging with novel devices.
更多查看译文
关键词
Automatic sleep staging,Deep learning,Electroencephalography,Neural network,Transfer learning
AI 理解论文
溯源树
样例
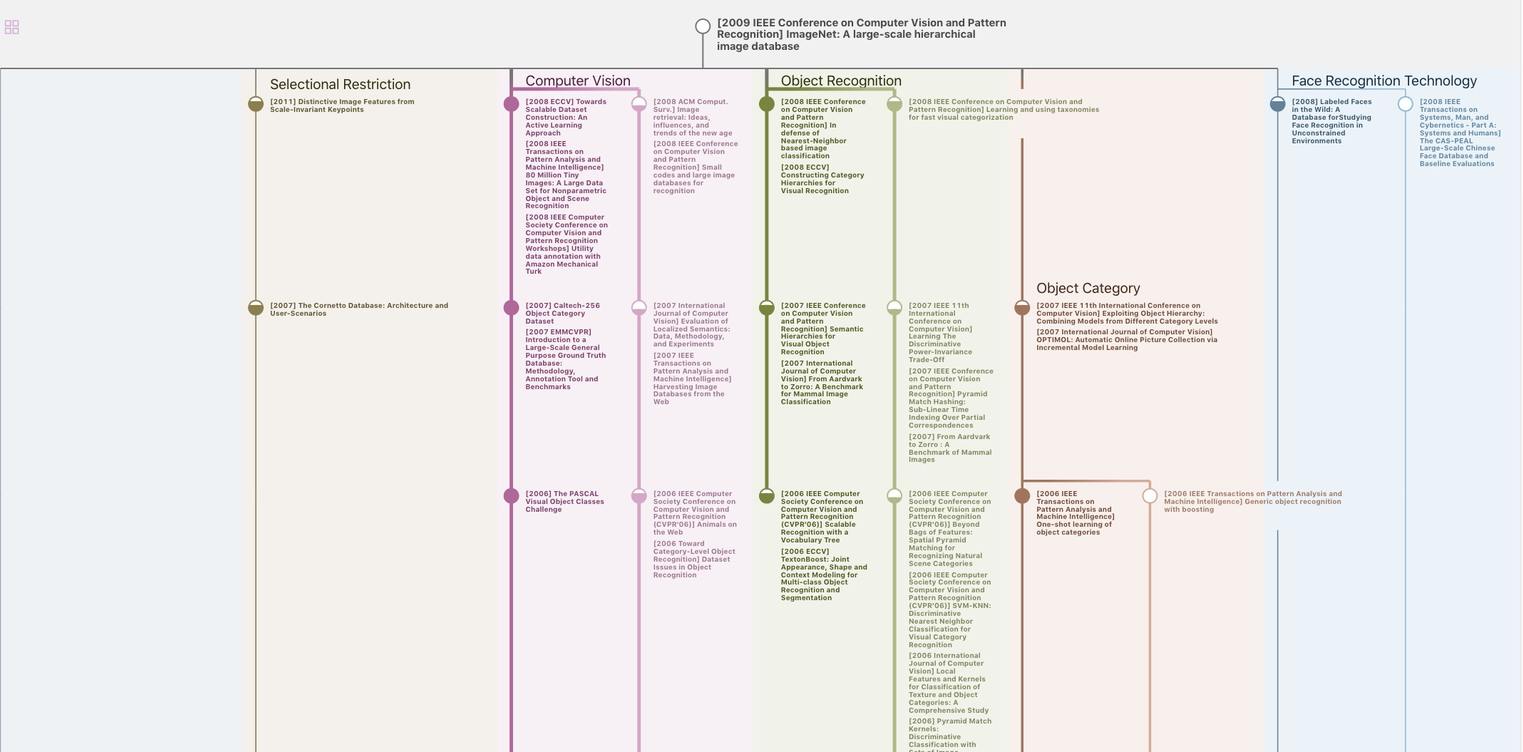
生成溯源树,研究论文发展脉络
Chat Paper
正在生成论文摘要