Assessment of Drug Proarrhythmicity Using Artificial Neural Networks With in silico Deterministic Model Outputs
FRONTIERS IN PHYSIOLOGY(2021)
摘要
As part of the Comprehensive in vitro Proarrhythmia Assay initiative, methodologies for predicting the occurrence of drug-induced torsade de pointes via computer simulations have been developed and verified recently. However, their predictive performance still requires improvement. Herein, we propose an artificial neural networks (ANN) model that uses nine multiple input features, considering the action potential morphology, calcium transient morphology, and charge features to further improve the performance of drug toxicity evaluation. The voltage clamp experimental data for 28 drugs were augmented to 2,000 data entries using an uncertainty quantification technique. By applying these data to the modified O'Hara Rudy in silico model, nine features (dVm/dt(max), AP(resting), APD90, APD50, Ca-resting, CaD90, CaD50, qNet, and qInward) were calculated. These nine features were used as inputs to an ANN model to classify drug toxicity into high-risk, intermediate-risk, and low-risk groups. The model was trained with data from 12 drugs and tested using the data of the remaining 16 drugs. The proposed ANN model demonstrated an AUC of 0.92 in the high-risk group, 0.83 in the intermediate-risk group, and 0.98 in the low-risk group. This was higher than the classification performance of the method proposed in previous studies.
更多查看译文
关键词
proarrhythmicity, toxicology classification, comprehensive in vitro proarrhythmic assay (CiPA), artificial neural network (ANN), in silico
AI 理解论文
溯源树
样例
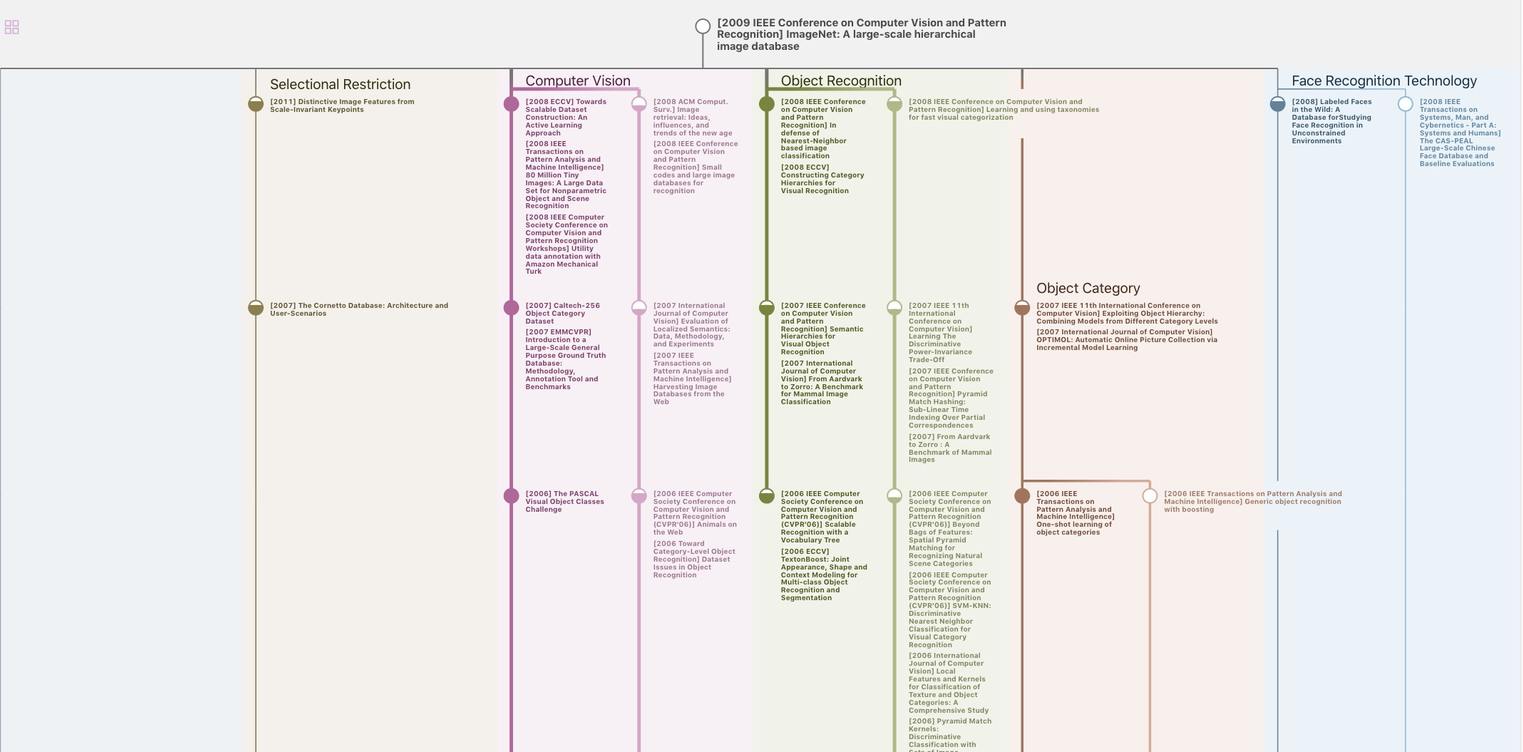
生成溯源树,研究论文发展脉络
Chat Paper
正在生成论文摘要