Hyperspectral Estimation of Winter Wheat Leaf Area Index Based on Continuous Wavelet Transform and Fractional Order Differentiation
SENSORS(2021)
摘要
Leaf area index (LAI) is highly related to crop growth, and the traditional LAI measurement methods are field destructive and unable to be acquired by large-scale, continuous, and real-time means. In this study, fractional order differential and continuous wavelet transform were used to process the canopy hyperspectral reflectance data of winter wheat, the fractional order differential spectral bands and wavelet energy coefficients with more sensitive to LAI changes were screened by correlation analysis, and the optimal subset regression and support vector machine were used to construct the LAI estimation models for different growth stages. The precision evaluation results showed that the LAI estimation models constructed by using wavelet energy coefficients combined with a support vector machine at the jointing stage, fractional order differential combined with support vector machine at the booting stage, and wavelet energy coefficients combined with optimal subset regression at the flowering and filling stages had the best prediction performance. Among these, both flowering and filling stages could be used as the best growth stages for LAI estimation with modeling and validation R-2 of 0.87 and 0.71, 0.84 and 0.77, respectively. This study can provide technical reference for LAI estimation of crops based on remote sensing technology.
更多查看译文
关键词
winter wheat, leaf area index, fractional order differential, continuous wavelet transform, optimal subset regression, support vector machine
AI 理解论文
溯源树
样例
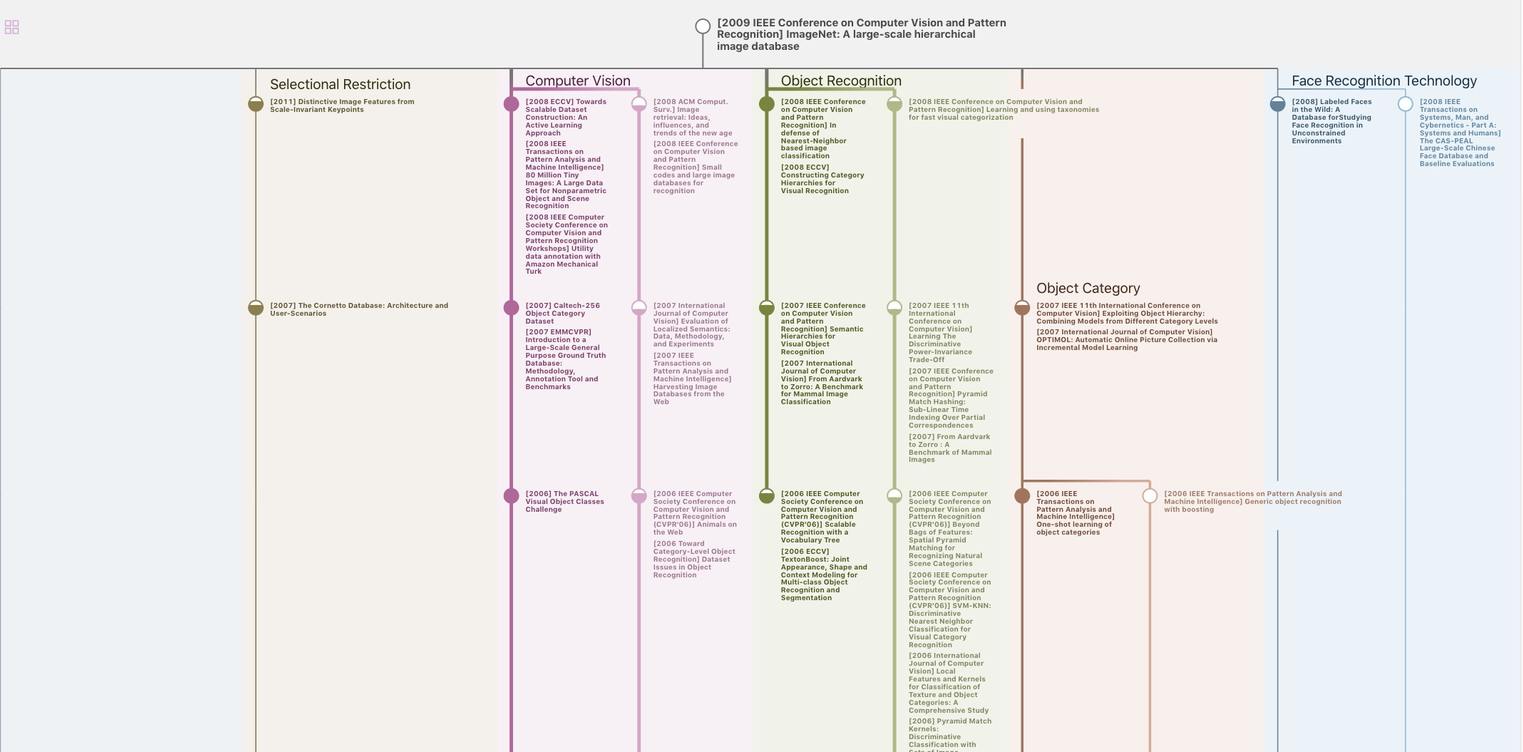
生成溯源树,研究论文发展脉络
Chat Paper
正在生成论文摘要