Metaknowledge Enhanced Open Domain Question Answering with Wiki Documents
SENSORS(2021)
摘要
The commonly-used large-scale knowledge bases have been facing challenges in open domain question answering tasks which are caused by the loose knowledge association and weak structural logic of triplet-based knowledge. To find a way out of this dilemma, this work proposes a novel metaknowledge enhanced approach for open domain question answering. We design an automatic approach to extract metaknowledge and build a metaknowledge network from Wiki documents. For the purpose of representing the directional weighted graph with hierarchical and semantic features, we present an original graph encoder GE4MK to model the metaknowledge network. Then, a metaknowledge enhanced graph reasoning model MEGr-Net is proposed for question answering, which aggregates both relational and neighboring interactions comparing with R-GCN and GAT. Experiments have proved the improvement of metaknowledge over main-stream triplet-based knowledge. We have found that the graph reasoning models and pre-trained language models also have influences on the metaknowledge enhanced question answering approaches.
更多查看译文
关键词
metaknowledge, graph modeling, question answering, graph neural networks, knowledge graph
AI 理解论文
溯源树
样例
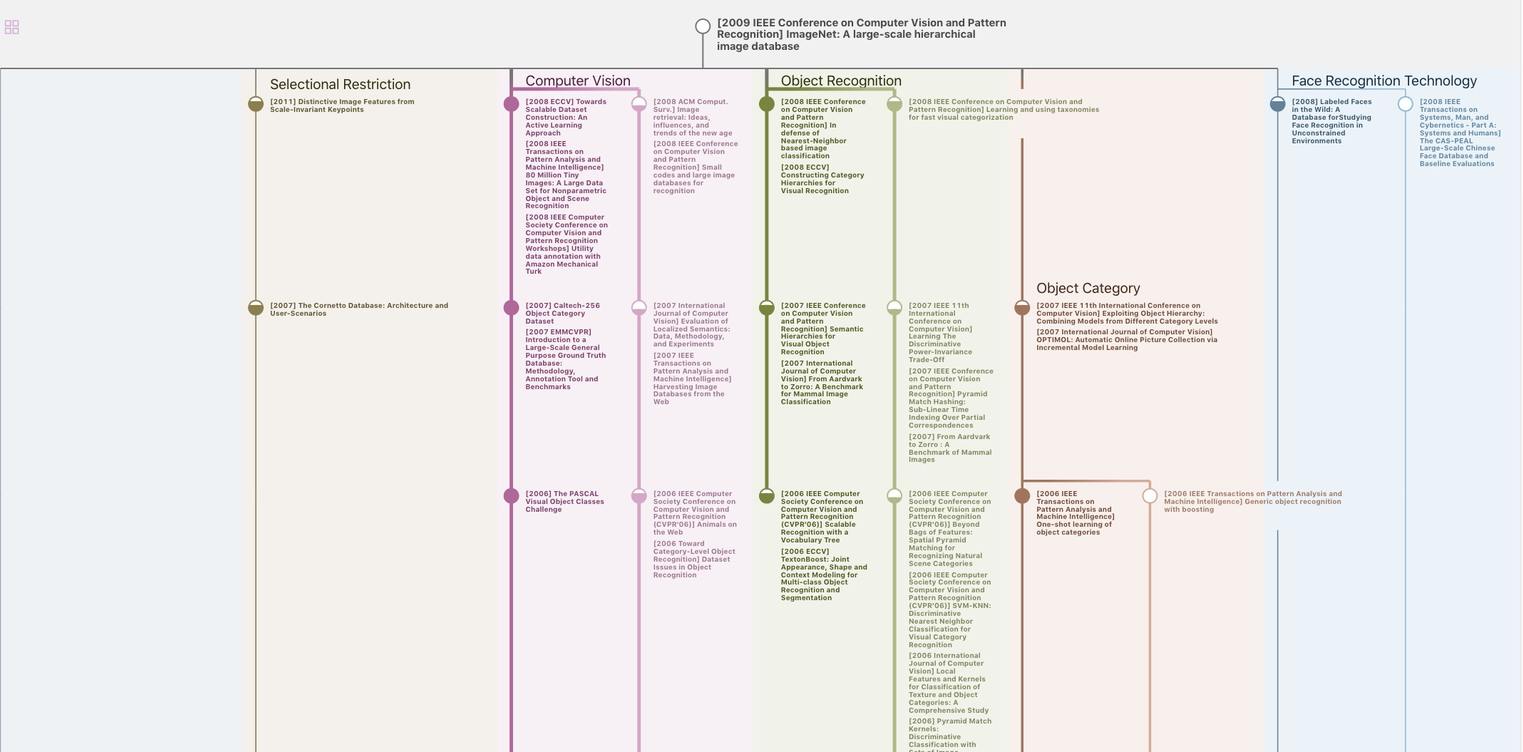
生成溯源树,研究论文发展脉络
Chat Paper
正在生成论文摘要