Hardware Aware Sparsity Generation for Convolutional Neural Networks
semanticscholar(2020)
摘要
Weight sparsity has gained a lot of attention as a compression method in recent years with the increasing demand of hardware containing sparse accelerators. Among all the methods of weight sparsity, AutoML leads to the best performance in terms of compression ratio and accuracy. However, the compression ratio cannot guarantee such network to perform the best on target hardware. Therefore, in this work we introduce hardware aware method of weight sparsity targeting optimal performance on the given hardware. Using our method, ResNets and MobileNetv2 can be reduced to 45% and 70% of original cycles respectively with almost no Top 1 accuracy loss which the other weight sparsity methods cannot achieve. Our work also bring insights of sparse weight distribution on hardware which contains sparse accelerators.
更多查看译文
AI 理解论文
溯源树
样例
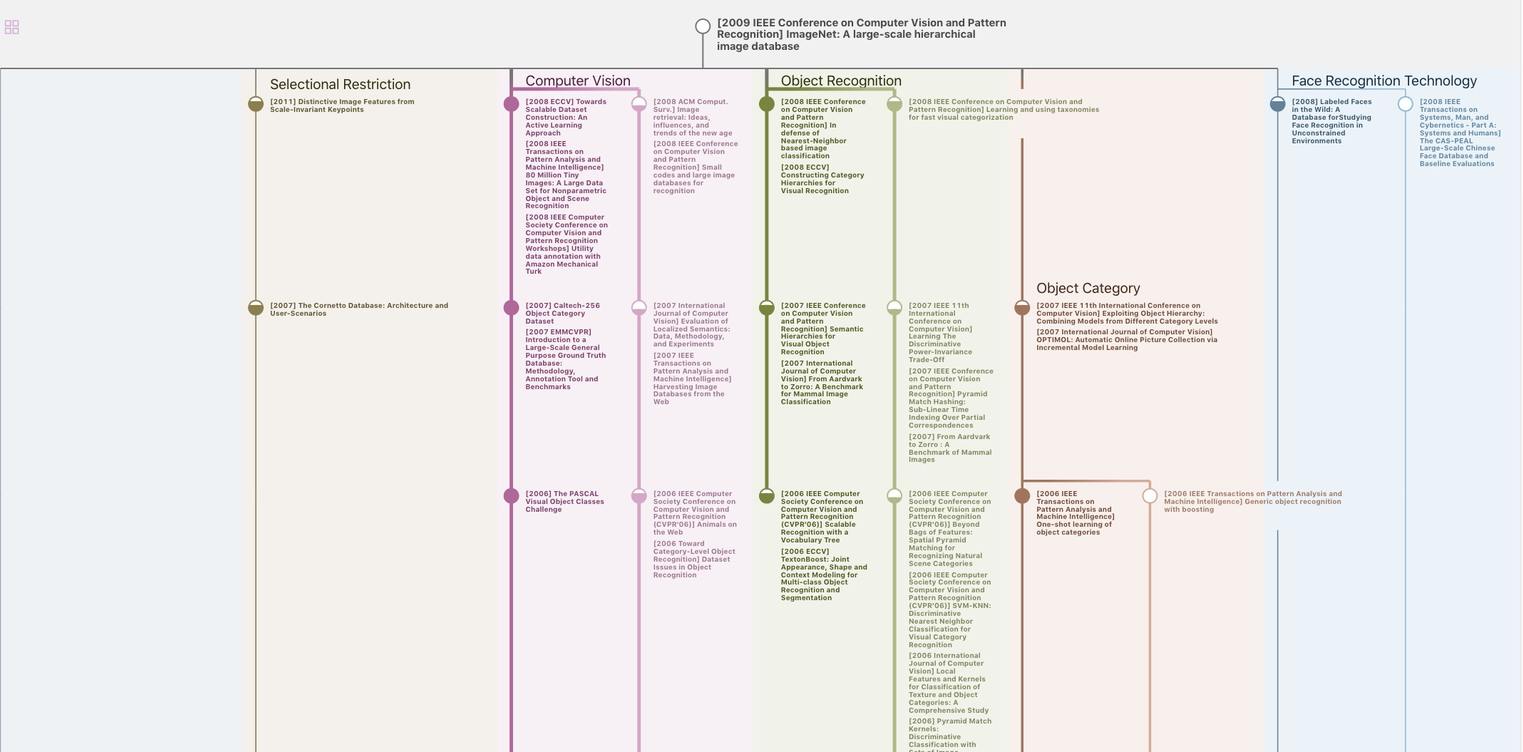
生成溯源树,研究论文发展脉络
Chat Paper
正在生成论文摘要