Generating Adversarial Samples For Training Wake-up Word Detection Systems Against Confusing Words
arxiv(2022)
摘要
Wake-up word detection models are widely used in real life, but suffer from severe performance degradation when encountering adversarial samples. In this paper we discuss the concept of confusing words in adversarial samples. Confusing words are commonly encountered, which are various kinds of words that sound similar to the predefined keywords. To enhance the wake word detection system's robustness against confusing words, we propose several methods to generate the adversarial confusing samples for simulating real confusing words scenarios in which we usually do not have any real confusing samples in the training set. The generated samples include concatenated audio, synthesized data, and partially masked keywords. Moreover, we use a domain embedding concatenated system to improve the performance. Experimental results show that the adversarial samples generated in our approach help improve the system's robustness in both the common scenario and the confusing words scenario. In addition, we release the confusing words testing database called HI-MIA-CW for future research.
更多查看译文
关键词
word detection
AI 理解论文
溯源树
样例
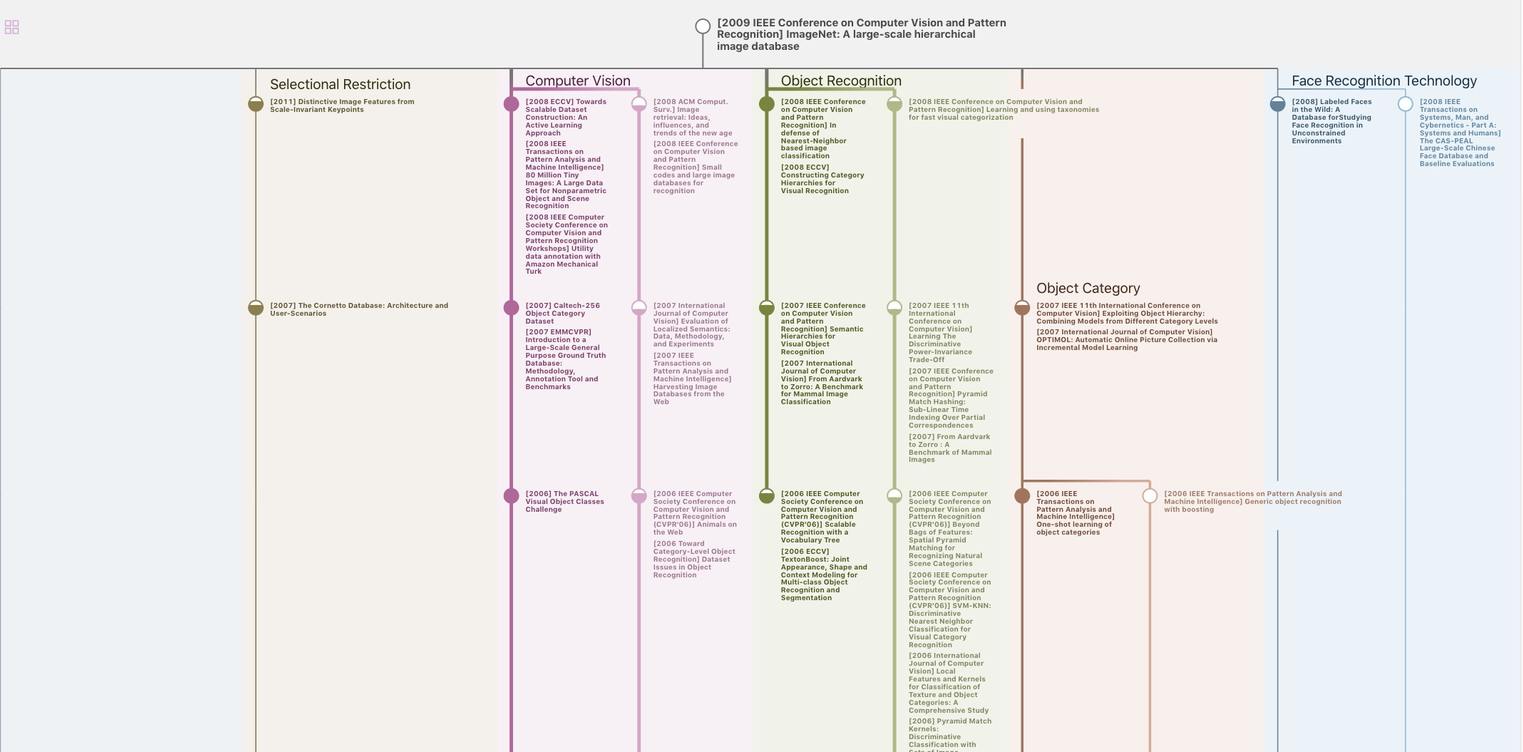
生成溯源树,研究论文发展脉络
Chat Paper
正在生成论文摘要