Rethinking the Data Annotation Process for Multi-view 3D Pose Estimation with Active Learning and Self-Training
arxiv(2023)
摘要
Pose estimation of the human body and hands is a fundamental problem in computer vision, and learning-based solutions require a large amount of annotated data. In this work, we improve the efficiency of the data annotation process for 3D pose estimation problems with Active Learning (AL) in a multi-view setting. AL selects examples with the highest value to annotate under limited annotation budgets (time and cost), but choosing the selection strategy is often nontrivial. We present a framework to efficiently extend existing singleview AL strategies. We then propose two novel AL strategies that make full use of multi-view geometry. Moreover, we demonstrate additional performance gains by incorporating pseudo-labels computed during the AL process, which is a form of self-training. Our system significantly outperforms simulated annotation baselines in 3D body and hand pose estimation on two large-scale benchmarks: CMU Panoptic Studio and InterHand2.6M. Notably, on CMU Panoptic Studio, we are able to reduce the turn-around time by 60% and annotation cost by 80% when compared to the conventional annotation process.
更多查看译文
关键词
active learning,data annotation process,multi-view,self-training
AI 理解论文
溯源树
样例
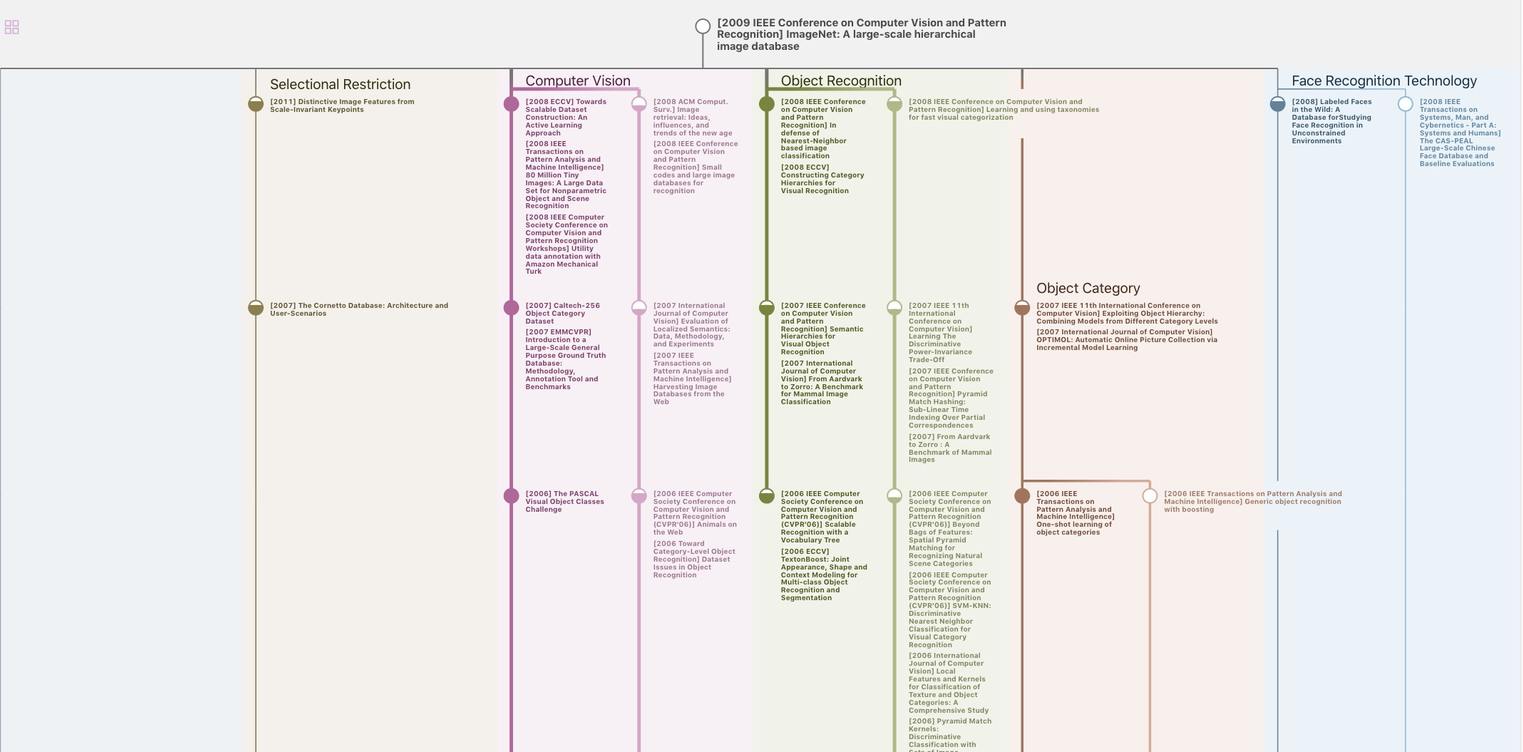
生成溯源树,研究论文发展脉络
Chat Paper
正在生成论文摘要