Self-Supervised Contrastive Learning for Volcanic Unrest Detection
IEEE GEOSCIENCE AND REMOTE SENSING LETTERS(2022)
摘要
Ground deformation measured from interferometric synthetic aperture radar (InSAR) data is considered a sign of volcanic unrest, statistically linked to a volcanic eruption. Recent studies have shown the potential of using Sentinel-1 InSAR data and supervised deep learning (DL) methods for the detection of volcanic deformation signals, toward global volcanic hazard mitigation. However, detection accuracy is compromised from the lack of labeled data and class imbalance. To overcome this, synthetic data are typically used for fine-tuning DL models pretrained on the ImageNet dataset. This approach suffers from poor generalization on real InSAR data. This letter proposes the use of self-supervised contrastive learning to learn quality visual representations hidden in unlabeled InSAR data. Our approach, based on the SimCLR framework, provides a solution that does not require a specialized architecture nor a large labeled or synthetic dataset. We show that our self-supervised pipeline achieves higher accuracy with respect to the state-of-the-art methods and shows excellent generalization even for out-of-distribution test data. Finally, we showcase the effectiveness of our approach for detecting the unrest episodes preceding the recent Icelandic Fagradalsfjall volcanic eruption.
更多查看译文
关键词
Strain, Task analysis, Training, Pipelines, Observatories, Convolutional neural networks, Volcanic activity, Contrastive learning, deep learning (DL), Fagradalsfjall eruption, interferometric synthetic aperture radar~(InSAR), self-supervised, SimCLR, volcano
AI 理解论文
溯源树
样例
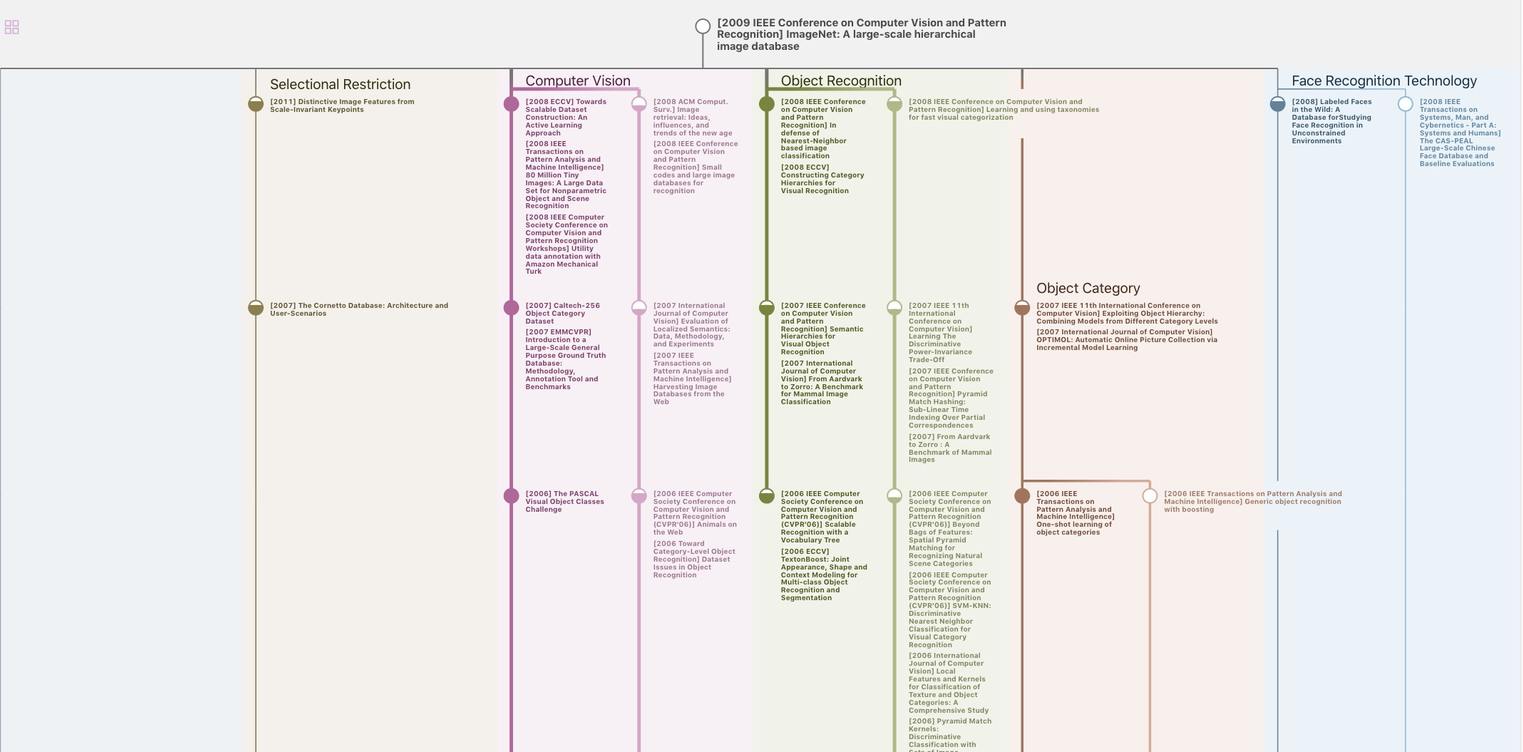
生成溯源树,研究论文发展脉络
Chat Paper
正在生成论文摘要