Interpretable network propagation with application to expanding the repertoire of human proteins that interact with SARS-CoV-2
GIGASCIENCE(2021)
摘要
Background: Network propagation has been widely used for nearly 20 years to predict gene functions and phenotypes. Despite the popularity of this approach, little attention has been paid to the question of provenance tracing in this context, e.g., determining how much any experimental observation in the input contributes to the score of every prediction. Results: We design a network propagation framework with 2 novel components and apply it to predict human proteins that directly or indirectly interact with SARS-CoV-2 proteins. First, we trace the provenance of each prediction to its experimentally validated sources, which in our case are human proteins experimentally determined to interact with viral proteins. Second, we design a technique that helps to reduce the manual adjustment of parameters by users. We find that for every top-ranking prediction, the highest contribution to its score arises from a direct neighbor in a human protein-protein interaction network. We further analyze these results to develop functional insights on SARS-CoV-2 that expand on known biology such as the connection between endoplasmic reticulum stress, HSPA5, and anti-clotting agents. Conclusions: We examine how our provenance-tracing method can be generalized to a broad class of network-based algorithms. We provide a useful resource for the SARS-CoV-2 community that implicates many previously undocumented proteins with putative functional relationships to viral infection. This resource includes potential drugs that can be opportunistically repositioned to target these proteins. We also discuss how our overall framework can be extended to other, newly emerging viruses.
更多查看译文
关键词
network propagation, interpretable machine learning, provenance tracing, SARS-CoV-2, COVID-19, virus-host protein interaction networks
AI 理解论文
溯源树
样例
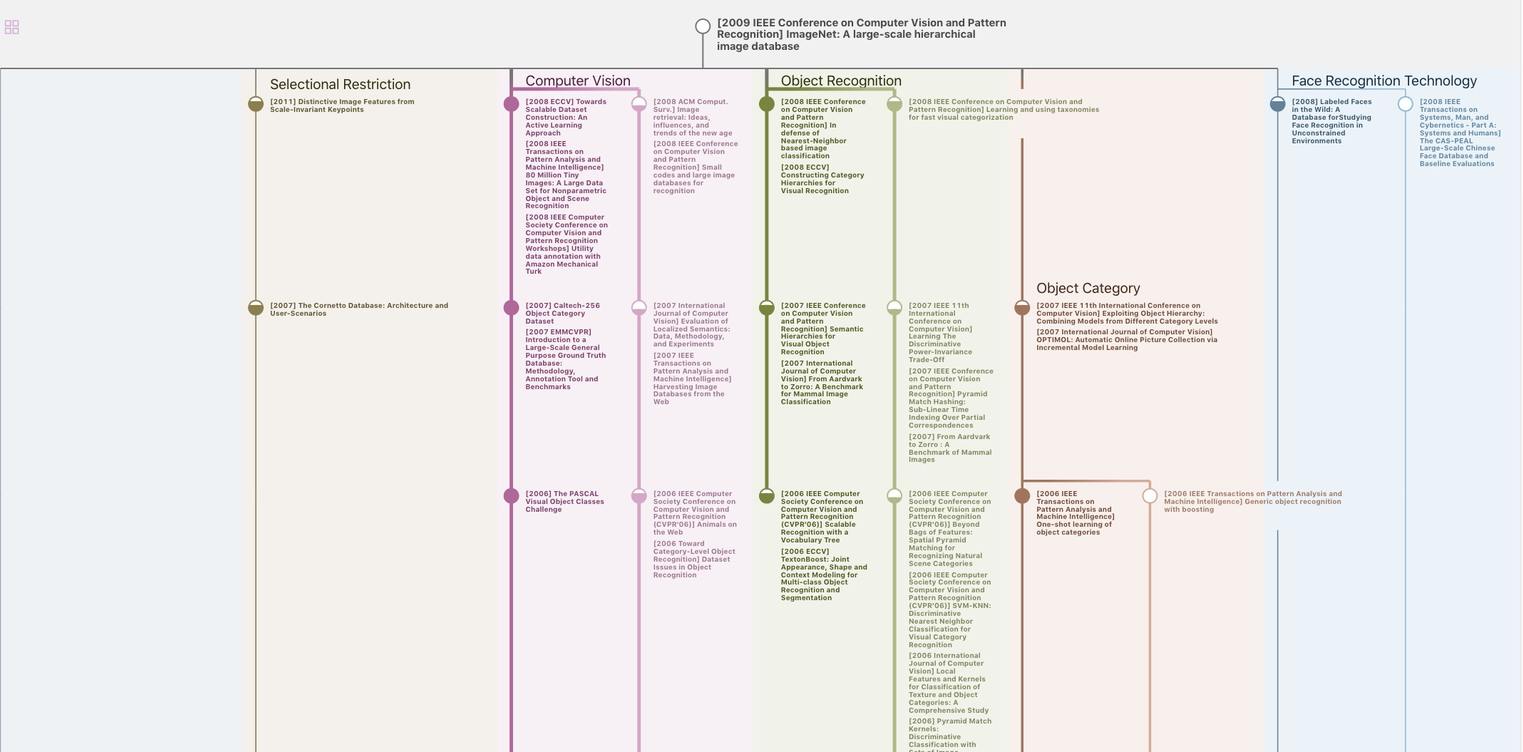
生成溯源树,研究论文发展脉络
Chat Paper
正在生成论文摘要