Glaucoma detection beyond the optic disc: The importance of the peripapillary region using explainable deep learning
ArXiv(2021)
摘要
Today, a large number of glaucoma cases remain undetected, resulting in irreversible blindness. In a quest for cost-effective screening, deep learning-based methods are being evaluated to detect glaucoma from color fundus images. Although unprecedented sensitivity and specificity values are reported, recent glaucoma detection deep learning models lack in decision transparency. Here, we propose a methodology that advances explainable deep learning in the field of glaucoma detection and vertical cup-disc ratio (VCDR), an important risk factor. We trained and evaluated a total of 64 deep learning models using fundus images that undergo a certain cropping policy. We defined the circular crop radius as a percentage of image size, centered on the optic nerve head (ONH), with an equidistant spaced range from 10%-60% (ONH crop policy). The inverse of the cropping mask was also applied to quantify the performance of models trained on ONH information exclusively (periphery crop policy). The performance of the models evaluated on original images resulted in an area under the curve (AUC) of 0.94 [95% CI: 0.92-0.96] for glaucoma detection, and a coefficient of determination (R2) equal to 77% [95% CI: 0.77-0.79] for VCDR estimation. Models that were trained on images with absence of the ONH are still able to obtain significant performance (0.88 [95% CI: 0.85-0.90] AUC for glaucoma detection and 37% [95% CI: 0.35-0.40] R2 score for VCDR estimation in the most extreme setup of 60% ONH crop). We validated our glaucoma detection models on a recent public data set (REFUGE) that contains images captured with a different camera, still achieving an AUC of 0.80 [95% CI: 0.76-0.84] when ONH crop policy of 60% image size was applied. Our findings provide the first irrefutable evidence that deep learning can detect glaucoma from fundus image regions outside the ONH. This can be useful in settings where ONH assessment is challenging. Glaucoma is a leading cause of irreversible blindness in our ageing society with a projected number of patients of 112 million by 2040.1 This chronic neuropathy induces structural optic nerve fiber damage with visible changes in and outside the optic disc, ultimately leading to functional vision loss. Glaucoma is associated with characteristic changes of the optic nerve head (ONH), also called the optic disc.2 During clinical examination and optic disc photo analysis, ophthalmologists evaluate the ONH, looking for typical changes such as generalized or focal neural rim thinning. Neuroretinal rim thinning can be quantified in fundus photos by measuring the vertical cup-to-disc ratio (VCDR).3 The optic cup is the distinguishable excavation in the central portion of the ONH. It is typically small in normal eyes but increases with neuroretinal rim loss.4 An elevated VCDR or interocular asymmetry > 0.2 is therefore considered suspicious for glaucoma (Figures 1-2).5 Although clinicians tend to focus mainly on the optic disc for diagnosing glaucoma, retinal nerve fiber layer (RNFL) defects (adjacent to the ONH) are also known as a typical indicator of glaucomatous damage.6 However, for the evaluation of RNFL defects, typically papillo-macular area centered red-free fundus images are used for optimal visualization of the RNFL. Even then, clinical detection of RNFL defects by red-free fundus photography is only possible after a 50% loss of the RNFL.7 Deep learning models and especially convolutional neural networks (CNN) are setting new benchmarks in medical image analysis. These models are finding their way in a plethora of healthcare applications including dermatologist-level classification of skin cancer8 and identification of pneumonia on chest CT9. In ophthalmology, the main research focus has been the diagnostic ability of CNNs in the ‘big four’ eye diseases (diabetic retinopathy10, glaucoma11, age-related macular degeneration12 and cataract13) using widely available color fundus photos and to a lesser extent optical coherence tomography (OCT) scans. Diagnostic models using deep learning can play a role in overcoming the challenge of glaucoma underdiagnosis while maintaining a limited false positive rate.14 Successes have already been booked in the field of automated glaucoma diagnosis11,15–31 and glaucoma-related parameters32,33 from fundus images using CNNs. The use of end-to-end deep learning in glaucoma led to a high reference sensitivity of 97.60% at 85% specificity in a recent international challenge.34 Unfortunately, those results came at the cost of lower insights into the decision process of the predictive model, as image features are no longer manually crafted and selected. Decision-making transparency, also referred to as explainability of the CNN, is crucial to build trust for future use of deep learning in medical diagnosis. Furthermore, it is currently unknown to what extent information outside the ONH (peripapillary area) in color fundus images is relevant to glaucoma diagnosis for deep learning. Trained deep learning models for glaucoma detection could leverage subtle changes such as RNFL thinning that human experts cannot detect. Several studies attempted to explain the deep learning model’s decision in glaucoma classification from fundus images.20,23–26,28,31,35 The majority of explainability studies20,24,28 employed some form of occlusion36, a technique in which parts of the test images are perturbed, and the effect on performance recorded. They mainly report significant importance of areas within the ONH. Some mentioned the presence of relevant regions directly outside the ONH in a small number of images. One major downside of occlusion testing is the violation of having a similar distribution in train and test sets. When training on a complete image, and evaluating on a perturbed image, it is impossible to assess whether the change in prediction is due to the perturbation or because the omitted information was truly (un)informative.37 A solution is to occlude the same part of the images used for training, a principle which was recently named Remove And Retrain (ROAR).38 Using two pseudo-anonymized data sets of disc-centered fundus images from the University Hospitals Leuven (UZL), a large glaucoma clinic in Belgium, the goal of this work was to analyze the importance of the regions beyond the ONH and provide objective explainability in the context of glaucoma detection and VCDR estimation. To achieve this, we trained and evaluated several CNNs with a varying amount of image covered and compared performance between cover size and application (glaucoma classification/VCDR regression). We validated our glaucoma detection models on REFUGE, a public data set of 1200 glaucoma-labeled color fundus images. Our findings provide hard evidence that deep learning utilizes information outside the ONH during glaucoma detection and VCDR estimation.
