Optimizing Machine Learning Inference Queries with Correlative Proxy Models
International Conference on Very Large Data Bases(2022)
摘要
We consider accelerating machine learning (ML) inference queries on unstructured datasets. Expensive operators such as feature extractors and classifiers are deployed as user-defined functions(UDFs), which are not penetrable with classic query optimization techniques such as predicate push-down. Recent optimization schemes (e.g., Probabilistic Predicates or PP) assume independence among the query predicates, build a proxy model for each predicate offline, and rewrite a new query by injecting these cheap proxy models in the front of the expensive ML UDFs. In such a manner, unlikely inputs that do not satisfy query predicates are filtered early to bypass the ML UDFs. We show that enforcing the independence assumption in this context may result in sub-optimal plans. In this paper, we propose CORE, a query optimizer that better exploits the predicate correlations and accelerates ML inference queries. Our solution builds the proxy models online for a new query and leverages a branch-and-bound search process to reduce the building costs. Results on three real-world text, image and video datasets show that CORE improves the query throughput by up to 63% compared to PP and up to 80% compared to running the queries as it is.
更多查看译文
关键词
machine learning inference queries,correlative proxy
AI 理解论文
溯源树
样例
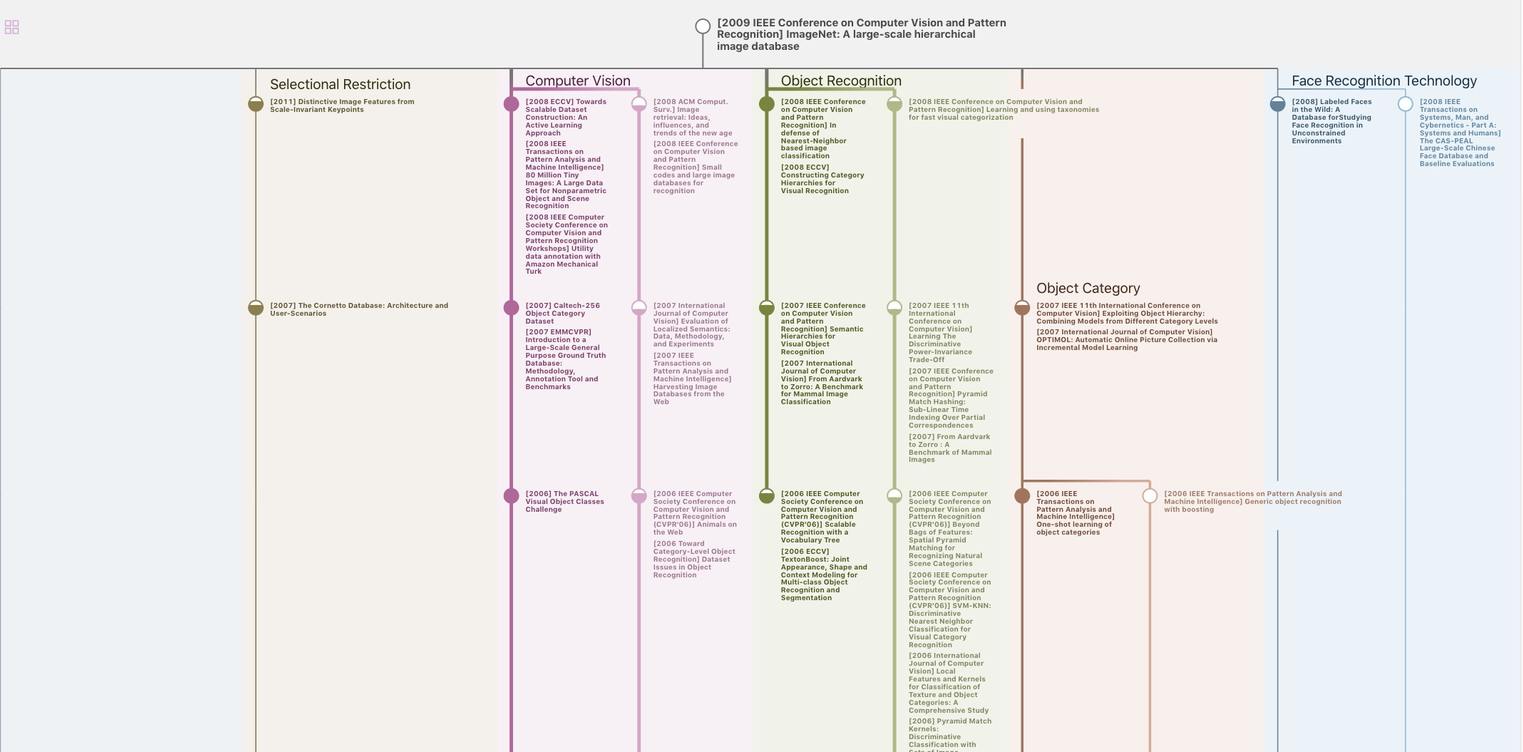
生成溯源树,研究论文发展脉络
Chat Paper
正在生成论文摘要