Bayesian stochastic configuration networks for robust data modeling
CONCURRENCY AND COMPUTATION-PRACTICE & EXPERIENCE(2022)
摘要
The SCN networks is incrementally generated by stochastic configuration (SC) algorithms. It randomly assigns the input weights and deviations of hidden nodes through a supervisory mechanism, which can be trained by solving linear modeling problems. The version that uses least squares to estimate the output weight performs well. This article introduces an alternative strategy for performing complete Bayesian inference (BI) of SCN networks. Different from the traditional way, the Bayesian training algorithm we proposed can obtain an entire probability distribution on the optimal output weight of the SCN networks, instead of a single pointwise estimate. The advantage of the Bayesian inference method lies in the possibility of introducing other prior knowledge during the training process, providing a way to measure uncertainty during the testing phase and the ability to automatically infer hyper-parameters from given data. The BI algorithm proposed in this article for regression problem can be implemented through an iterative process under some practical assumptions. Experimental results show that our proposed Bayesian SCN algorithm performs well in solving data modeling problems with a large number of outliers.
更多查看译文
关键词
Bayesian, robust, stochastic configuration networks
AI 理解论文
溯源树
样例
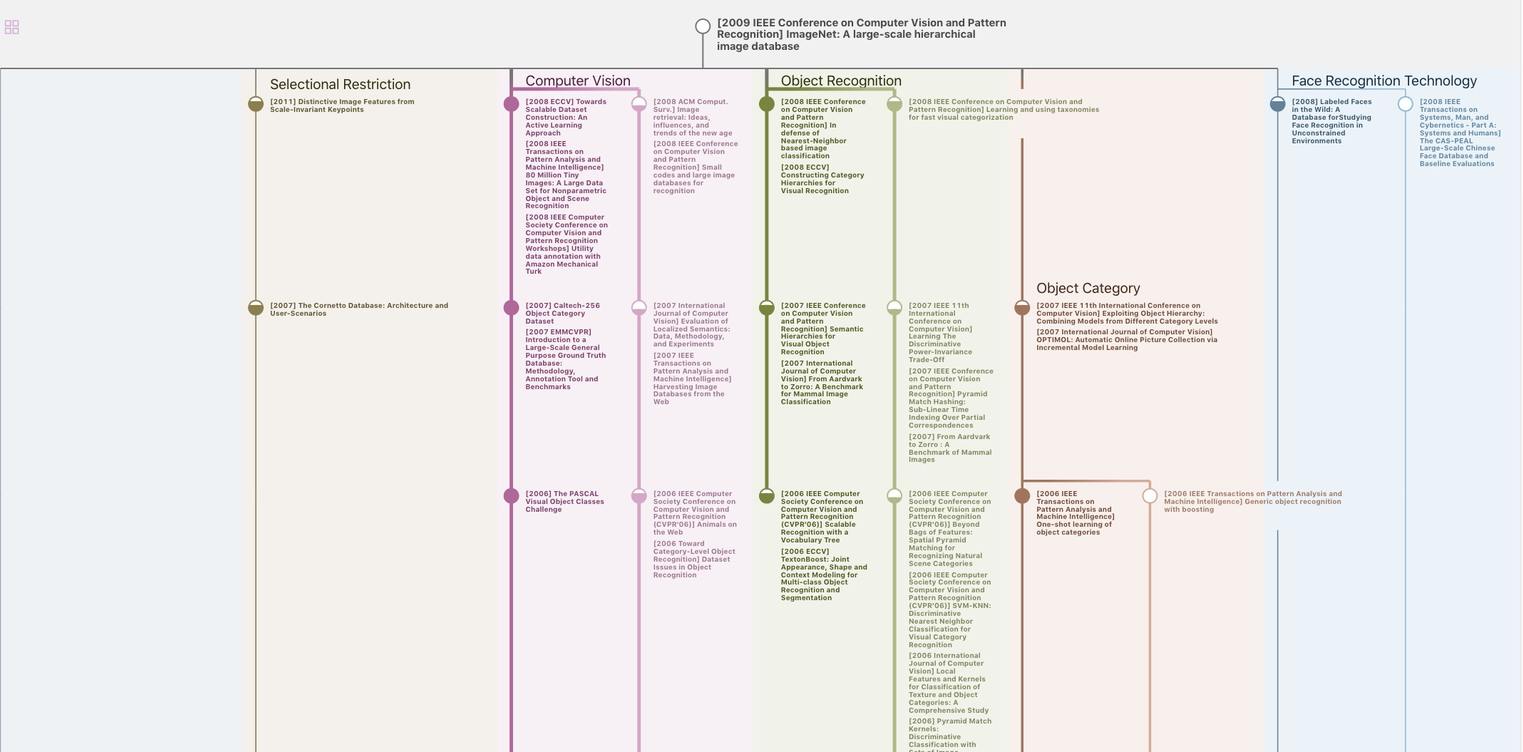
生成溯源树,研究论文发展脉络
Chat Paper
正在生成论文摘要