Transformer Uncertainty Estimation with Hierarchical Stochastic Attention.
AAAI Conference on Artificial Intelligence(2022)
摘要
Transformers are state-of-the-art in a wide range of NLP tasks and have also been applied to many real-world products. Understanding the reliability and certainty of transformer models is crucial for building trustable machine learning applications, e.g., medical diagnosis. Although many recent transformer extensions have been proposed, the study of the uncertainty estimation of transformer models is under-explored. In this work, we propose a novel way to enable transformers to have the capability of uncertainty estimation and, meanwhile, retain the original predictive performance. This is achieved by learning hierarchical stochastic self-attention that attends to values and a set of learnable centroids, respectively. Then new attention heads are formed with a mixture of sampled centroids using the Gumbel-Softmax trick. We theoretically show that the self-attention approximation by sampling from a Gumbel distribution is upper bounded. We empirically evaluate our model on two text classification tasks with both in-domain (ID) and out-of-domain (OOD) datasets. The experimental results demonstrate that our approach: (1) achieves the best predictive-uncertainty trade-off among compared methods; (2) exhibits very competitive (in most cases, better) predictive performance on ID datasets; (3) is on par with Monte Carlo dropout and ensemble methods in uncertainty estimation on OOD datasets.
更多查看译文
关键词
Speech & Natural Language Processing (SNLP)
AI 理解论文
溯源树
样例
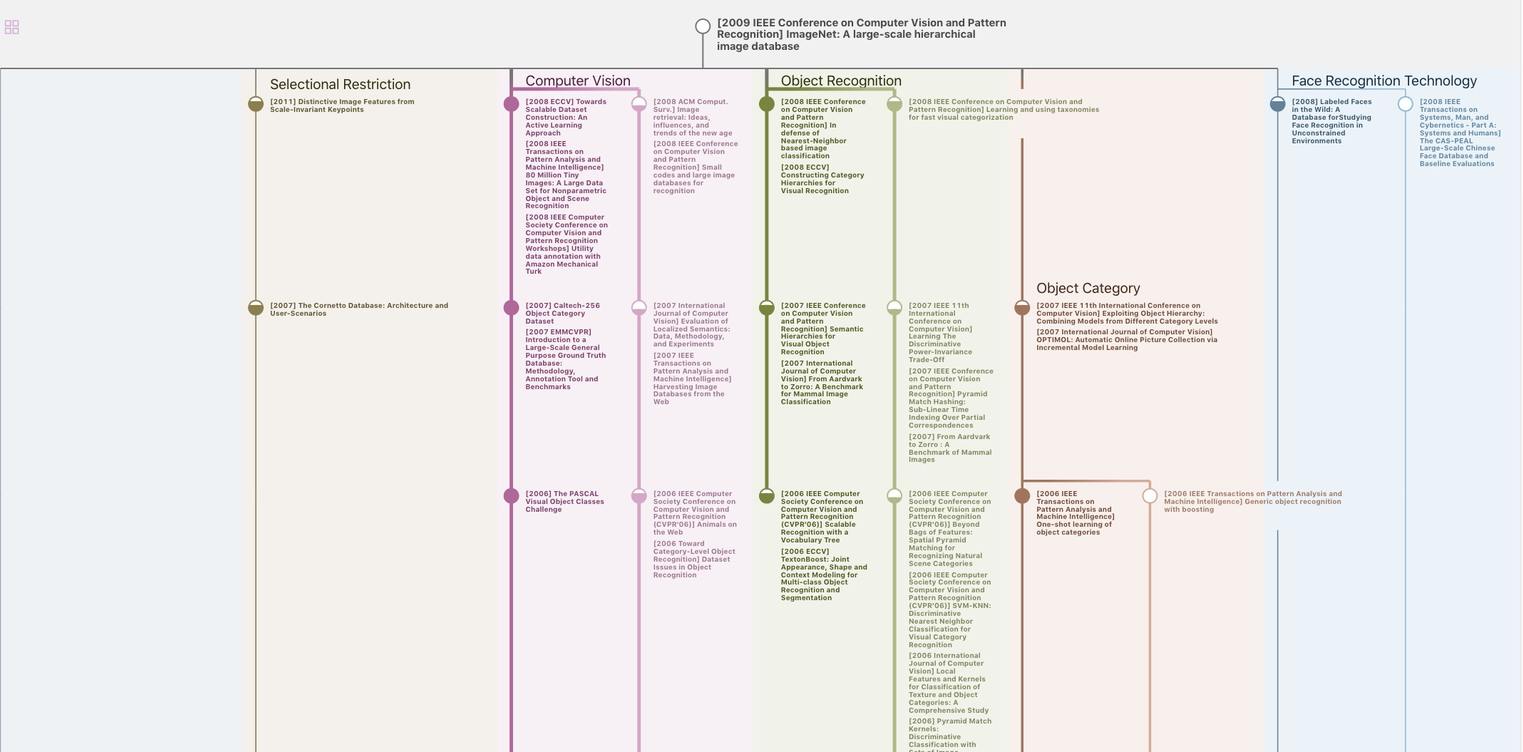
生成溯源树,研究论文发展脉络
Chat Paper
正在生成论文摘要