Towards Arabic aspect-based sentiment analysis: a transfer learning-based approach
SOCIAL NETWORK ANALYSIS AND MINING(2021)
摘要
Aspect-based sentiment analysis (ABSA) is a fine-grained sentiment analysis task that aims to extract the discussed aspects and identify their corresponding sentiment polarities from a given text. Most of the existing Arabic ABSA methods rely heavily on tedious preprocessing and feature-engineering tasks in addition to the use of external resources (e.g., lexicons). Hence, this paper tries to overcome these shortcomings by proposing a transfer learning method using pre-trained language models to perform two ABSA tasks in Arabic, namely aspect term extraction (ATE) and aspect category detection (ACD). The proposed models are built based on the Arabic version (AraBERT) of the BERT model. Different implementations of BERT are compared, including fine-tuning and feature-based methods. The main findings of this paper are: (1) Fine-tuning is more suitable in low-resource settings. (2) Designing customized downstream layers enhances the default fine-tuned BERT model results. The experiments were conducted on a reference ABSA dataset on Arabic news posts. The results show that our models outperform the baseline methods and the related work with an overall enhancement of more than 6% for the ATE task and more than 19% for the ACD task.
更多查看译文
关键词
Deep learning, Transfer learning, Natural language processing, Aspect-based sentiment analysis, Arabic language, BERT
AI 理解论文
溯源树
样例
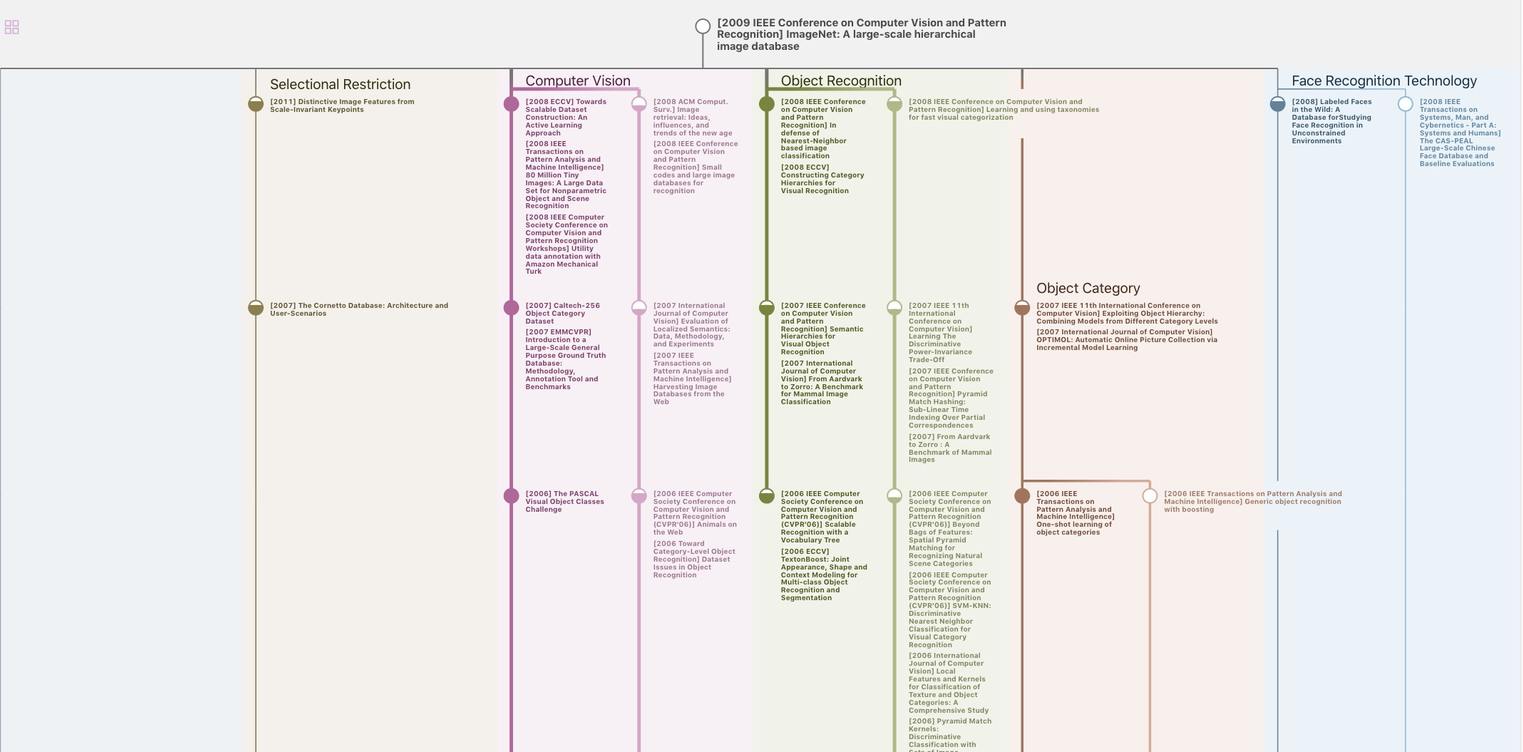
生成溯源树,研究论文发展脉络
Chat Paper
正在生成论文摘要