Invertible network for unpaired low-light image enhancement
The Visual Computer(2024)
摘要
Existing unpaired image enhancement approaches prefer to employ the traditional two-way generative adversarial network (GAN) framework, in which two convolutional neural network (CNN) generators are deployed for enhancement and degradation separately. However, such data-driven models ignore the inherent characteristics of transformation between the low-light and normal-light images, leading to unstable training and artifacts. Here, we propose to leverage the invertible neural network to enhance the low-light images in the forward process and degrade the unpaired normal-light photograph inversely. The generated and real images are then fed into discriminators for adversarial learning. In addition to the adversarial loss, we design transformation-consistent loss to ensure the stability of training, detail-preserving loss to preserve more image details, and reversibility loss to alleviate the over-exposure problem. Moreover, we present a progressive self-guided enhancement process in inference and achieve favorable performance against the state-of-the-art methods.
更多查看译文
关键词
Unpaired learning,Invertible network,Reversibility loss,Progressive self-guided enhancement
AI 理解论文
溯源树
样例
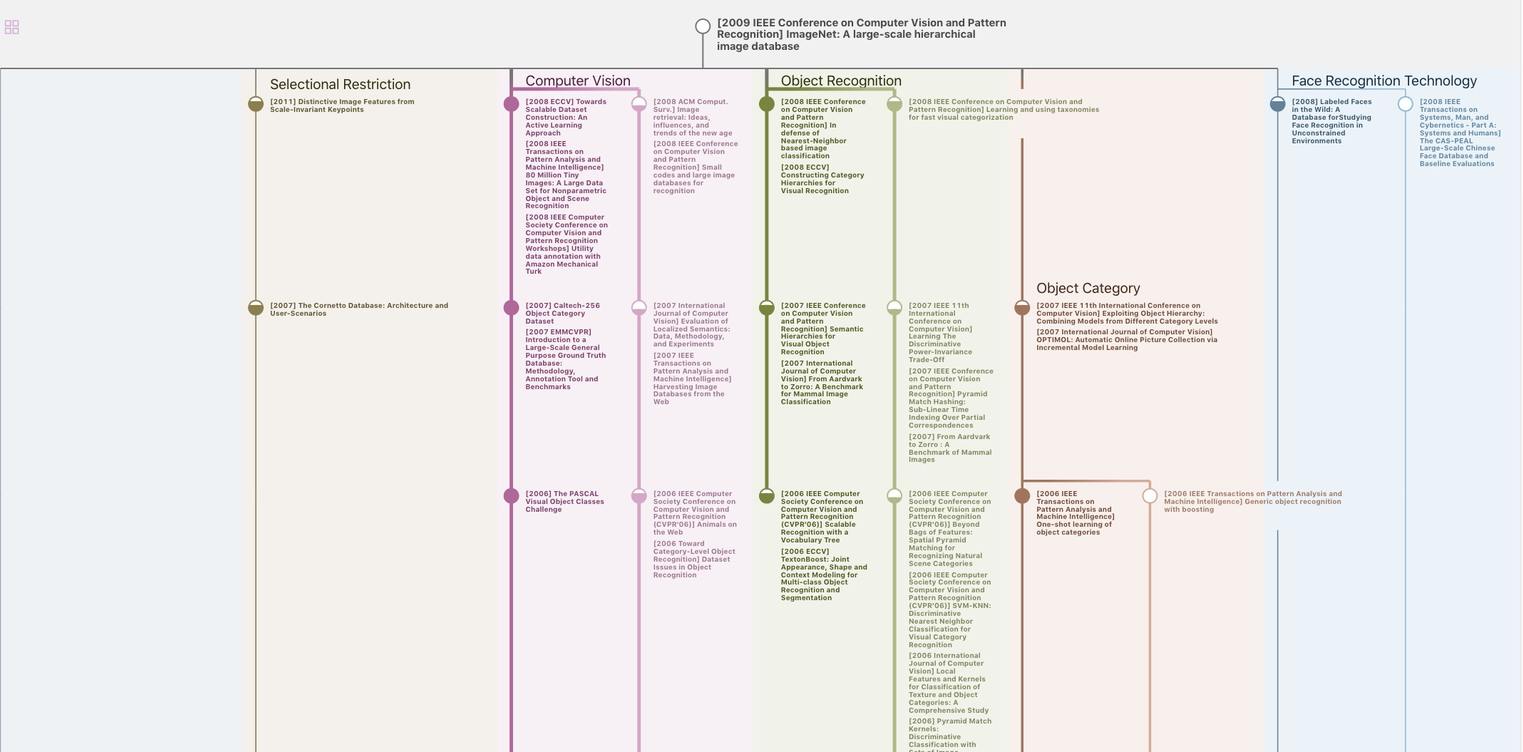
生成溯源树,研究论文发展脉络
Chat Paper
正在生成论文摘要