更多查看译文
关键词
glaucoma detection,explainable deep learning,peripapillary region,optic disc,deep learning
AI 理解论文
溯源树
样例
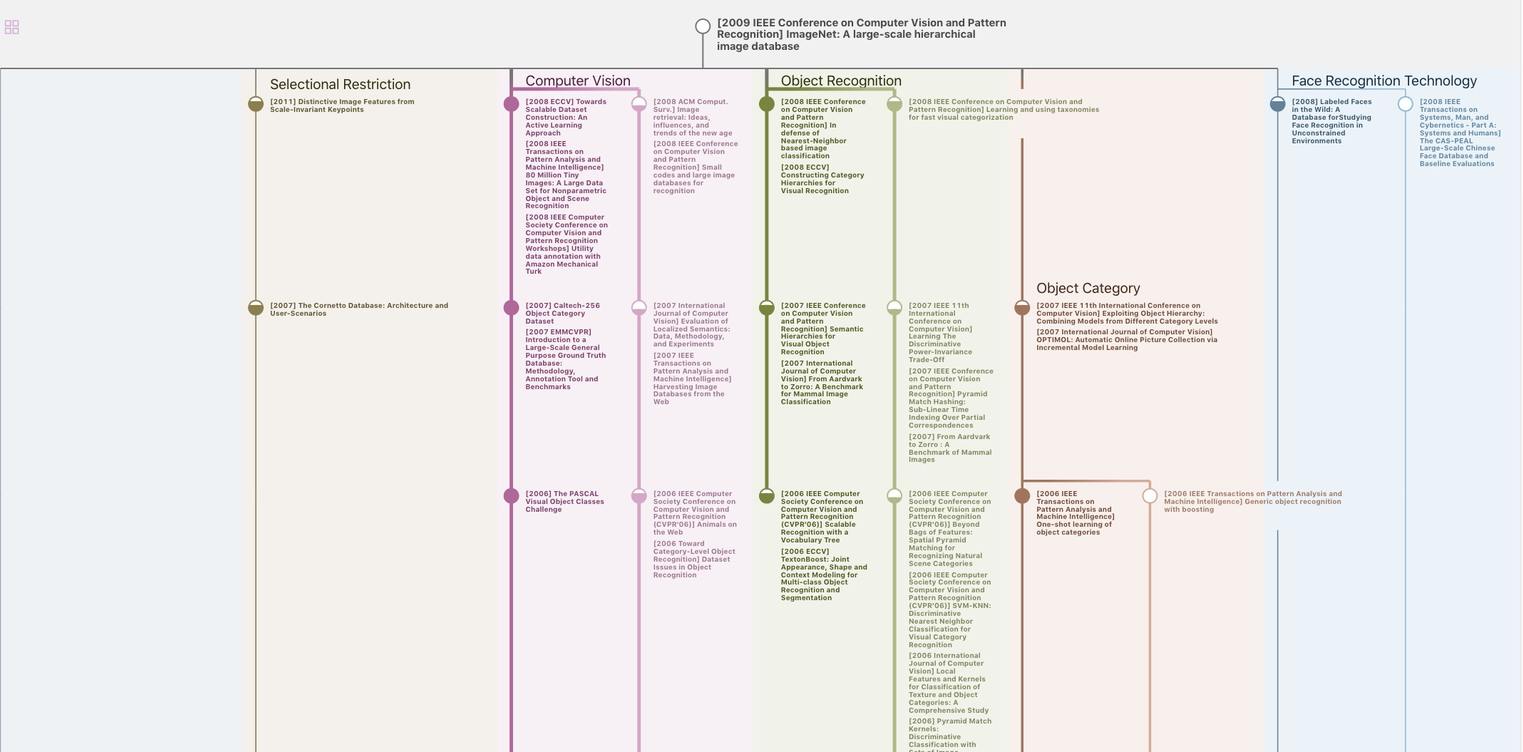
生成溯源树,研究论文发展脉络
Chat Paper
正在生成论文摘要