Stealth Data Injection Attacks with Sparsity Constraints
arxiv(2022)
摘要
Sparse stealth attack constructions that minimize the mutual information between the state variables and the observations are proposed. The attack construction is formulated as the design of a multivariate Gaussian distribution that aims to minimize the mutual information while limiting the Kullback-Leibler divergence between the distribution of the observations under attack and the distribution of the observations without attack. The sparsity constraint is incorporated as a support constraint of the attack distribution. Two heuristic greedy algorithms for the attack construction are proposed. The first algorithm assumes that the attack vector consists of independent entries, and therefore, requires no communication between different attacked locations. The second algorithm considers correlation between the attack vector entries which results in better attack performance at the expense of coordination between different locations. We numerically evaluate the performance of the proposed attack constructions on IEEE test systems and show that it is feasible to construct stealth attacks that generate significant disruption with a low number of compromised sensors.
更多查看译文
关键词
attacks,injection
AI 理解论文
溯源树
样例
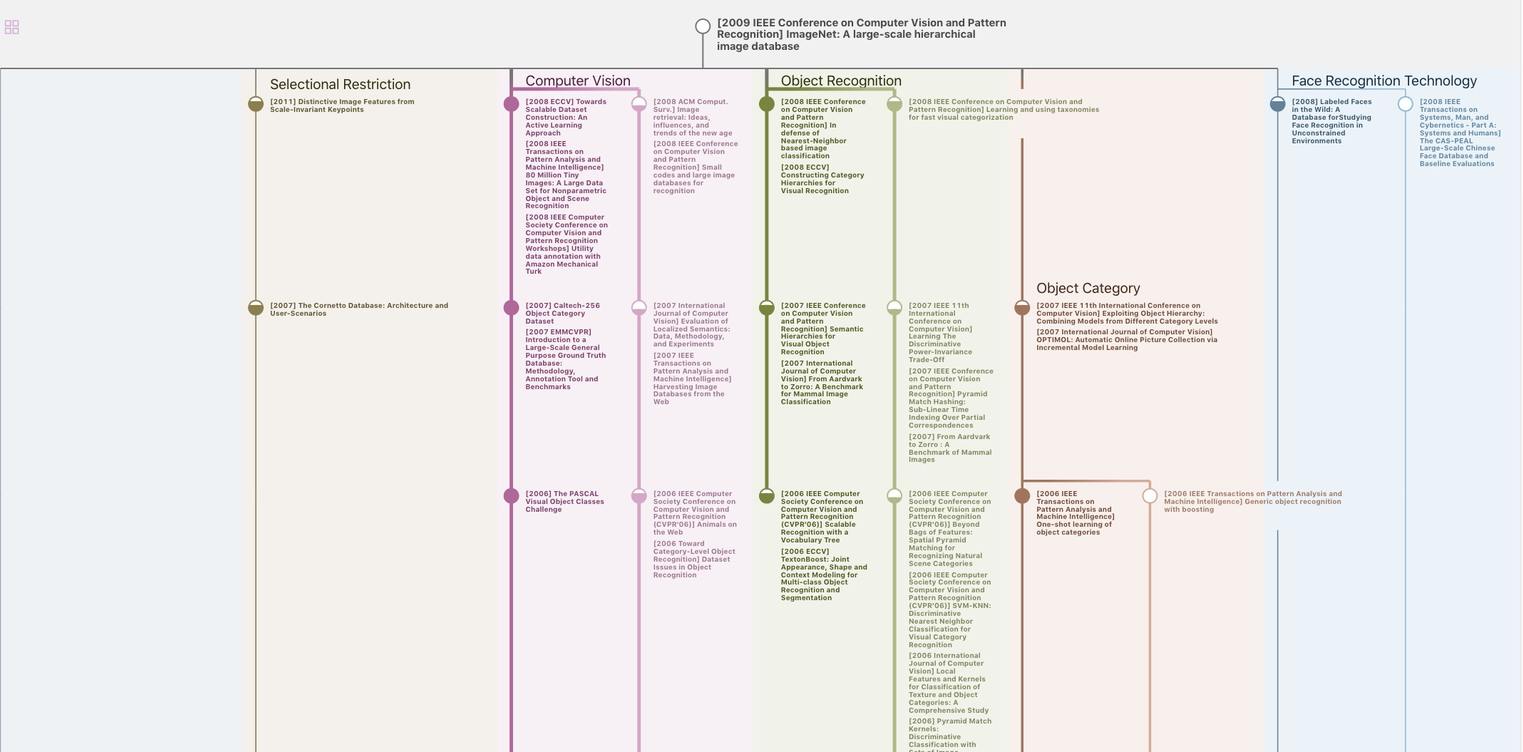
生成溯源树,研究论文发展脉络
Chat Paper
正在生成论文摘要