The Effect of Image Resolution on Automated Classification of Chest X-rays
medRxiv(2021)
摘要
Deep learning models have received much attention lately for their ability to achieve expert-level performance on the accurate automated analysis of chest X-rays. Although publicly available chest X-ray datasets include high resolution images, most models are trained on reduced size images due to limitations on GPU memory and training time. As compute capability continues to advance, it will become feasible to train large convolutional neural networks on high-resolution images. This study is based on the publicly available MIMIC-CXR-JPG dataset, comprising 377,110 high resolution chest X-ray images, and provided with 14 labels to the corresponding free-text radiology reports. We find, interestingly, that tasks that require a large receptive field are better suited to downscaled input images, and we verify this qualitatively by inspecting effective receptive fields and class activation maps of trained models. Finally, we show that stacking an ensemble across resolutions outperforms each individual learner at all input resolutions while providing interpretable scale weights, suggesting that multi-scale features are crucially important to information extraction from high-resolution chest X-rays.
更多查看译文
关键词
image resolution,classification,x-rays
AI 理解论文
溯源树
样例
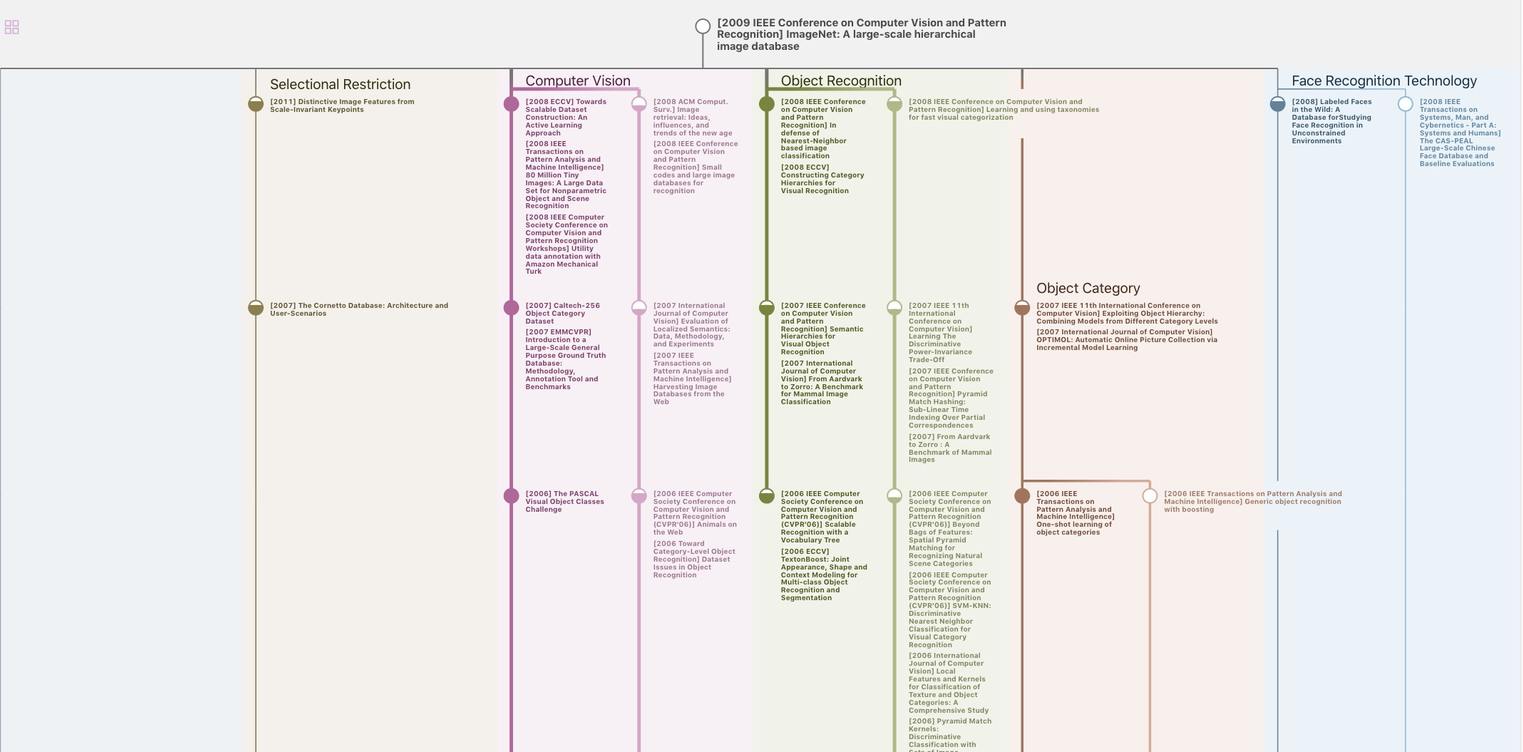
生成溯源树,研究论文发展脉络
Chat Paper
正在生成论文摘要