Learned Coarse Models for Efficient Turbulence Simulation
arxiv(2021)
摘要
Turbulence simulation with classical numerical solvers requires very high-resolution grids to accurately resolve dynamics. Here we train learned simulators at low spatial and temporal resolutions to capture turbulent dynamics generated at high resolution. We show that our proposed model can simulate turbulent dynamics more accurately than classical numerical solvers at the same low resolutions across various scientifically relevant metrics. Our model is trained end-to-end from data and is capable of learning a range of challenging chaotic and turbulent dynamics at low resolution, including trajectories generated by the state-of-the-art Athena++ engine. We show that our simpler, general-purpose architecture outperforms various more specialized, turbulence-specific architectures from the learned turbulence simulation literature. In general, we see that learned simulators yield unstable trajectories; however, we show that tuning training noise and temporal downsampling solves this problem. We also find that while generalization beyond the training distribution is a challenge for learned models, training noise, convolutional architectures, and added loss constraints can help. Broadly, we conclude that our learned simulator outperforms traditional solvers run on coarser grids, and emphasize that simple design choices can offer stability and robust generalization.
更多查看译文
关键词
coarse models,simulation
AI 理解论文
溯源树
样例
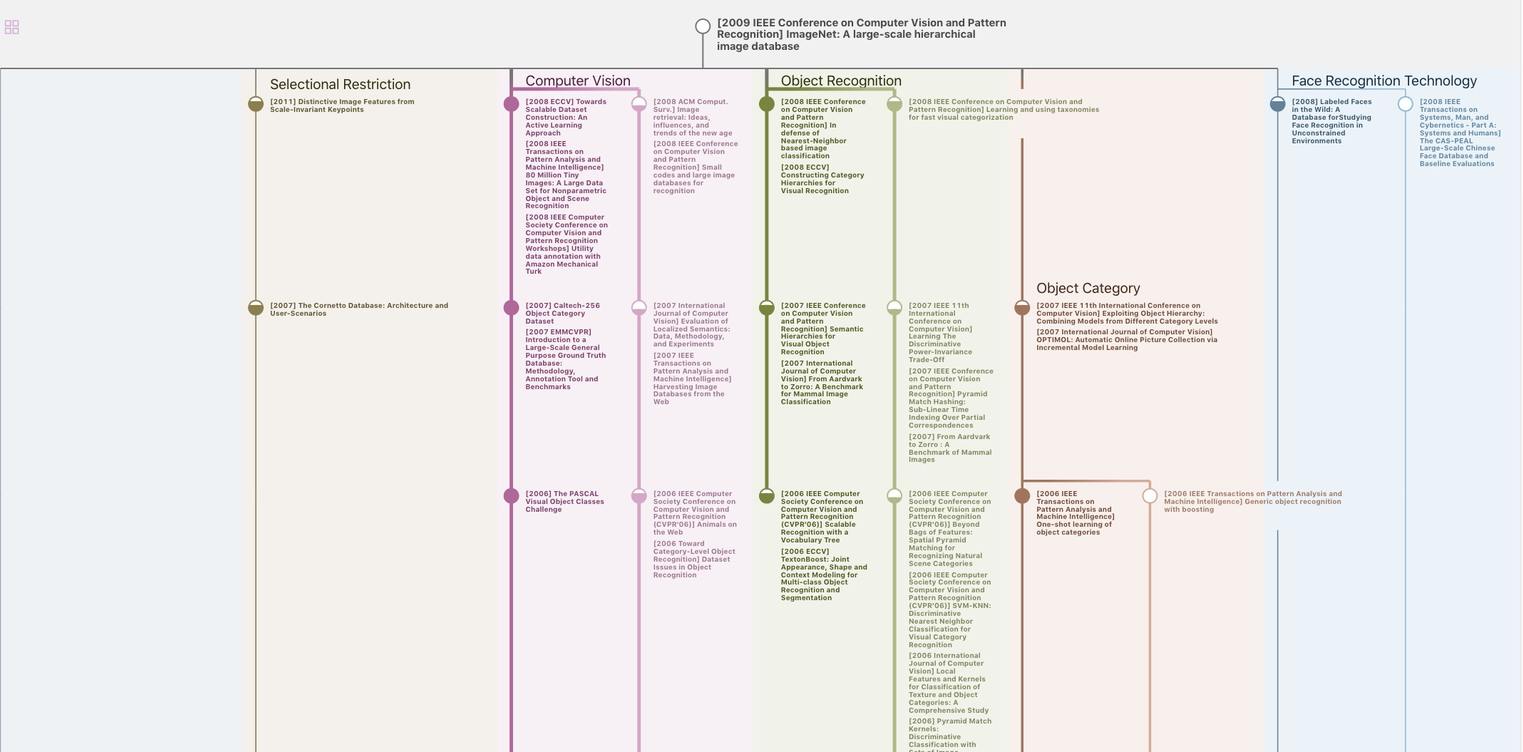
生成溯源树,研究论文发展脉络
Chat Paper
正在生成论文摘要