Bayesian neural hawkes process for event uncertainty prediction
arxiv(2023)
摘要
Event data consisting of time of occurrence of the events arises in several real-world applications. A commonly used framework to model such events is known as temporal point process , which is a stochastic process that predicts when the event will occur given the sequence of past events. Recent works have introduced neural network based point processes which outperformed statistical models such as Hawkes process and were shown to provide state-of-the-art performance in predicting event-times. However, neural point process models lack uncertainty quantification capability on predictions. A proper uncertainty quantification over event modeling will help in better decision-making for many practical and critical applications. In this work, we propose a novel and lightweight point process model, Bayesian Neural Hawkes process (BNHP) which leverages uncertainty modeling capability of Bayesian models and generalization capability of the neural networks to model event occurrence times. Additionally, we augment the model with spatio-temporal modeling capability where it can consider uncertainty over predicted time and location of the events. Experiments on several simulated and real-world datasets show that BNHP significantly improves prediction performance and uncertainty quantification for event prediction.
更多查看译文
关键词
bayesian neural hawkes process,event uncertainty prediction
AI 理解论文
溯源树
样例
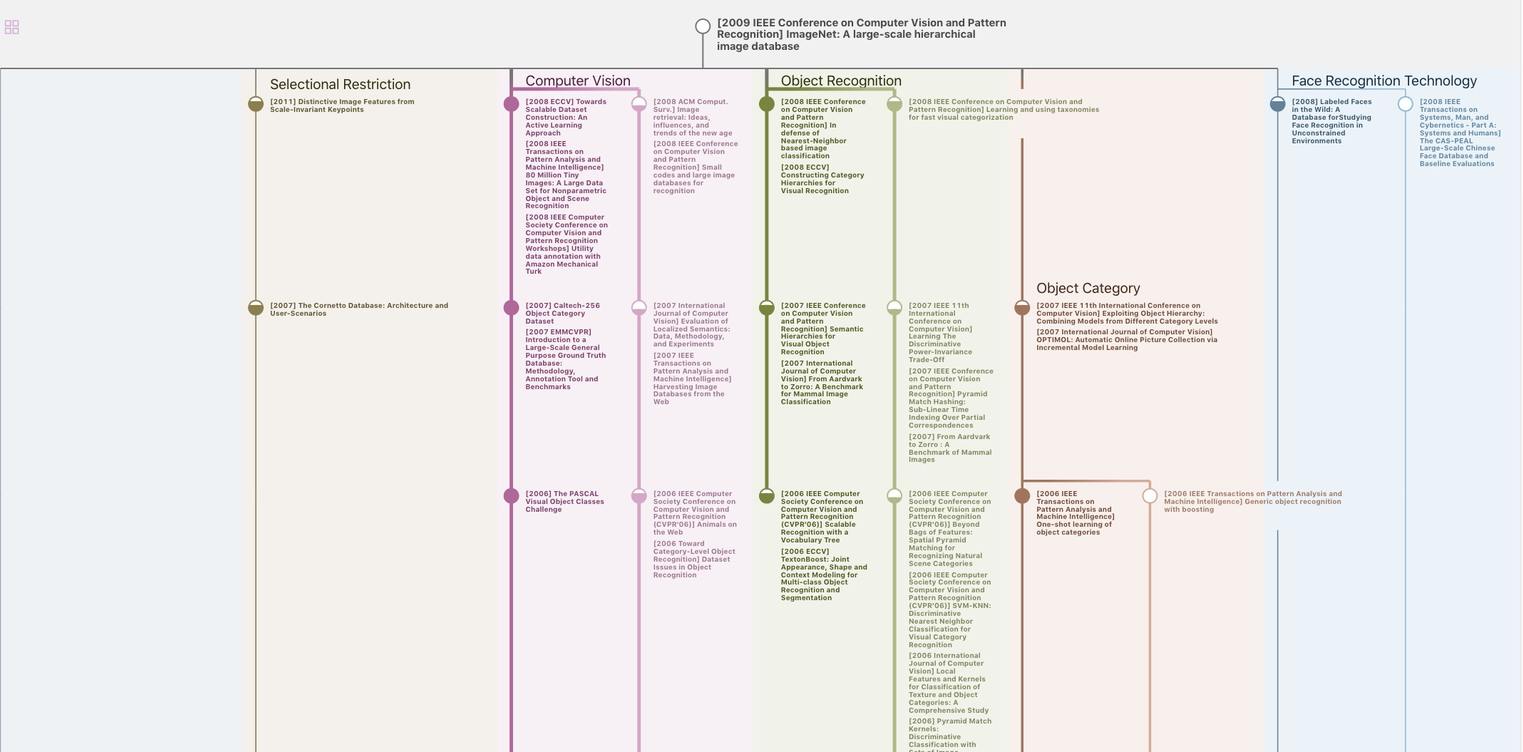
生成溯源树,研究论文发展脉络
Chat Paper
正在生成论文摘要