Adversarial Forces of Physical Models
semanticscholar(2020)
摘要
While most systems are governed by quantum mechanics at the nanoscale, it is almost always prohibitively expensive to simulate these systems by exactly solving Schrödinger’s equation. For this reason, a hierarchy of approximate models are commonly used in biology, chemistry, and materials science that allow practitioners to trade-off between accuracy and speed to simulate larger systems at longer time scales. Recently, significant attention has been devoted to leveraging machine learning to develop new and more accurate approximations. While these approximate models have typically been assessed based on their average-case performance, recent work in the adversarial example literature in other domains has offered ample evidence that this is often a poor indicator of worst-case performance. Here we show that there is a well defined sense of adversarial direction that governs the worst-case behavior for these approximate models of physical systems. Unlike in other contexts, where adversarial examples are scarce absent malicious intervention, in physical systems we show that the laws of physics can naturally lead the system to move in adversarial directions. Surprisingly, we find that these adversarial directions can exist even for traditional, analytic force fields such as the BKS potential. We verify our predictions by comparing a variety of hand-designed and machine learned models of quantum mechanical energies, including BehlerParrinello and graph neural networks trained on energies or forces, and ab initio quantum mechanical calculations. We conclude by discussing strategies that can prevent a physical model from moving in its adversarial directions, such as training on forces or adversarial forces.
更多查看译文
AI 理解论文
溯源树
样例
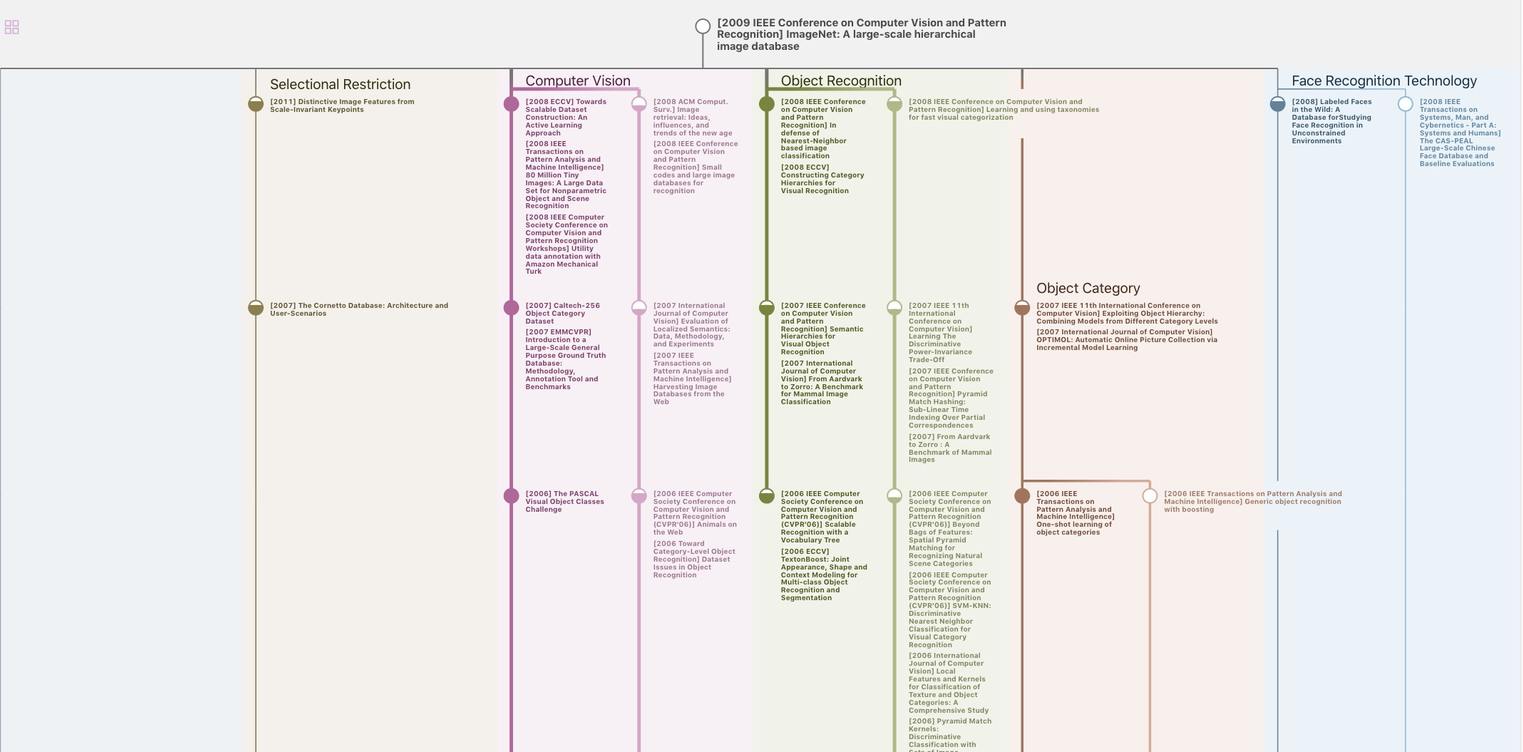
生成溯源树,研究论文发展脉络
Chat Paper
正在生成论文摘要