DTSMLA: A dynamic task scheduling multi-level attention model for stock ranking
EXPERT SYSTEMS WITH APPLICATIONS(2024)
摘要
Predicting stock ranking is a complex and challenging task due to the intricate nature of real stock market systems. There are two main obstacles for current methods to directly using historical trading data to predict stock ranking: (1) Predicting stock rankings is a complex process influenced by a variety of factors, such as the temporal dependence of each stock, the spatial correlation between stocks, and the influence of the market on the stock; (2) The stock market's considerable noise creates volatility in directly predicting stock rankings on a given day, presenting difficulties in training models. To overcome these two challenges, a dynamic task scheduling multi-level attention model (DTSMLA) is proposed to enhance stock ranking prediction through market index and multi-task learning. In response to complexity of stock data, we synthesize the factors affecting stocks and propose a multi-level attention prediction framework. To address the noise issue, we introduce multi-task learning and use a task scheduler algorithm to dynamically select auxiliary tasks for model training. Experimental results conducted on four real-world stock datasets have demonstrated that our method outperforms several state-of-the-art approaches.
更多查看译文
关键词
Stock ranking,Market index,Multi-task learning,Task scheduling
AI 理解论文
溯源树
样例
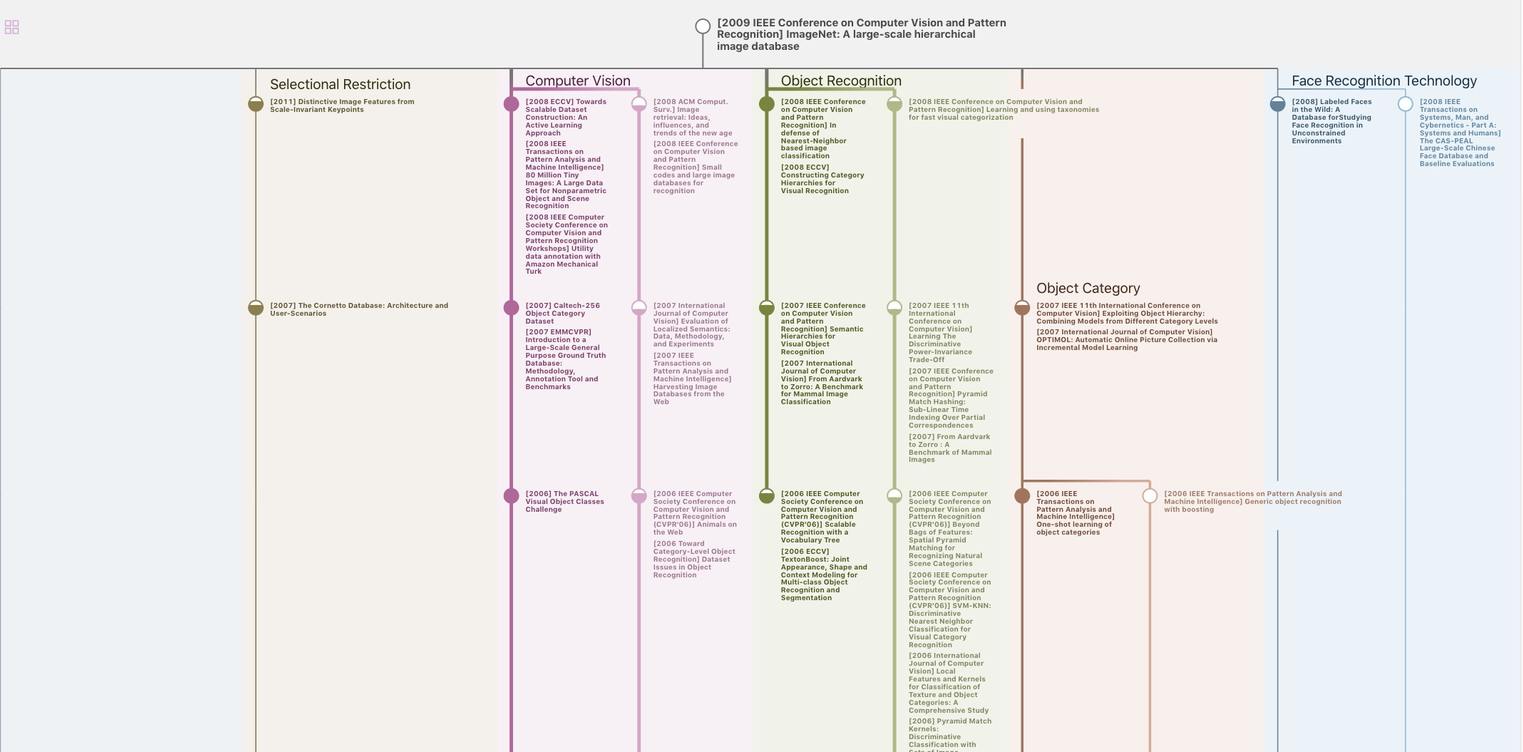
生成溯源树,研究论文发展脉络
Chat Paper
正在生成论文摘要