Annealed Leap-Point Sampler for Multimodal Target Distributions
arXiv (Cornell University)(2021)
摘要
In Bayesian statistics, exploring multimodal posterior distribution poses major challenges for existing techniques such as Markov Chain Monte Carlo (MCMC). These problems are exacerbated in high-dimensional settings where MCMC methods typically rely upon localised proposal mechanisms. This paper introduces the Annealed Leap-Point Sampler (ALPS), which augments the target distribution state space with modified annealed (cooled) target distributions, in contrast to traditional approaches which have employed tempering. The temperature of the coldest state is chosen such that its corresponding annealed target density can be sufficiently well-approximated by a Laplace approximation. As a result, a Gaussian mixture independence Metropolis-Hastings sampler can perform mode-jumping proposals even in high-dimensional problems. The ability of this procedure to "mode hop" at this super-cold state is then filtered through to the target state using a sequence of tempered targets in a similar way to that in parallel tempering methods. ALPS also incorporates the best aspects of current gold-standard approaches to multimodal sampling in high-dimensional contexts. A theoretical analysis of the ALPS approach in high dimensions is given, providing practitioners with a gauge on the optimal setup as well as the scalability of the algorithm. For a $d$-dimensional problem the it is shown that the coldest inverse temperature level required for the ALPS only needs to be linear in the dimension, $\mathcal{O}(d)$, and this means that for a collection of multimodal problems the algorithmic cost is polynomial, $\mathcal{O}\left(d^{3}\right)$. ALPS is illustrated on a complex multimodal posterior distribution that arises from a seemingly-unrelated regression (SUR) model of longitudinal data from U.S. manufacturing firms.
更多查看译文
关键词
multimodal target distributions,leap-point
AI 理解论文
溯源树
样例
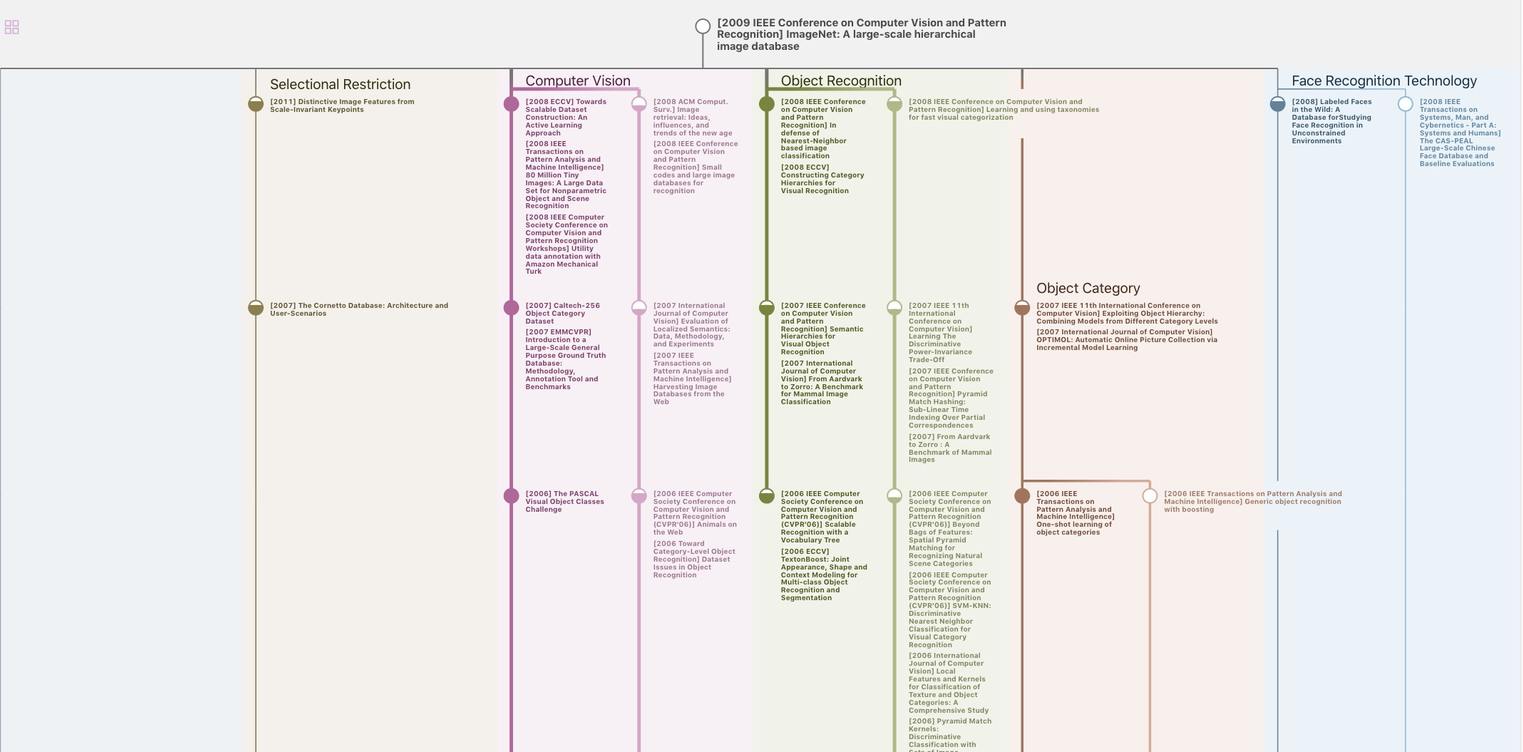
生成溯源树,研究论文发展脉络
Chat Paper
正在生成论文摘要