Range-Doppler Spectrograms-Based Clutter Suppression of HF Passive Bistatic Radar by D-CycleGAN
IEEE SENSORS JOURNAL(2021)
摘要
As a new attempt to reject clutter in High Frequency Passive Bistatic Radar (HFPBR), the Cycle-Consistent Adversarial Network (CycleGAN)-based image domain clutter suppression algorithm works successfully by treating the clutter rejection problem as an image-to-image migration between the range-doppler spectrograms without and with clutter suppression. However, this algorithm also has some disadvantages such as too many calculation parameters, difficult model training and low clutter suppression rate. In this paper, a novel clutter suppression method based on an improved DenseNet CycleGAN (D-CycleGAN) is proposed. In this method, the ResNet block of the generator in the original CycleGAN network is replaced with the DenseNet block, to better extract clutter features. To better evaluate the clutter suppression performance of the deep learning network-based graph-relational method, a new index suitable for HF passive radar is introduced. Furthermore, the influence of the number of Dense blocks on the performance of the algorithm is also discussed. Finally, the performance of the proposed method is tested and verified on the measured data, compared with the traditional clutter suppression method and other popular image migration algorithms, such as Pix2pix. The results show that the D-CycleGAN-based method has better clutter suppression performance, stronger generalization ability, and better retained radar targets simultaneously.
更多查看译文
关键词
Clutter,Spectrogram,Feature extraction,Generators,Deep learning,Sensors,Radar,Passive bistatic radar,clutter suppression,range-doppler spectrogram,CycleGAN,denseNet
AI 理解论文
溯源树
样例
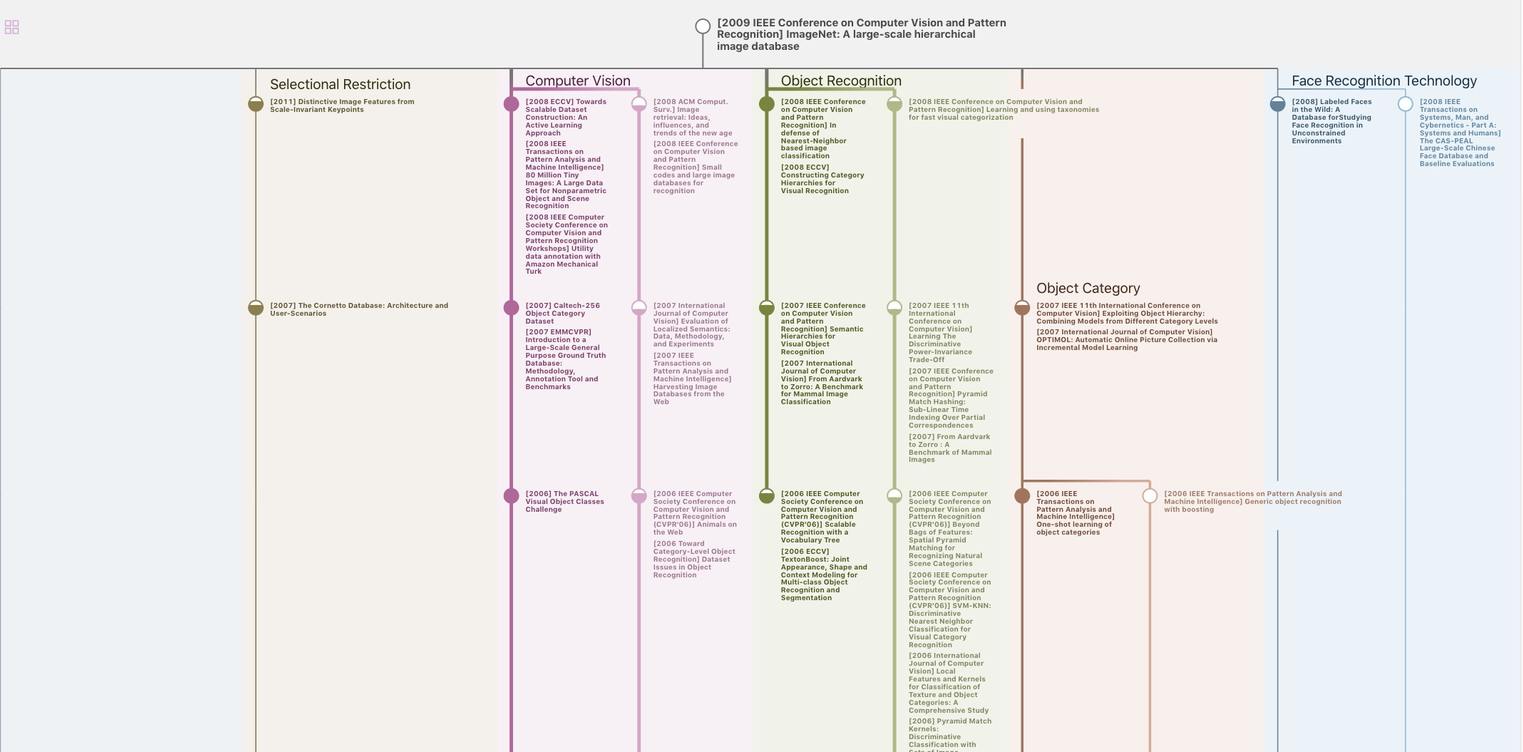
生成溯源树,研究论文发展脉络
Chat Paper
正在生成论文摘要